This report benchmark the performance of colWeightedMedians() and rowWeightedMedians() against alternative methods.
Alternative methods
- apply() + weightedMedian()
Data
> rmatrix <- function(nrow, ncol, mode = c("logical", "double", "integer", "index"), range = c(-100,
+ +100), na_prob = 0) {
+ mode <- match.arg(mode)
+ n <- nrow * ncol
+ if (mode == "logical") {
+ x <- sample(c(FALSE, TRUE), size = n, replace = TRUE)
+ } else if (mode == "index") {
+ x <- seq_len(n)
+ mode <- "integer"
+ } else {
+ x <- runif(n, min = range[1], max = range[2])
+ }
+ storage.mode(x) <- mode
+ if (na_prob > 0)
+ x[sample(n, size = na_prob * n)] <- NA
+ dim(x) <- c(nrow, ncol)
+ x
+ }
> rmatrices <- function(scale = 10, seed = 1, ...) {
+ set.seed(seed)
+ data <- list()
+ data[[1]] <- rmatrix(nrow = scale * 1, ncol = scale * 1, ...)
+ data[[2]] <- rmatrix(nrow = scale * 10, ncol = scale * 10, ...)
+ data[[3]] <- rmatrix(nrow = scale * 100, ncol = scale * 1, ...)
+ data[[4]] <- t(data[[3]])
+ data[[5]] <- rmatrix(nrow = scale * 10, ncol = scale * 100, ...)
+ data[[6]] <- t(data[[5]])
+ names(data) <- sapply(data, FUN = function(x) paste(dim(x), collapse = "x"))
+ data
+ }
> data <- rmatrices(mode = "double")
Results
10x10 matrix
> X <- data[["10x10"]]
> w <- runif(nrow(X))
> gc()
used (Mb) gc trigger (Mb) max used (Mb)
Ncells 5336126 285.0 8529671 455.6 8529671 455.6
Vcells 10891982 83.1 31876688 243.2 60562128 462.1
> colStats <- microbenchmark(colWeightedMedians = colWeightedMedians(X, w = w, na.rm = FALSE), `apply+weigthedMedian` = apply(X,
+ MARGIN = 2L, FUN = weightedMedian, w = w, na.rm = FALSE), unit = "ms")
> X <- t(X)
> gc()
used (Mb) gc trigger (Mb) max used (Mb)
Ncells 5325293 284.5 8529671 455.6 8529671 455.6
Vcells 10856360 82.9 31876688 243.2 60562128 462.1
> rowStats <- microbenchmark(rowWeightedMedians = rowWeightedMedians(X, w = w, na.rm = FALSE), `apply+weigthedMedian` = apply(X,
+ MARGIN = 1L, FUN = weightedMedian, w = w, na.rm = FALSE), unit = "ms")
Table: Benchmarking of colWeightedMedians() and apply+weigthedMedian() on 10x10 data. The top panel shows times in milliseconds and the bottom panel shows relative times.
|
expr |
min |
lq |
mean |
median |
uq |
max |
2 |
apply+weigthedMedian |
0.057735 |
0.0607080 |
0.0643024 |
0.0626710 |
0.0653680 |
0.152657 |
1 |
colWeightedMedians |
0.069744 |
0.0727645 |
0.0767094 |
0.0751535 |
0.0786175 |
0.112733 |
|
expr |
min |
lq |
mean |
median |
uq |
max |
2 |
apply+weigthedMedian |
1.000000 |
1.000000 |
1.000000 |
1.000000 |
1.000000 |
1.0000000 |
1 |
colWeightedMedians |
1.208002 |
1.198598 |
1.192948 |
1.199175 |
1.202691 |
0.7384725 |
Table: Benchmarking of rowWeightedMedians() and apply+weigthedMedian() on 10x10 data (transposed). The top panel shows times in milliseconds and the bottom panel shows relative times.
|
expr |
min |
lq |
mean |
median |
uq |
max |
2 |
apply+weigthedMedian |
0.054782 |
0.0593475 |
0.0631794 |
0.0613125 |
0.0648945 |
0.145517 |
1 |
rowWeightedMedians |
0.067214 |
0.0728870 |
0.0767203 |
0.0758585 |
0.0801185 |
0.112614 |
|
expr |
min |
lq |
mean |
median |
uq |
max |
2 |
apply+weigthedMedian |
1.000000 |
1.000000 |
1.000000 |
1.000000 |
1.000000 |
1.000000 |
1 |
rowWeightedMedians |
1.226936 |
1.228139 |
1.214324 |
1.237244 |
1.234596 |
0.773889 |
Figure: Benchmarking of colWeightedMedians() and apply+weigthedMedian() on 10x10 data as well as rowWeightedMedians() and apply+weigthedMedian() on the same data transposed. Outliers are displayed as crosses. Times are in milliseconds.
