colWeightedMeans() and rowWeightedMeans() benchmarks
This report benchmark the performance of colWeightedMeans() and rowWeightedMeans() against alternative methods.
Alternative methods
- apply() + weighted.mean()
Data
> rmatrix <- function(nrow, ncol, mode = c("logical", "double", "integer", "index"), range = c(-100,
+ +100), na_prob = 0) {
+ mode <- match.arg(mode)
+ n <- nrow * ncol
+ if (mode == "logical") {
+ x <- sample(c(FALSE, TRUE), size = n, replace = TRUE)
+ } else if (mode == "index") {
+ x <- seq_len(n)
+ mode <- "integer"
+ } else {
+ x <- runif(n, min = range[1], max = range[2])
+ }
+ storage.mode(x) <- mode
+ if (na_prob > 0)
+ x[sample(n, size = na_prob * n)] <- NA
+ dim(x) <- c(nrow, ncol)
+ x
+ }
> rmatrices <- function(scale = 10, seed = 1, ...) {
+ set.seed(seed)
+ data <- list()
+ data[[1]] <- rmatrix(nrow = scale * 1, ncol = scale * 1, ...)
+ data[[2]] <- rmatrix(nrow = scale * 10, ncol = scale * 10, ...)
+ data[[3]] <- rmatrix(nrow = scale * 100, ncol = scale * 1, ...)
+ data[[4]] <- t(data[[3]])
+ data[[5]] <- rmatrix(nrow = scale * 10, ncol = scale * 100, ...)
+ data[[6]] <- t(data[[5]])
+ names(data) <- sapply(data, FUN = function(x) paste(dim(x), collapse = "x"))
+ data
+ }
> data <- rmatrices(mode = "double")
Results
10x10 matrix
> X <- data[["10x10"]]
> w <- runif(nrow(X))
> gc()
used (Mb) gc trigger (Mb) max used (Mb)
Ncells 5329774 284.7 8529671 455.6 8529671 455.6
Vcells 10836871 82.7 31876688 243.2 60562128 462.1
> colStats <- microbenchmark(colWeightedMeans = colWeightedMeans(X, w = w, na.rm = FALSE), `apply+weigthed.mean` = apply(X,
+ MARGIN = 2L, FUN = weighted.mean, w = w, na.rm = FALSE), unit = "ms")
> X <- t(X)
> gc()
used (Mb) gc trigger (Mb) max used (Mb)
Ncells 5319055 284.1 8529671 455.6 8529671 455.6
Vcells 10801549 82.5 31876688 243.2 60562128 462.1
> rowStats <- microbenchmark(rowWeightedMeans = rowWeightedMeans(X, w = w, na.rm = FALSE), `apply+weigthed.mean` = apply(X,
+ MARGIN = 1L, FUN = weighted.mean, w = w, na.rm = FALSE), unit = "ms")
Table: Benchmarking of colWeightedMeans() and apply+weigthed.mean() on 10x10 data. The top panel shows times in milliseconds and the bottom panel shows relative times.
|
expr |
min |
lq |
mean |
median |
uq |
max |
1 |
colWeightedMeans |
0.013956 |
0.0151095 |
0.0173941 |
0.0166140 |
0.0183450 |
0.051339 |
2 |
apply+weigthed.mean |
0.079649 |
0.0828495 |
0.0888316 |
0.0857315 |
0.0885965 |
0.306993 |
|
expr |
min |
lq |
mean |
median |
uq |
max |
1 |
colWeightedMeans |
1.000000 |
1.000000 |
1.000000 |
1.000000 |
1.000000 |
1.000000 |
2 |
apply+weigthed.mean |
5.707151 |
5.483272 |
5.106996 |
5.160196 |
4.829463 |
5.979723 |
Table: Benchmarking of rowWeightedMeans() and apply+weigthed.mean() on 10x10 data (transposed). The top panel shows times in milliseconds and the bottom panel shows relative times.
|
expr |
min |
lq |
mean |
median |
uq |
max |
1 |
rowWeightedMeans |
0.019123 |
0.0217185 |
0.0240152 |
0.023250 |
0.0248235 |
0.066777 |
2 |
apply+weigthed.mean |
0.079402 |
0.0830350 |
0.0891472 |
0.086606 |
0.0915680 |
0.174824 |
|
expr |
min |
lq |
mean |
median |
uq |
max |
1 |
rowWeightedMeans |
1.000000 |
1.000000 |
1.000000 |
1.000000 |
1.000000 |
1.000000 |
2 |
apply+weigthed.mean |
4.152173 |
3.823238 |
3.712118 |
3.724989 |
3.688763 |
2.618027 |
Figure: Benchmarking of colWeightedMeans() and apply+weigthed.mean() on 10x10 data as well as rowWeightedMeans() and apply+weigthed.mean() on the same data transposed. Outliers are displayed as crosses. Times are in milliseconds.
