colQuantiles() and rowQuantiles() benchmarks
This report benchmark the performance of colQuantiles() and rowQuantiles() against alternative methods.
Alternative methods
Data
> rmatrix <- function(nrow, ncol, mode = c("logical", "double", "integer", "index"), range = c(-100,
+ +100), na_prob = 0) {
+ mode <- match.arg(mode)
+ n <- nrow * ncol
+ if (mode == "logical") {
+ x <- sample(c(FALSE, TRUE), size = n, replace = TRUE)
+ } else if (mode == "index") {
+ x <- seq_len(n)
+ mode <- "integer"
+ } else {
+ x <- runif(n, min = range[1], max = range[2])
+ }
+ storage.mode(x) <- mode
+ if (na_prob > 0)
+ x[sample(n, size = na_prob * n)] <- NA
+ dim(x) <- c(nrow, ncol)
+ x
+ }
> rmatrices <- function(scale = 10, seed = 1, ...) {
+ set.seed(seed)
+ data <- list()
+ data[[1]] <- rmatrix(nrow = scale * 1, ncol = scale * 1, ...)
+ data[[2]] <- rmatrix(nrow = scale * 10, ncol = scale * 10, ...)
+ data[[3]] <- rmatrix(nrow = scale * 100, ncol = scale * 1, ...)
+ data[[4]] <- t(data[[3]])
+ data[[5]] <- rmatrix(nrow = scale * 10, ncol = scale * 100, ...)
+ data[[6]] <- t(data[[5]])
+ names(data) <- sapply(data, FUN = function(x) paste(dim(x), collapse = "x"))
+ data
+ }
> data <- rmatrices(mode = "double")
Results
10x10 matrix
> X <- data[["10x10"]]
> gc()
used (Mb) gc trigger (Mb) max used (Mb)
Ncells 5282673 282.2 8529671 455.6 8529671 455.6
Vcells 10520122 80.3 31876688 243.2 60562128 462.1
> probs <- seq(from = 0, to = 1, by = 0.25)
> colStats <- microbenchmark(colQuantiles = colQuantiles(X, probs = probs, na.rm = FALSE), `apply+quantile` = apply(X,
+ MARGIN = 2L, FUN = quantile, probs = probs, na.rm = FALSE), unit = "ms")
> X <- t(X)
> gc()
used (Mb) gc trigger (Mb) max used (Mb)
Ncells 5272450 281.6 8529671 455.6 8529671 455.6
Vcells 10486542 80.1 31876688 243.2 60562128 462.1
> rowStats <- microbenchmark(rowQuantiles = rowQuantiles(X, probs = probs, na.rm = FALSE), `apply+quantile` = apply(X,
+ MARGIN = 1L, FUN = quantile, probs = probs, na.rm = FALSE), unit = "ms")
Table: Benchmarking of colQuantiles() and apply+quantile() on 10x10 data. The top panel shows times in milliseconds and the bottom panel shows relative times.
|
expr |
min |
lq |
mean |
median |
uq |
max |
1 |
colQuantiles |
0.240633 |
0.2628575 |
0.2890274 |
0.277496 |
0.3025895 |
0.479952 |
2 |
apply+quantile |
1.060257 |
1.1672905 |
1.2570903 |
1.212705 |
1.2817150 |
2.025870 |
|
expr |
min |
lq |
mean |
median |
uq |
max |
1 |
colQuantiles |
1.000000 |
1.000000 |
1.000000 |
1.000000 |
1.000000 |
1.000000 |
2 |
apply+quantile |
4.406116 |
4.440773 |
4.349382 |
4.370173 |
4.235821 |
4.220985 |
Table: Benchmarking of rowQuantiles() and apply+quantile() on 10x10 data (transposed). The top panel shows times in milliseconds and the bottom panel shows relative times.
|
expr |
min |
lq |
mean |
median |
uq |
max |
1 |
rowQuantiles |
0.235269 |
0.2596625 |
0.2863194 |
0.2733315 |
0.302111 |
0.468712 |
2 |
apply+quantile |
1.069531 |
1.1232670 |
1.2350757 |
1.1880585 |
1.277098 |
2.041848 |
|
expr |
min |
lq |
mean |
median |
uq |
max |
1 |
rowQuantiles |
1.000000 |
1.000000 |
1.000000 |
1.000000 |
1.000000 |
1.000000 |
2 |
apply+quantile |
4.545992 |
4.325873 |
4.313629 |
4.346585 |
4.227248 |
4.356296 |
Figure: Benchmarking of colQuantiles() and apply+quantile() on 10x10 data as well as rowQuantiles() and apply+quantile() on the same data transposed. Outliers are displayed as crosses. Times are in milliseconds.