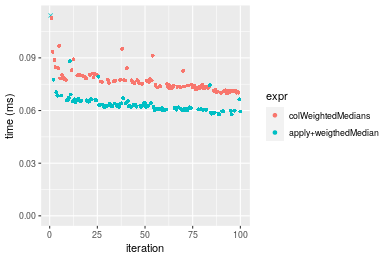
Table: Benchmarking of colWeightedMedians() and rowWeightedMedians() on 10x10 data (original and transposed). The top panel shows times in milliseconds and the bottom panel shows relative times.
|
expr |
min |
lq |
mean |
median |
uq |
max |
1 |
colWeightedMedians |
69.744 |
72.7645 |
76.70944 |
75.1535 |
78.6175 |
112.733 |
2 |
rowWeightedMedians |
67.214 |
72.8870 |
76.72029 |
75.8585 |
80.1185 |
112.614 |
|
expr |
min |
lq |
mean |
median |
uq |
max |
1 |
colWeightedMedians |
1.0000000 |
1.000000 |
1.000000 |
1.000000 |
1.000000 |
1.0000000 |
2 |
rowWeightedMedians |
0.9637245 |
1.001683 |
1.000141 |
1.009381 |
1.019092 |
0.9989444 |
Figure: Benchmarking of colWeightedMedians() and rowWeightedMedians() on 10x10 data (original and transposed). Outliers are displayed as crosses. Times are in milliseconds.
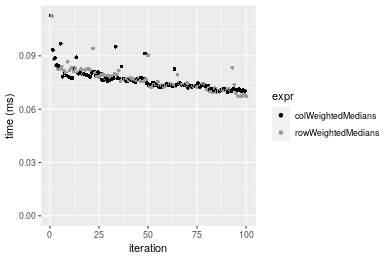
100x100 matrix
> X <- data[["100x100"]]
> w <- runif(nrow(X))
> gc()
used (Mb) gc trigger (Mb) max used (Mb)
Ncells 5323872 284.4 8529671 455.6 8529671 455.6
Vcells 10471222 79.9 31876688 243.2 60562128 462.1
> colStats <- microbenchmark(colWeightedMedians = colWeightedMedians(X, w = w, na.rm = FALSE), `apply+weigthedMedian` = apply(X,
+ MARGIN = 2L, FUN = weightedMedian, w = w, na.rm = FALSE), unit = "ms")
> X <- t(X)
> gc()
used (Mb) gc trigger (Mb) max used (Mb)
Ncells 5323848 284.4 8529671 455.6 8529671 455.6
Vcells 10481235 80.0 31876688 243.2 60562128 462.1
> rowStats <- microbenchmark(rowWeightedMedians = rowWeightedMedians(X, w = w, na.rm = FALSE), `apply+weigthedMedian` = apply(X,
+ MARGIN = 1L, FUN = weightedMedian, w = w, na.rm = FALSE), unit = "ms")
Table: Benchmarking of colWeightedMedians() and apply+weigthedMedian() on 100x100 data. The top panel shows times in milliseconds and the bottom panel shows relative times.
|
expr |
min |
lq |
mean |
median |
uq |
max |
2 |
apply+weigthedMedian |
0.698149 |
0.7197470 |
0.7778816 |
0.755399 |
0.7825195 |
1.254192 |
1 |
colWeightedMedians |
0.755686 |
0.7709115 |
0.8548933 |
0.818420 |
0.8587720 |
1.262195 |
|
expr |
min |
lq |
mean |
median |
uq |
max |
2 |
apply+weigthedMedian |
1.000000 |
1.000000 |
1.000000 |
1.000000 |
1.000000 |
1.000000 |
1 |
colWeightedMedians |
1.082414 |
1.071087 |
1.099002 |
1.083427 |
1.097445 |
1.006381 |
Table: Benchmarking of rowWeightedMedians() and apply+weigthedMedian() on 100x100 data (transposed). The top panel shows times in milliseconds and the bottom panel shows relative times.