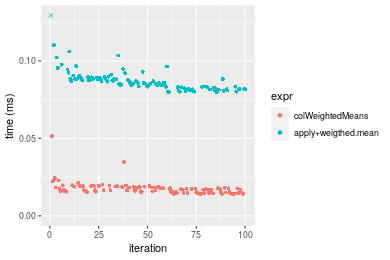
Table: Benchmarking of colWeightedMeans() and rowWeightedMeans() on 10x10 data (original and transposed). The top panel shows times in milliseconds and the bottom panel shows relative times.
|
expr |
min |
lq |
mean |
median |
uq |
max |
1 |
colWeightedMeans |
13.956 |
15.1095 |
17.39410 |
16.614 |
18.3450 |
51.339 |
2 |
rowWeightedMeans |
19.123 |
21.7185 |
24.01519 |
23.250 |
24.8235 |
66.777 |
|
expr |
min |
lq |
mean |
median |
uq |
max |
1 |
colWeightedMeans |
1.000000 |
1.000000 |
1.000000 |
1.000000 |
1.000000 |
1.000000 |
2 |
rowWeightedMeans |
1.370235 |
1.437407 |
1.380651 |
1.399422 |
1.353148 |
1.300707 |
Figure: Benchmarking of colWeightedMeans() and rowWeightedMeans() on 10x10 data (original and transposed). Outliers are displayed as crosses. Times are in milliseconds.
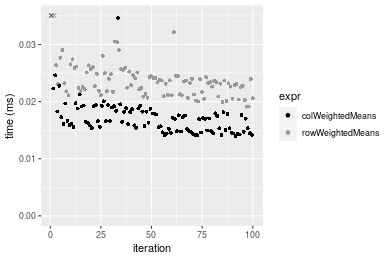
100x100 matrix
> X <- data[["100x100"]]
> w <- runif(nrow(X))
> gc()
used (Mb) gc trigger (Mb) max used (Mb)
Ncells 5317634 284.0 8529671 455.6 8529671 455.6
Vcells 10416411 79.5 31876688 243.2 60562128 462.1
> colStats <- microbenchmark(colWeightedMeans = colWeightedMeans(X, w = w, na.rm = FALSE), `apply+weigthed.mean` = apply(X,
+ MARGIN = 2L, FUN = weighted.mean, w = w, na.rm = FALSE), unit = "ms")
> X <- t(X)
> gc()
used (Mb) gc trigger (Mb) max used (Mb)
Ncells 5317610 284.0 8529671 455.6 8529671 455.6
Vcells 10426424 79.6 31876688 243.2 60562128 462.1
> rowStats <- microbenchmark(rowWeightedMeans = rowWeightedMeans(X, w = w, na.rm = FALSE), `apply+weigthed.mean` = apply(X,
+ MARGIN = 1L, FUN = weighted.mean, w = w, na.rm = FALSE), unit = "ms")
Table: Benchmarking of colWeightedMeans() and apply+weigthed.mean() on 100x100 data. The top panel shows times in milliseconds and the bottom panel shows relative times.
|
expr |
min |
lq |
mean |
median |
uq |
max |
1 |
colWeightedMeans |
0.030232 |
0.0341905 |
0.0415913 |
0.0396795 |
0.0481765 |
0.088324 |
2 |
apply+weigthed.mean |
0.555367 |
0.5899205 |
0.7002991 |
0.6624400 |
0.7478170 |
1.354487 |
|
expr |
min |
lq |
mean |
median |
uq |
max |
1 |
colWeightedMeans |
1.00000 |
1.00000 |
1.00000 |
1.00000 |
1.00000 |
1.00000 |
2 |
apply+weigthed.mean |
18.37017 |
17.25393 |
16.83764 |
16.69477 |
15.52244 |
15.33544 |
Table: Benchmarking of rowWeightedMeans() and apply+weigthed.mean() on 100x100 data (transposed). The top panel shows times in milliseconds and the bottom panel shows relative times.