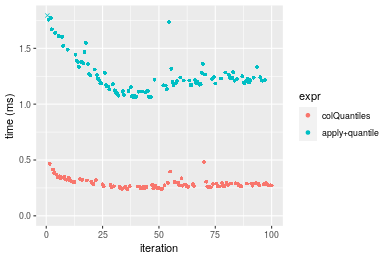
Table: Benchmarking of colQuantiles() and rowQuantiles() on 10x10 data (original and transposed). The top panel shows times in milliseconds and the bottom panel shows relative times.
|
expr |
min |
lq |
mean |
median |
uq |
max |
2 |
rowQuantiles |
235.269 |
259.6625 |
286.3194 |
273.3315 |
302.1110 |
468.712 |
1 |
colQuantiles |
240.633 |
262.8575 |
289.0274 |
277.4960 |
302.5895 |
479.952 |
|
expr |
min |
lq |
mean |
median |
uq |
max |
2 |
rowQuantiles |
1.000000 |
1.000000 |
1.000000 |
1.000000 |
1.000000 |
1.000000 |
1 |
colQuantiles |
1.022799 |
1.012304 |
1.009458 |
1.015236 |
1.001584 |
1.023981 |
Figure: Benchmarking of colQuantiles() and rowQuantiles() on 10x10 data (original and transposed). Outliers are displayed as crosses. Times are in milliseconds.
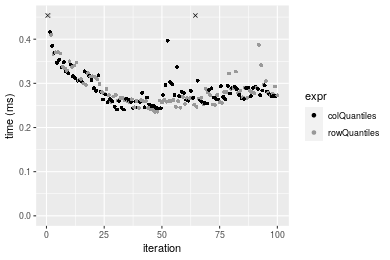
100x100 matrix
> X <- data[["100x100"]]
> gc()
used (Mb) gc trigger (Mb) max used (Mb)
Ncells 5271016 281.6 8529671 455.6 8529671 455.6
Vcells 10102985 77.1 31876688 243.2 60562128 462.1
> probs <- seq(from = 0, to = 1, by = 0.25)
> colStats <- microbenchmark(colQuantiles = colQuantiles(X, probs = probs, na.rm = FALSE), `apply+quantile` = apply(X,
+ MARGIN = 2L, FUN = quantile, probs = probs, na.rm = FALSE), unit = "ms")
> X <- t(X)
> gc()
used (Mb) gc trigger (Mb) max used (Mb)
Ncells 5271004 281.6 8529671 455.6 8529671 455.6
Vcells 10113018 77.2 31876688 243.2 60562128 462.1
> rowStats <- microbenchmark(rowQuantiles = rowQuantiles(X, probs = probs, na.rm = FALSE), `apply+quantile` = apply(X,
+ MARGIN = 1L, FUN = quantile, probs = probs, na.rm = FALSE), unit = "ms")
Table: Benchmarking of colQuantiles() and apply+quantile() on 100x100 data. The top panel shows times in milliseconds and the bottom panel shows relative times.
|
expr |
min |
lq |
mean |
median |
uq |
max |
1 |
colQuantiles |
1.888284 |
2.136058 |
2.195115 |
2.186279 |
2.243244 |
3.22735 |
2 |
apply+quantile |
10.923141 |
12.262892 |
12.932966 |
12.499504 |
12.717576 |
33.25679 |
|
expr |
min |
lq |
mean |
median |
uq |
max |
1 |
colQuantiles |
1.000000 |
1.000000 |
1.000000 |
1.00000 |
1.000000 |
1.00000 |
2 |
apply+quantile |
5.784692 |
5.740897 |
5.891703 |
5.71725 |
5.669279 |
10.30467 |
Table: Benchmarking of rowQuantiles() and apply+quantile() on 100x100 data (transposed). The top panel shows times in milliseconds and the bottom panel shows relative times.
|
expr |
min |
lq |
mean |
median |
uq |
max |
1 |
rowQuantiles |
1.947457 |
2.172823 |
2.370571 |
2.23365 |
2.274193 |
10.16345 |
2 |
apply+quantile |
10.936896 |
12.217553 |
12.536485 |
12.40464 |
12.653492 |
21.03754 |
|
expr |
min |
lq |
mean |
median |
uq |
max |
1 |
rowQuantiles |
1.000000 |
1.000000 |
1.000000 |
1.000000 |
1.000000 |
1.00000 |
2 |
apply+quantile |
5.615988 |
5.622895 |
5.288382 |
5.553526 |
5.563948 |
2.06992 |
Figure: Benchmarking of colQuantiles() and apply+quantile() on 100x100 data as well as rowQuantiles() and apply+quantile() on the same data transposed. Outliers are displayed as crosses. Times are in milliseconds.