|
expr |
min |
lq |
mean |
median |
uq |
max |
2 |
apply+weigthedMedian |
0.699066 |
0.712970 |
0.7666201 |
0.7449215 |
0.7633770 |
1.247787 |
1 |
rowWeightedMedians |
0.762679 |
0.785641 |
0.8554737 |
0.8185300 |
0.8626815 |
1.267212 |
|
expr |
min |
lq |
mean |
median |
uq |
max |
2 |
apply+weigthedMedian |
1.000000 |
1.000000 |
1.000000 |
1.000000 |
1.000000 |
1.000000 |
1 |
rowWeightedMedians |
1.090997 |
1.101927 |
1.115903 |
1.098814 |
1.130086 |
1.015568 |
Figure: Benchmarking of colWeightedMedians() and apply+weigthedMedian() on 100x100 data as well as rowWeightedMedians() and apply+weigthedMedian() on the same data transposed. Outliers are displayed as crosses. Times are in milliseconds.
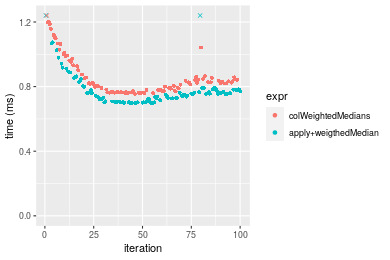
Table: Benchmarking of colWeightedMedians() and rowWeightedMedians() on 100x100 data (original and transposed). The top panel shows times in milliseconds and the bottom panel shows relative times.
|
expr |
min |
lq |
mean |
median |
uq |
max |
1 |
colWeightedMedians |
755.686 |
770.9115 |
854.8933 |
818.42 |
858.7720 |
1262.195 |
2 |
rowWeightedMedians |
762.679 |
785.6410 |
855.4737 |
818.53 |
862.6815 |
1267.212 |
|
expr |
min |
lq |
mean |
median |
uq |
max |
1 |
colWeightedMedians |
1.000000 |
1.000000 |
1.000000 |
1.000000 |
1.000000 |
1.000000 |
2 |
rowWeightedMedians |
1.009254 |
1.019107 |
1.000679 |
1.000134 |
1.004552 |
1.003975 |
Figure: Benchmarking of colWeightedMedians() and rowWeightedMedians() on 100x100 data (original and transposed). Outliers are displayed as crosses. Times are in milliseconds.
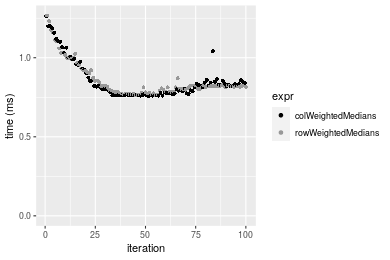
1000x10 matrix
> X <- data[["1000x10"]]
> w <- runif(nrow(X))
> gc()
used (Mb) gc trigger (Mb) max used (Mb)
Ncells 5324573 284.4 8529671 455.6 8529671 455.6
Vcells 10475586 80.0 31876688 243.2 60562128 462.1
> colStats <- microbenchmark(colWeightedMedians = colWeightedMedians(X, w = w, na.rm = FALSE), `apply+weigthedMedian` = apply(X,
+ MARGIN = 2L, FUN = weightedMedian, w = w, na.rm = FALSE), unit = "ms")
> X <- t(X)
> gc()
used (Mb) gc trigger (Mb) max used (Mb)
Ncells 5324567 284.4 8529671 455.6 8529671 455.6
Vcells 10485629 80.0 31876688 243.2 60562128 462.1
> rowStats <- microbenchmark(rowWeightedMedians = rowWeightedMedians(X, w = w, na.rm = FALSE), `apply+weigthedMedian` = apply(X,
+ MARGIN = 1L, FUN = weightedMedian, w = w, na.rm = FALSE), unit = "ms")
Table: Benchmarking of colWeightedMedians() and apply+weigthedMedian() on 1000x10 data. The top panel shows times in milliseconds and the bottom panel shows relative times.
|
expr |
min |
lq |
mean |
median |
uq |
max |
2 |
apply+weigthedMedian |
0.566671 |
0.5727345 |
0.6213638 |
0.5881475 |
0.6245870 |
0.941552 |
1 |
colWeightedMedians |
0.585212 |
0.5931230 |
0.6585967 |
0.6203480 |
0.6876295 |
1.078221 |
|
expr |
min |
lq |
mean |
median |
uq |
max |
2 |
apply+weigthedMedian |
1.000000 |
1.000000 |
1.000000 |
1.000000 |
1.000000 |
1.000000 |
1 |
colWeightedMedians |
1.032719 |
1.035599 |
1.059921 |
1.054749 |
1.100935 |
1.145153 |
Table: Benchmarking of rowWeightedMedians() and apply+weigthedMedian() on 1000x10 data (transposed). The top panel shows times in milliseconds and the bottom panel shows relative times.