|
expr |
min |
lq |
mean |
median |
uq |
max |
1 |
rowWeightedMeans |
0.119904 |
0.1255045 |
0.1474997 |
0.142242 |
0.162559 |
0.245560 |
2 |
apply+weigthed.mean |
0.549818 |
0.5672270 |
0.6628345 |
0.641835 |
0.719392 |
1.138727 |
|
expr |
min |
lq |
mean |
median |
uq |
max |
1 |
rowWeightedMeans |
1.000000 |
1.000000 |
1.000000 |
1.000000 |
1.000000 |
1.000000 |
2 |
apply+weigthed.mean |
4.585485 |
4.519575 |
4.493802 |
4.512275 |
4.425421 |
4.637266 |
Figure: Benchmarking of colWeightedMeans() and apply+weigthed.mean() on 100x100 data as well as rowWeightedMeans() and apply+weigthed.mean() on the same data transposed. Outliers are displayed as crosses. Times are in milliseconds.
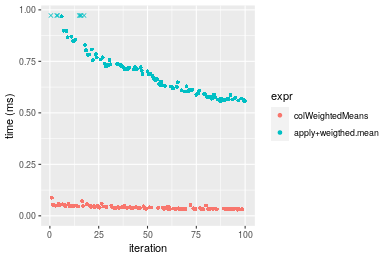
Table: Benchmarking of colWeightedMeans() and rowWeightedMeans() on 100x100 data (original and transposed). The top panel shows times in milliseconds and the bottom panel shows relative times.
|
expr |
min |
lq |
mean |
median |
uq |
max |
1 |
colWeightedMeans |
30.232 |
34.1905 |
41.59129 |
39.6795 |
48.1765 |
88.324 |
2 |
rowWeightedMeans |
119.904 |
125.5045 |
147.49972 |
142.2420 |
162.5590 |
245.560 |
|
expr |
min |
lq |
mean |
median |
uq |
max |
1 |
colWeightedMeans |
1.000000 |
1.000000 |
1.000000 |
1.000000 |
1.000000 |
1.000000 |
2 |
rowWeightedMeans |
3.966129 |
3.670742 |
3.546409 |
3.584773 |
3.374239 |
2.780218 |
Figure: Benchmarking of colWeightedMeans() and rowWeightedMeans() on 100x100 data (original and transposed). Outliers are displayed as crosses. Times are in milliseconds.
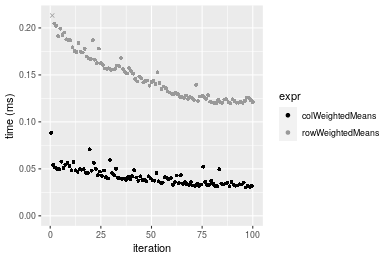
1000x10 matrix
> X <- data[["1000x10"]]
> w <- runif(nrow(X))
> gc()
used (Mb) gc trigger (Mb) max used (Mb)
Ncells 5318335 284.1 8529671 455.6 8529671 455.6
Vcells 10420778 79.6 31876688 243.2 60562128 462.1
> colStats <- microbenchmark(colWeightedMeans = colWeightedMeans(X, w = w, na.rm = FALSE), `apply+weigthed.mean` = apply(X,
+ MARGIN = 2L, FUN = weighted.mean, w = w, na.rm = FALSE), unit = "ms")
> X <- t(X)
> gc()
used (Mb) gc trigger (Mb) max used (Mb)
Ncells 5318329 284.1 8529671 455.6 8529671 455.6
Vcells 10430821 79.6 31876688 243.2 60562128 462.1
> rowStats <- microbenchmark(rowWeightedMeans = rowWeightedMeans(X, w = w, na.rm = FALSE), `apply+weigthed.mean` = apply(X,
+ MARGIN = 1L, FUN = weighted.mean, w = w, na.rm = FALSE), unit = "ms")
Table: Benchmarking of colWeightedMeans() and apply+weigthed.mean() on 1000x10 data. The top panel shows times in milliseconds and the bottom panel shows relative times.
|
expr |
min |
lq |
mean |
median |
uq |
max |
1 |
colWeightedMeans |
0.049971 |
0.0548630 |
0.0609515 |
0.0588590 |
0.0650855 |
0.135331 |
2 |
apply+weigthed.mean |
0.211792 |
0.2281235 |
0.2468247 |
0.2400965 |
0.2623080 |
0.355333 |
|
expr |
min |
lq |
mean |
median |
uq |
max |
1 |
colWeightedMeans |
1.000000 |
1.000000 |
1.000000 |
1.000000 |
1.000000 |
1.000000 |
2 |
apply+weigthed.mean |
4.238298 |
4.158057 |
4.049527 |
4.079181 |
4.030206 |
2.625659 |
Table: Benchmarking of rowWeightedMeans() and apply+weigthed.mean() on 1000x10 data (transposed). The top panel shows times in milliseconds and the bottom panel shows relative times.