|
expr |
min |
lq |
mean |
median |
uq |
max |
2 |
apply+weigthedMedian |
0.567541 |
0.572906 |
0.6191645 |
0.585090 |
0.6164795 |
0.860573 |
1 |
rowWeightedMedians |
0.588173 |
0.594631 |
0.6423677 |
0.610705 |
0.6421510 |
0.978510 |
|
expr |
min |
lq |
mean |
median |
uq |
max |
2 |
apply+weigthedMedian |
1.000000 |
1.000000 |
1.000000 |
1.00000 |
1.000000 |
1.000000 |
1 |
rowWeightedMedians |
1.036353 |
1.037921 |
1.037475 |
1.04378 |
1.041642 |
1.137045 |
Figure: Benchmarking of colWeightedMedians() and apply+weigthedMedian() on 1000x10 data as well as rowWeightedMedians() and apply+weigthedMedian() on the same data transposed. Outliers are displayed as crosses. Times are in milliseconds.
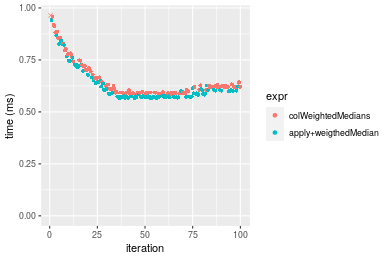
Table: Benchmarking of colWeightedMedians() and rowWeightedMedians() on 1000x10 data (original and transposed). The top panel shows times in milliseconds and the bottom panel shows relative times.
|
expr |
min |
lq |
mean |
median |
uq |
max |
2 |
rowWeightedMedians |
588.173 |
594.631 |
642.3677 |
610.705 |
642.1510 |
978.510 |
1 |
colWeightedMedians |
585.212 |
593.123 |
658.5968 |
620.348 |
687.6295 |
1078.221 |
|
expr |
min |
lq |
mean |
median |
uq |
max |
2 |
rowWeightedMedians |
1.0000000 |
1.000000 |
1.000000 |
1.00000 |
1.000000 |
1.000000 |
1 |
colWeightedMedians |
0.9949658 |
0.997464 |
1.025265 |
1.01579 |
1.070822 |
1.101901 |
Figure: Benchmarking of colWeightedMedians() and rowWeightedMedians() on 1000x10 data (original and transposed). Outliers are displayed as crosses. Times are in milliseconds.
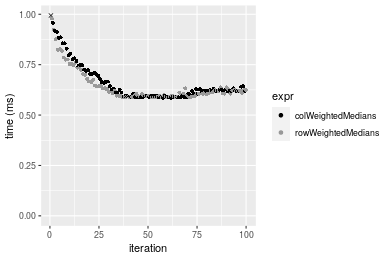
10x1000 matrix
> X <- data[["10x1000"]]
> w <- runif(nrow(X))
> gc()
used (Mb) gc trigger (Mb) max used (Mb)
Ncells 5324789 284.4 8529671 455.6 8529671 455.6
Vcells 10475454 80.0 31876688 243.2 60562128 462.1
> colStats <- microbenchmark(colWeightedMedians = colWeightedMedians(X, w = w, na.rm = FALSE), `apply+weigthedMedian` = apply(X,
+ MARGIN = 2L, FUN = weightedMedian, w = w, na.rm = FALSE), unit = "ms")
> X <- t(X)
> gc()
used (Mb) gc trigger (Mb) max used (Mb)
Ncells 5324765 284.4 8529671 455.6 8529671 455.6
Vcells 10485467 80.0 31876688 243.2 60562128 462.1
> rowStats <- microbenchmark(rowWeightedMedians = rowWeightedMedians(X, w = w, na.rm = FALSE), `apply+weigthedMedian` = apply(X,
+ MARGIN = 1L, FUN = weightedMedian, w = w, na.rm = FALSE), unit = "ms")
Table: Benchmarking of colWeightedMedians() and apply+weigthedMedian() on 10x1000 data. The top panel shows times in milliseconds and the bottom panel shows relative times.