|
expr |
min |
lq |
mean |
median |
uq |
max |
1 |
rowWeightedMeans |
0.152673 |
0.1696105 |
0.1855038 |
0.1826945 |
0.1975075 |
0.304953 |
2 |
apply+weigthed.mean |
0.195234 |
0.2165075 |
0.2399114 |
0.2333165 |
0.2564390 |
0.351846 |
|
expr |
min |
lq |
mean |
median |
uq |
max |
1 |
rowWeightedMeans |
1.000000 |
1.000000 |
1.000000 |
1.000000 |
1.000000 |
1.000000 |
2 |
apply+weigthed.mean |
1.278772 |
1.276498 |
1.293296 |
1.277086 |
1.298376 |
1.153771 |
Figure: Benchmarking of colWeightedMeans() and apply+weigthed.mean() on 1000x10 data as well as rowWeightedMeans() and apply+weigthed.mean() on the same data transposed. Outliers are displayed as crosses. Times are in milliseconds.
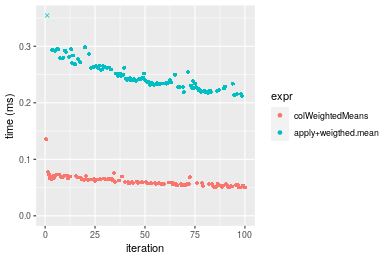
Table: Benchmarking of colWeightedMeans() and rowWeightedMeans() on 1000x10 data (original and transposed). The top panel shows times in milliseconds and the bottom panel shows relative times.
|
expr |
min |
lq |
mean |
median |
uq |
max |
1 |
colWeightedMeans |
49.971 |
54.8630 |
60.9515 |
58.8590 |
65.0855 |
135.331 |
2 |
rowWeightedMeans |
152.673 |
169.6105 |
185.5038 |
182.6945 |
197.5075 |
304.953 |
|
expr |
min |
lq |
mean |
median |
uq |
max |
1 |
colWeightedMeans |
1.000000 |
1.000000 |
1.000000 |
1.000000 |
1.000000 |
1.000000 |
2 |
rowWeightedMeans |
3.055232 |
3.091528 |
3.043466 |
3.103935 |
3.034585 |
2.253386 |
Figure: Benchmarking of colWeightedMeans() and rowWeightedMeans() on 1000x10 data (original and transposed). Outliers are displayed as crosses. Times are in milliseconds.
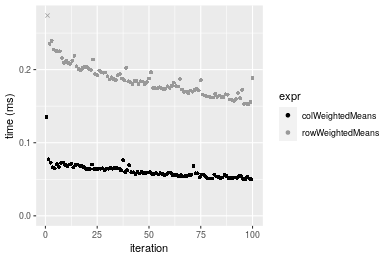
10x1000 matrix
> X <- data[["10x1000"]]
> w <- runif(nrow(X))
> gc()
used (Mb) gc trigger (Mb) max used (Mb)
Ncells 5318551 284.1 8529671 455.6 8529671 455.6
Vcells 10420605 79.6 31876688 243.2 60562128 462.1
> colStats <- microbenchmark(colWeightedMeans = colWeightedMeans(X, w = w, na.rm = FALSE), `apply+weigthed.mean` = apply(X,
+ MARGIN = 2L, FUN = weighted.mean, w = w, na.rm = FALSE), unit = "ms")
> X <- t(X)
> gc()
used (Mb) gc trigger (Mb) max used (Mb)
Ncells 5318527 284.1 8529671 455.6 8529671 455.6
Vcells 10430618 79.6 31876688 243.2 60562128 462.1
> rowStats <- microbenchmark(rowWeightedMeans = rowWeightedMeans(X, w = w, na.rm = FALSE), `apply+weigthed.mean` = apply(X,
+ MARGIN = 1L, FUN = weighted.mean, w = w, na.rm = FALSE), unit = "ms")
Table: Benchmarking of colWeightedMeans() and apply+weigthed.mean() on 10x1000 data. The top panel shows times in milliseconds and the bottom panel shows relative times.