|
expr |
min |
lq |
mean |
median |
uq |
max |
2 |
apply+weigthedMedian |
2.753192 |
3.078094 |
3.213874 |
3.144999 |
3.216814 |
9.785959 |
1 |
colWeightedMedians |
3.321706 |
3.697391 |
3.871337 |
3.772746 |
3.853225 |
10.209476 |
|
expr |
min |
lq |
mean |
median |
uq |
max |
2 |
apply+weigthedMedian |
1.000000 |
1.000000 |
1.00000 |
1.000000 |
1.000000 |
1.000000 |
1 |
colWeightedMedians |
1.206493 |
1.201195 |
1.20457 |
1.199601 |
1.197839 |
1.043278 |
Table: Benchmarking of rowWeightedMedians() and apply+weigthedMedian() on 10x1000 data (transposed). The top panel shows times in milliseconds and the bottom panel shows relative times.
|
expr |
min |
lq |
mean |
median |
uq |
max |
2 |
apply+weigthedMedian |
2.783791 |
3.10578 |
3.236681 |
3.157621 |
3.213204 |
9.654826 |
1 |
rowWeightedMedians |
3.281124 |
3.66063 |
3.842434 |
3.759111 |
3.838177 |
10.139083 |
|
expr |
min |
lq |
mean |
median |
uq |
max |
2 |
apply+weigthedMedian |
1.000000 |
1.000000 |
1.000000 |
1.000000 |
1.000000 |
1.000000 |
1 |
rowWeightedMedians |
1.178653 |
1.178651 |
1.187153 |
1.190488 |
1.194502 |
1.050157 |
Figure: Benchmarking of colWeightedMedians() and apply+weigthedMedian() on 10x1000 data as well as rowWeightedMedians() and apply+weigthedMedian() on the same data transposed. Outliers are displayed as crosses. Times are in milliseconds.
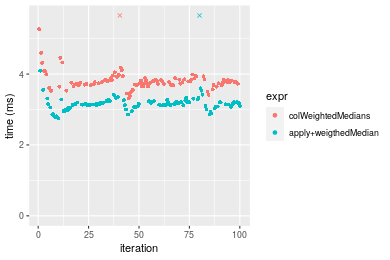
Table: Benchmarking of colWeightedMedians() and rowWeightedMedians() on 10x1000 data (original and transposed). The top panel shows times in milliseconds and the bottom panel shows relative times.
|
expr |
min |
lq |
mean |
median |
uq |
max |
2 |
rowWeightedMedians |
3.281124 |
3.660630 |
3.842434 |
3.759111 |
3.838177 |
10.13908 |
1 |
colWeightedMedians |
3.321706 |
3.697391 |
3.871337 |
3.772746 |
3.853225 |
10.20948 |
|
expr |
min |
lq |
mean |
median |
uq |
max |
2 |
rowWeightedMedians |
1.000000 |
1.000000 |
1.000000 |
1.000000 |
1.00000 |
1.000000 |
1 |
colWeightedMedians |
1.012368 |
1.010042 |
1.007522 |
1.003627 |
1.00392 |
1.006943 |
Figure: Benchmarking of colWeightedMedians() and rowWeightedMedians() on 10x1000 data (original and transposed). Outliers are displayed as crosses. Times are in milliseconds.
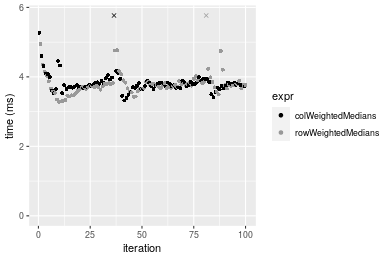
100x1000 matrix
> X <- data[["100x1000"]]
> w <- runif(nrow(X))
> gc()
used (Mb) gc trigger (Mb) max used (Mb)
Ncells 5324967 284.4 8529671 455.6 8529671 455.6
Vcells 10476036 80.0 31876688 243.2 60562128 462.1
> colStats <- microbenchmark(colWeightedMedians = colWeightedMedians(X, w = w, na.rm = FALSE), `apply+weigthedMedian` = apply(X,
+ MARGIN = 2L, FUN = weightedMedian, w = w, na.rm = FALSE), unit = "ms")
> X <- t(X)
> gc()
used (Mb) gc trigger (Mb) max used (Mb)
Ncells 5324955 284.4 8529671 455.6 8529671 455.6
Vcells 10576069 80.7 31876688 243.2 60562128 462.1
> rowStats <- microbenchmark(rowWeightedMedians = rowWeightedMedians(X, w = w, na.rm = FALSE), `apply+weigthedMedian` = apply(X,
+ MARGIN = 1L, FUN = weightedMedian, w = w, na.rm = FALSE), unit = "ms")
Table: Benchmarking of colWeightedMedians() and apply+weigthedMedian() on 100x1000 data. The top panel shows times in milliseconds and the bottom panel shows relative times.