|
expr |
min |
lq |
mean |
median |
uq |
max |
1 |
colWeightedMeans |
0.029489 |
0.032739 |
0.0394763 |
0.0368465 |
0.042318 |
0.10219 |
2 |
apply+weigthed.mean |
4.084716 |
4.528123 |
4.8010556 |
4.6832870 |
4.777128 |
11.05300 |
|
expr |
min |
lq |
mean |
median |
uq |
max |
1 |
colWeightedMeans |
1.0000 |
1.0000 |
1.0000 |
1.0000 |
1.0000 |
1.0000 |
2 |
apply+weigthed.mean |
138.5166 |
138.3097 |
121.6186 |
127.1026 |
112.8864 |
108.1613 |
Table: Benchmarking of rowWeightedMeans() and apply+weigthed.mean() on 10x1000 data (transposed). The top panel shows times in milliseconds and the bottom panel shows relative times.
|
expr |
min |
lq |
mean |
median |
uq |
max |
1 |
rowWeightedMeans |
0.118433 |
0.1310465 |
0.1392433 |
0.137177 |
0.142301 |
0.223296 |
2 |
apply+weigthed.mean |
4.093245 |
4.5486995 |
4.8070154 |
4.706437 |
4.788188 |
11.003123 |
|
expr |
min |
lq |
mean |
median |
uq |
max |
1 |
rowWeightedMeans |
1.00000 |
1.00000 |
1.00000 |
1.00000 |
1.00000 |
1.00000 |
2 |
apply+weigthed.mean |
34.56169 |
34.71058 |
34.52242 |
34.30923 |
33.64831 |
49.27595 |
Figure: Benchmarking of colWeightedMeans() and apply+weigthed.mean() on 10x1000 data as well as rowWeightedMeans() and apply+weigthed.mean() on the same data transposed. Outliers are displayed as crosses. Times are in milliseconds.
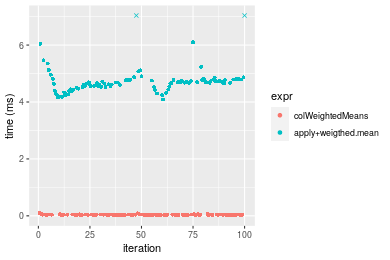
Table: Benchmarking of colWeightedMeans() and rowWeightedMeans() on 10x1000 data (original and transposed). The top panel shows times in milliseconds and the bottom panel shows relative times.
|
expr |
min |
lq |
mean |
median |
uq |
max |
1 |
colWeightedMeans |
29.489 |
32.7390 |
39.47633 |
36.8465 |
42.318 |
102.190 |
2 |
rowWeightedMeans |
118.433 |
131.0465 |
139.24328 |
137.1770 |
142.301 |
223.296 |
|
expr |
min |
lq |
mean |
median |
uq |
max |
1 |
colWeightedMeans |
1.000000 |
1.000000 |
1.00000 |
1.000000 |
1.000000 |
1.000000 |
2 |
rowWeightedMeans |
4.016176 |
4.002764 |
3.52726 |
3.722932 |
3.362659 |
2.185106 |
Figure: Benchmarking of colWeightedMeans() and rowWeightedMeans() on 10x1000 data (original and transposed). Outliers are displayed as crosses. Times are in milliseconds.
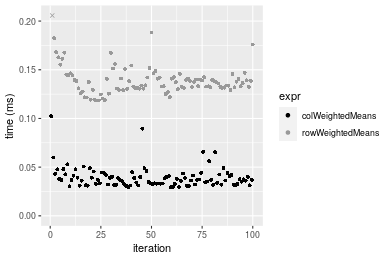
100x1000 matrix
> X <- data[["100x1000"]]
> w <- runif(nrow(X))
> gc()
used (Mb) gc trigger (Mb) max used (Mb)
Ncells 5318741 284.1 8529671 455.6 8529671 455.6
Vcells 10421196 79.6 31876688 243.2 60562128 462.1
> colStats <- microbenchmark(colWeightedMeans = colWeightedMeans(X, w = w, na.rm = FALSE), `apply+weigthed.mean` = apply(X,
+ MARGIN = 2L, FUN = weighted.mean, w = w, na.rm = FALSE), unit = "ms")
> X <- t(X)
> gc()
used (Mb) gc trigger (Mb) max used (Mb)
Ncells 5318717 284.1 8529671 455.6 8529671 455.6
Vcells 10521209 80.3 31876688 243.2 60562128 462.1
> rowStats <- microbenchmark(rowWeightedMeans = rowWeightedMeans(X, w = w, na.rm = FALSE), `apply+weigthed.mean` = apply(X,
+ MARGIN = 1L, FUN = weighted.mean, w = w, na.rm = FALSE), unit = "ms")
Table: Benchmarking of colWeightedMeans() and apply+weigthed.mean() on 100x1000 data. The top panel shows times in milliseconds and the bottom panel shows relative times.