|
expr |
min |
lq |
mean |
median |
uq |
max |
2 |
apply+weigthedMedian |
6.890591 |
7.480619 |
7.825088 |
7.554851 |
7.633451 |
27.24606 |
1 |
colWeightedMedians |
7.395820 |
8.068511 |
8.369593 |
8.148709 |
8.241084 |
26.70743 |
|
expr |
min |
lq |
mean |
median |
uq |
max |
2 |
apply+weigthedMedian |
1.000000 |
1.000000 |
1.000000 |
1.000000 |
1.000000 |
1.000000 |
1 |
colWeightedMedians |
1.073322 |
1.078589 |
1.069584 |
1.078606 |
1.079601 |
0.980231 |
Table: Benchmarking of rowWeightedMedians() and apply+weigthedMedian() on 100x1000 data (transposed). The top panel shows times in milliseconds and the bottom panel shows relative times.
|
expr |
min |
lq |
mean |
median |
uq |
max |
2 |
apply+weigthedMedian |
6.926695 |
7.492705 |
7.639871 |
7.559138 |
7.635518 |
9.637283 |
1 |
rowWeightedMedians |
7.464479 |
8.106582 |
8.607993 |
8.156696 |
8.231931 |
27.903324 |
|
expr |
min |
lq |
mean |
median |
uq |
max |
2 |
apply+weigthedMedian |
1.000000 |
1.00000 |
1.00000 |
1.000000 |
1.00000 |
1.000000 |
1 |
rowWeightedMedians |
1.077639 |
1.08193 |
1.12672 |
1.079051 |
1.07811 |
2.895352 |
Figure: Benchmarking of colWeightedMedians() and apply+weigthedMedian() on 100x1000 data as well as rowWeightedMedians() and apply+weigthedMedian() on the same data transposed. Outliers are displayed as crosses. Times are in milliseconds.
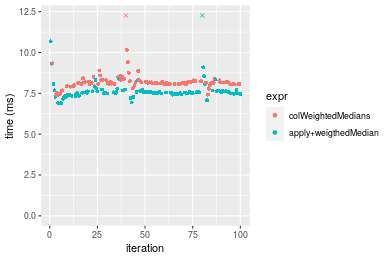
Table: Benchmarking of colWeightedMedians() and rowWeightedMedians() on 100x1000 data (original and transposed). The top panel shows times in milliseconds and the bottom panel shows relative times.
|
expr |
min |
lq |
mean |
median |
uq |
max |
1 |
colWeightedMedians |
7.395820 |
8.068511 |
8.369593 |
8.148709 |
8.241084 |
26.70743 |
2 |
rowWeightedMedians |
7.464479 |
8.106582 |
8.607993 |
8.156696 |
8.231931 |
27.90332 |
|
expr |
min |
lq |
mean |
median |
uq |
max |
1 |
colWeightedMedians |
1.000000 |
1.000000 |
1.000000 |
1.00000 |
1.0000000 |
1.000000 |
2 |
rowWeightedMedians |
1.009284 |
1.004719 |
1.028484 |
1.00098 |
0.9988893 |
1.044778 |
Figure: Benchmarking of colWeightedMedians() and rowWeightedMedians() on 100x1000 data (original and transposed). Outliers are displayed as crosses. Times are in milliseconds.