|
expr |
min |
lq |
mean |
median |
uq |
max |
1 |
colWeightedMeans |
0.185700 |
0.219297 |
0.4396765 |
0.2470125 |
0.272899 |
19.48006 |
2 |
apply+weigthed.mean |
5.412697 |
5.866960 |
6.5537505 |
5.9813235 |
6.263001 |
28.49123 |
|
expr |
min |
lq |
mean |
median |
uq |
max |
1 |
colWeightedMeans |
1.00000 |
1.00000 |
1.00000 |
1.00000 |
1.00000 |
1.000000 |
2 |
apply+weigthed.mean |
29.14753 |
26.75349 |
14.90585 |
24.21466 |
22.94989 |
1.462584 |
Table: Benchmarking of rowWeightedMeans() and apply+weigthed.mean() on 100x1000 data (transposed). The top panel shows times in milliseconds and the bottom panel shows relative times.
|
expr |
min |
lq |
mean |
median |
uq |
max |
1 |
rowWeightedMeans |
1.054020 |
1.153162 |
1.269207 |
1.182492 |
1.305193 |
2.109278 |
2 |
apply+weigthed.mean |
5.405374 |
5.879766 |
7.117222 |
6.029890 |
6.585137 |
31.438123 |
|
expr |
min |
lq |
mean |
median |
uq |
max |
1 |
rowWeightedMeans |
1.000000 |
1.000000 |
1.000000 |
1.000000 |
1.000000 |
1.00000 |
2 |
apply+weigthed.mean |
5.128341 |
5.098821 |
5.607613 |
5.099308 |
5.045336 |
14.90468 |
Figure: Benchmarking of colWeightedMeans() and apply+weigthed.mean() on 100x1000 data as well as rowWeightedMeans() and apply+weigthed.mean() on the same data transposed. Outliers are displayed as crosses. Times are in milliseconds.
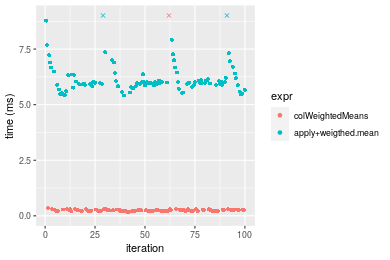
Table: Benchmarking of colWeightedMeans() and rowWeightedMeans() on 100x1000 data (original and transposed). The top panel shows times in milliseconds and the bottom panel shows relative times.
|
expr |
min |
lq |
mean |
median |
uq |
max |
1 |
colWeightedMeans |
185.70 |
219.297 |
439.6765 |
247.0125 |
272.899 |
19480.060 |
2 |
rowWeightedMeans |
1054.02 |
1153.162 |
1269.2071 |
1182.4920 |
1305.193 |
2109.278 |
|
expr |
min |
lq |
mean |
median |
uq |
max |
1 |
colWeightedMeans |
1.000000 |
1.000000 |
1.000000 |
1.000000 |
1.000000 |
1.0000000 |
2 |
rowWeightedMeans |
5.675929 |
5.258449 |
2.886684 |
4.787175 |
4.782696 |
0.1082788 |
Figure: Benchmarking of colWeightedMeans() and rowWeightedMeans() on 100x1000 data (original and transposed). Outliers are displayed as crosses. Times are in milliseconds.
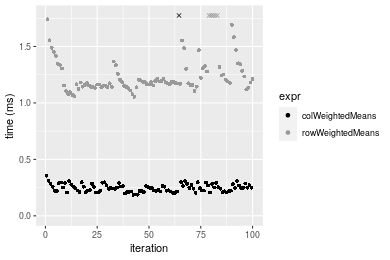
1000x100 matrix
> X <- data[["1000x100"]]
> w <- runif(nrow(X))
> gc()
used (Mb) gc trigger (Mb) max used (Mb)
Ncells 5318910 284.1 8529671 455.6 8529671 455.6
Vcells 10422719 79.6 31876688 243.2 60562128 462.1
> colStats <- microbenchmark(colWeightedMeans = colWeightedMeans(X, w = w, na.rm = FALSE), `apply+weigthed.mean` = apply(X,
+ MARGIN = 2L, FUN = weighted.mean, w = w, na.rm = FALSE), unit = "ms")
> X <- t(X)
> gc()
used (Mb) gc trigger (Mb) max used (Mb)
Ncells 5318904 284.1 8529671 455.6 8529671 455.6
Vcells 10522762 80.3 31876688 243.2 60562128 462.1
> rowStats <- microbenchmark(rowWeightedMeans = rowWeightedMeans(X, w = w, na.rm = FALSE), `apply+weigthed.mean` = apply(X,
+ MARGIN = 1L, FUN = weighted.mean, w = w, na.rm = FALSE), unit = "ms")
Table: Benchmarking of colWeightedMeans() and apply+weigthed.mean() on 1000x100 data. The top panel shows times in milliseconds and the bottom panel shows relative times.
|
expr |
min |
lq |
mean |
median |
uq |
max |
1 |
colWeightedMeans |
0.205215 |
0.2276095 |
0.3425132 |
0.245658 |
0.2785555 |
8.429282 |
2 |
apply+weigthed.mean |
1.540183 |
1.6469045 |
2.0503194 |
1.837805 |
2.0657935 |
10.520204 |
|
expr |
min |
lq |
mean |
median |
uq |
max |
1 |
colWeightedMeans |
1.000000 |
1.000000 |
1.000000 |
1.000000 |
1.000000 |
1.000000 |
2 |
apply+weigthed.mean |
7.505217 |
7.235658 |
5.986104 |
7.481153 |
7.416093 |
1.248055 |
Table: Benchmarking of rowWeightedMeans() and apply+weigthed.mean() on 1000x100 data (transposed). The top panel shows times in milliseconds and the bottom panel shows relative times.
|
expr |
min |
lq |
mean |
median |
uq |
max |
1 |
rowWeightedMeans |
1.068420 |
1.125180 |
1.245604 |
1.194421 |
1.330394 |
1.94012 |
2 |
apply+weigthed.mean |
1.561156 |
1.628209 |
2.060293 |
1.714316 |
1.977224 |
10.16854 |
|
expr |
min |
lq |
mean |
median |
uq |
max |
1 |
rowWeightedMeans |
1.000000 |
1.000000 |
1.000000 |
1.000000 |
1.000000 |
1.000000 |
2 |
apply+weigthed.mean |
1.461182 |
1.447065 |
1.654052 |
1.435269 |
1.486195 |
5.241191 |
Figure: Benchmarking of colWeightedMeans() and apply+weigthed.mean() on 1000x100 data as well as rowWeightedMeans() and apply+weigthed.mean() on the same data transposed. Outliers are displayed as crosses. Times are in milliseconds.
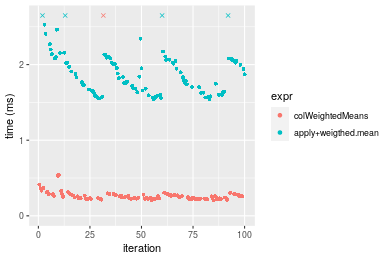
Table: Benchmarking of colWeightedMeans() and rowWeightedMeans() on 1000x100 data (original and transposed). The top panel shows times in milliseconds and the bottom panel shows relative times.
|
expr |
min |
lq |
mean |
median |
uq |
max |
1 |
colWeightedMeans |
205.215 |
227.6095 |
342.5132 |
245.658 |
278.5555 |
8429.282 |
2 |
rowWeightedMeans |
1068.420 |
1125.1800 |
1245.6036 |
1194.421 |
1330.3935 |
1940.120 |
|
expr |
min |
lq |
mean |
median |
uq |
max |
1 |
colWeightedMeans |
1.000000 |
1.000000 |
1.000000 |
1.000000 |
1.000000 |
1.0000000 |
2 |
rowWeightedMeans |
5.206345 |
4.943467 |
3.636659 |
4.862129 |
4.776045 |
0.2301643 |
Figure: Benchmarking of colWeightedMeans() and rowWeightedMeans() on 1000x100 data (original and transposed). Outliers are displayed as crosses. Times are in milliseconds.