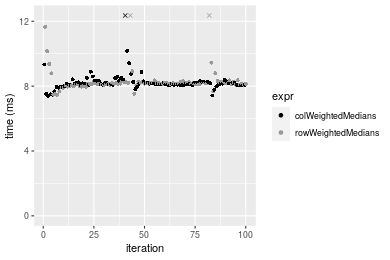
1000x100 matrix
> X <- data[["1000x100"]]
> w <- runif(nrow(X))
> gc()
used (Mb) gc trigger (Mb) max used (Mb)
Ncells 5325154 284.4 8529671 455.6 8529671 455.6
Vcells 10477585 80.0 31876688 243.2 60562128 462.1
> colStats <- microbenchmark(colWeightedMedians = colWeightedMedians(X, w = w, na.rm = FALSE), `apply+weigthedMedian` = apply(X,
+ MARGIN = 2L, FUN = weightedMedian, w = w, na.rm = FALSE), unit = "ms")
> X <- t(X)
> gc()
used (Mb) gc trigger (Mb) max used (Mb)
Ncells 5325142 284.4 8529671 455.6 8529671 455.6
Vcells 10577618 80.8 31876688 243.2 60562128 462.1
> rowStats <- microbenchmark(rowWeightedMedians = rowWeightedMedians(X, w = w, na.rm = FALSE), `apply+weigthedMedian` = apply(X,
+ MARGIN = 1L, FUN = weightedMedian, w = w, na.rm = FALSE), unit = "ms")
Table: Benchmarking of colWeightedMedians() and apply+weigthedMedian() on 1000x100 data. The top panel shows times in milliseconds and the bottom panel shows relative times.
|
expr |
min |
lq |
mean |
median |
uq |
max |
2 |
apply+weigthedMedian |
5.487842 |
5.976517 |
6.143820 |
6.082755 |
6.133801 |
14.53874 |
1 |
colWeightedMedians |
5.581019 |
6.066359 |
6.287458 |
6.192349 |
6.224260 |
15.38716 |
|
expr |
min |
lq |
mean |
median |
uq |
max |
2 |
apply+weigthedMedian |
1.000000 |
1.000000 |
1.000000 |
1.000000 |
1.000000 |
1.000000 |
1 |
colWeightedMedians |
1.016979 |
1.015032 |
1.023379 |
1.018017 |
1.014748 |
1.058356 |
Table: Benchmarking of rowWeightedMedians() and apply+weigthedMedian() on 1000x100 data (transposed). The top panel shows times in milliseconds and the bottom panel shows relative times.
|
expr |
min |
lq |
mean |
median |
uq |
max |
2 |
apply+weigthedMedian |
5.535188 |
6.018122 |
6.206917 |
6.141623 |
6.169907 |
15.39945 |
1 |
rowWeightedMedians |
5.601261 |
6.088288 |
6.376918 |
6.229851 |
6.260240 |
15.11609 |
|
expr |
min |
lq |
mean |
median |
uq |
max |
2 |
apply+weigthedMedian |
1.000000 |
1.000000 |
1.000000 |
1.000000 |
1.000000 |
1.0000000 |
1 |
rowWeightedMedians |
1.011937 |
1.011659 |
1.027389 |
1.014365 |
1.014641 |
0.9815997 |
Figure: Benchmarking of colWeightedMedians() and apply+weigthedMedian() on 1000x100 data as well as rowWeightedMedians() and apply+weigthedMedian() on the same data transposed. Outliers are displayed as crosses. Times are in milliseconds.
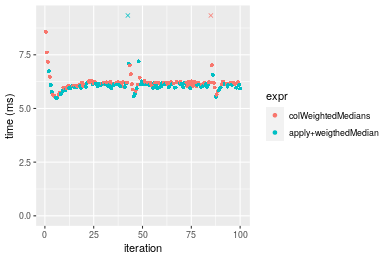
Table: Benchmarking of colWeightedMedians() and rowWeightedMedians() on 1000x100 data (original and transposed). The top panel shows times in milliseconds and the bottom panel shows relative times.
|
expr |
min |
lq |
mean |
median |
uq |
max |
1 |
colWeightedMedians |
5.581019 |
6.066359 |
6.287458 |
6.192349 |
6.22426 |
15.38716 |
2 |
rowWeightedMedians |
5.601261 |
6.088288 |
6.376918 |
6.229851 |
6.26024 |
15.11609 |
|
expr |
min |
lq |
mean |
median |
uq |
max |
1 |
colWeightedMedians |
1.000000 |
1.000000 |
1.000000 |
1.000000 |
1.00000 |
1.0000000 |
2 |
rowWeightedMedians |
1.003627 |
1.003615 |
1.014228 |
1.006056 |
1.00578 |
0.9823835 |
Figure: Benchmarking of colWeightedMedians() and rowWeightedMedians() on 1000x100 data (original and transposed). Outliers are displayed as crosses. Times are in milliseconds.