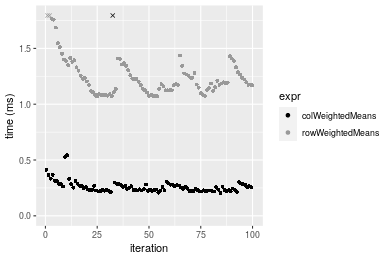
Appendix
R version 4.1.1 Patched (2021-08-10 r80727)
Platform: x86_64-pc-linux-gnu (64-bit)
Running under: Ubuntu 18.04.5 LTS
Matrix products: default
BLAS: /home/hb/software/R-devel/R-4-1-branch/lib/R/lib/libRblas.so
LAPACK: /home/hb/software/R-devel/R-4-1-branch/lib/R/lib/libRlapack.so
locale:
[1] LC_CTYPE=en_US.UTF-8 LC_NUMERIC=C
[3] LC_TIME=en_US.UTF-8 LC_COLLATE=en_US.UTF-8
[5] LC_MONETARY=en_US.UTF-8 LC_MESSAGES=en_US.UTF-8
[7] LC_PAPER=en_US.UTF-8 LC_NAME=C
[9] LC_ADDRESS=C LC_TELEPHONE=C
[11] LC_MEASUREMENT=en_US.UTF-8 LC_IDENTIFICATION=C
attached base packages:
[1] stats graphics grDevices utils datasets methods base
other attached packages:
[1] microbenchmark_1.4-7 matrixStats_0.60.1 ggplot2_3.3.5
[4] knitr_1.33 R.devices_2.17.0 R.utils_2.10.1
[7] R.oo_1.24.0 R.methodsS3_1.8.1-9001 history_0.0.1-9000
loaded via a namespace (and not attached):
[1] Biobase_2.52.0 httr_1.4.2 splines_4.1.1
[4] bit64_4.0.5 network_1.17.1 assertthat_0.2.1
[7] highr_0.9 stats4_4.1.1 blob_1.2.2
[10] GenomeInfoDbData_1.2.6 robustbase_0.93-8 pillar_1.6.2
[13] RSQLite_2.2.8 lattice_0.20-44 glue_1.4.2
[16] digest_0.6.27 XVector_0.32.0 colorspace_2.0-2
[19] Matrix_1.3-4 XML_3.99-0.7 pkgconfig_2.0.3
[22] zlibbioc_1.38.0 genefilter_1.74.0 purrr_0.3.4
[25] ergm_4.1.2 xtable_1.8-4 scales_1.1.1
[28] tibble_3.1.4 annotate_1.70.0 KEGGREST_1.32.0
[31] farver_2.1.0 generics_0.1.0 IRanges_2.26.0
[34] ellipsis_0.3.2 cachem_1.0.6 withr_2.4.2
[37] BiocGenerics_0.38.0 mime_0.11 survival_3.2-13
[40] magrittr_2.0.1 crayon_1.4.1 statnet.common_4.5.0
[43] memoise_2.0.0 laeken_0.5.1 fansi_0.5.0
[46] R.cache_0.15.0 MASS_7.3-54 R.rsp_0.44.0
[49] progressr_0.8.0 tools_4.1.1 lifecycle_1.0.0
[52] S4Vectors_0.30.0 trust_0.1-8 munsell_0.5.0
[55] tabby_0.0.1-9001 AnnotationDbi_1.54.1 Biostrings_2.60.2
[58] compiler_4.1.1 GenomeInfoDb_1.28.1 rlang_0.4.11
[61] grid_4.1.1 RCurl_1.98-1.4 cwhmisc_6.6
[64] rappdirs_0.3.3 startup_0.15.0 labeling_0.4.2
[67] bitops_1.0-7 base64enc_0.1-3 boot_1.3-28
[70] gtable_0.3.0 DBI_1.1.1 markdown_1.1
[73] R6_2.5.1 lpSolveAPI_5.5.2.0-17.7 rle_0.9.2
[76] dplyr_1.0.7 fastmap_1.1.0 bit_4.0.4
[79] utf8_1.2.2 parallel_4.1.1 Rcpp_1.0.7
[82] vctrs_0.3.8 png_0.1-7 DEoptimR_1.0-9
[85] tidyselect_1.1.1 xfun_0.25 coda_0.19-4
Total processing time was 14.77 secs.
Reproducibility
To reproduce this report, do:
html <- matrixStats:::benchmark('colWeightedMeans')
Copyright Henrik Bengtsson. Last updated on 2021-08-25 19:11:39 (+0200 UTC). Powered by RSP.