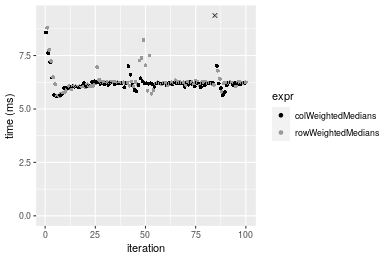
Appendix
R version 4.1.1 Patched (2021-08-10 r80727)
Platform: x86_64-pc-linux-gnu (64-bit)
Running under: Ubuntu 18.04.5 LTS
Matrix products: default
BLAS: /home/hb/software/R-devel/R-4-1-branch/lib/R/lib/libRblas.so
LAPACK: /home/hb/software/R-devel/R-4-1-branch/lib/R/lib/libRlapack.so
locale:
[1] LC_CTYPE=en_US.UTF-8 LC_NUMERIC=C
[3] LC_TIME=en_US.UTF-8 LC_COLLATE=en_US.UTF-8
[5] LC_MONETARY=en_US.UTF-8 LC_MESSAGES=en_US.UTF-8
[7] LC_PAPER=en_US.UTF-8 LC_NAME=C
[9] LC_ADDRESS=C LC_TELEPHONE=C
[11] LC_MEASUREMENT=en_US.UTF-8 LC_IDENTIFICATION=C
attached base packages:
[1] stats graphics grDevices utils datasets methods base
other attached packages:
[1] microbenchmark_1.4-7 matrixStats_0.60.1 ggplot2_3.3.5
[4] knitr_1.33 R.devices_2.17.0 R.utils_2.10.1
[7] R.oo_1.24.0 R.methodsS3_1.8.1-9001 history_0.0.1-9000
loaded via a namespace (and not attached):
[1] Biobase_2.52.0 httr_1.4.2 splines_4.1.1
[4] bit64_4.0.5 network_1.17.1 assertthat_0.2.1
[7] highr_0.9 stats4_4.1.1 blob_1.2.2
[10] GenomeInfoDbData_1.2.6 robustbase_0.93-8 pillar_1.6.2
[13] RSQLite_2.2.8 lattice_0.20-44 glue_1.4.2
[16] digest_0.6.27 XVector_0.32.0 colorspace_2.0-2
[19] Matrix_1.3-4 XML_3.99-0.7 pkgconfig_2.0.3
[22] zlibbioc_1.38.0 genefilter_1.74.0 purrr_0.3.4
[25] ergm_4.1.2 xtable_1.8-4 scales_1.1.1
[28] tibble_3.1.4 annotate_1.70.0 KEGGREST_1.32.0
[31] farver_2.1.0 generics_0.1.0 IRanges_2.26.0
[34] ellipsis_0.3.2 cachem_1.0.6 withr_2.4.2
[37] BiocGenerics_0.38.0 mime_0.11 survival_3.2-13
[40] magrittr_2.0.1 crayon_1.4.1 statnet.common_4.5.0
[43] memoise_2.0.0 laeken_0.5.1 fansi_0.5.0
[46] R.cache_0.15.0 MASS_7.3-54 R.rsp_0.44.0
[49] progressr_0.8.0 tools_4.1.1 lifecycle_1.0.0
[52] S4Vectors_0.30.0 trust_0.1-8 munsell_0.5.0
[55] tabby_0.0.1-9001 AnnotationDbi_1.54.1 Biostrings_2.60.2
[58] compiler_4.1.1 GenomeInfoDb_1.28.1 rlang_0.4.11
[61] grid_4.1.1 RCurl_1.98-1.4 cwhmisc_6.6
[64] rappdirs_0.3.3 startup_0.15.0 labeling_0.4.2
[67] bitops_1.0-7 base64enc_0.1-3 boot_1.3-28
[70] gtable_0.3.0 DBI_1.1.1 markdown_1.1
[73] R6_2.5.1 lpSolveAPI_5.5.2.0-17.7 rle_0.9.2
[76] dplyr_1.0.7 fastmap_1.1.0 bit_4.0.4
[79] utf8_1.2.2 parallel_4.1.1 Rcpp_1.0.7
[82] vctrs_0.3.8 png_0.1-7 DEoptimR_1.0-9
[85] tidyselect_1.1.1 xfun_0.25 coda_0.19-4
Total processing time was 19.23 secs.
Reproducibility
To reproduce this report, do:
html <- matrixStats:::benchmark('colWeightedMedians')
Copyright Henrik Bengtsson. Last updated on 2021-08-25 19:12:19 (+0200 UTC). Powered by RSP.