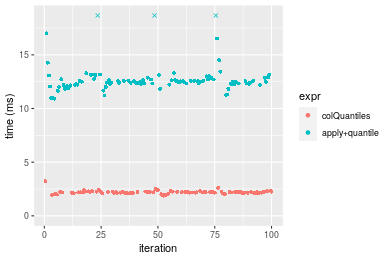
Table: Benchmarking of colQuantiles() and rowQuantiles() on 100x100 data (original and transposed). The top panel shows times in milliseconds and the bottom panel shows relative times.
|
expr |
min |
lq |
mean |
median |
uq |
max |
1 |
colQuantiles |
1.888284 |
2.136058 |
2.195115 |
2.186279 |
2.243244 |
3.22735 |
2 |
rowQuantiles |
1.947457 |
2.172823 |
2.370571 |
2.233650 |
2.274193 |
10.16345 |
|
expr |
min |
lq |
mean |
median |
uq |
max |
1 |
colQuantiles |
1.000000 |
1.000000 |
1.00000 |
1.000000 |
1.000000 |
1.000000 |
2 |
rowQuantiles |
1.031337 |
1.017211 |
1.07993 |
1.021668 |
1.013796 |
3.149164 |
Figure: Benchmarking of colQuantiles() and rowQuantiles() on 100x100 data (original and transposed). Outliers are displayed as crosses. Times are in milliseconds.
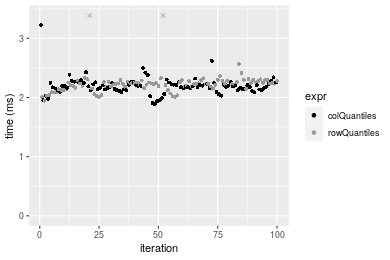
1000x10 matrix
> X <- data[["1000x10"]]
> gc()
used (Mb) gc trigger (Mb) max used (Mb)
Ncells 5271758 281.6 8529671 455.6 8529671 455.6
Vcells 10106514 77.2 31876688 243.2 60562128 462.1
> probs <- seq(from = 0, to = 1, by = 0.25)
> colStats <- microbenchmark(colQuantiles = colQuantiles(X, probs = probs, na.rm = FALSE), `apply+quantile` = apply(X,
+ MARGIN = 2L, FUN = quantile, probs = probs, na.rm = FALSE), unit = "ms")
> X <- t(X)
> gc()
used (Mb) gc trigger (Mb) max used (Mb)
Ncells 5271734 281.6 8529671 455.6 8529671 455.6
Vcells 10116527 77.2 31876688 243.2 60562128 462.1
> rowStats <- microbenchmark(rowQuantiles = rowQuantiles(X, probs = probs, na.rm = FALSE), `apply+quantile` = apply(X,
+ MARGIN = 1L, FUN = quantile, probs = probs, na.rm = FALSE), unit = "ms")
Table: Benchmarking of colQuantiles() and apply+quantile() on 1000x10 data. The top panel shows times in milliseconds and the bottom panel shows relative times.
|
expr |
min |
lq |
mean |
median |
uq |
max |
1 |
colQuantiles |
0.601190 |
0.6562685 |
0.6981146 |
0.6862355 |
0.705663 |
1.156208 |
2 |
apply+quantile |
1.503947 |
1.6398185 |
1.7177534 |
1.7044685 |
1.746963 |
2.498938 |
|
expr |
min |
lq |
mean |
median |
uq |
max |
1 |
colQuantiles |
1.000000 |
1.000000 |
1.000000 |
1.000000 |
1.000000 |
1.000000 |
2 |
apply+quantile |
2.501617 |
2.498701 |
2.460561 |
2.483795 |
2.475634 |
2.161322 |
Table: Benchmarking of rowQuantiles() and apply+quantile() on 1000x10 data (transposed). The top panel shows times in milliseconds and the bottom panel shows relative times.
|
expr |
min |
lq |
mean |
median |
uq |
max |
1 |
rowQuantiles |
0.643236 |
0.694242 |
0.7400125 |
0.7194075 |
0.7373645 |
1.230742 |
2 |
apply+quantile |
1.527078 |
1.654772 |
1.7342194 |
1.7038935 |
1.7511335 |
2.642374 |
|
expr |
min |
lq |
mean |
median |
uq |
max |
1 |
rowQuantiles |
1.000000 |
1.000000 |
1.0000 |
1.000000 |
1.000000 |
1.000000 |
2 |
apply+quantile |
2.374056 |
2.383567 |
2.3435 |
2.368468 |
2.374855 |
2.146976 |
Figure: Benchmarking of colQuantiles() and apply+quantile() on 1000x10 data as well as rowQuantiles() and apply+quantile() on the same data transposed. Outliers are displayed as crosses. Times are in milliseconds.
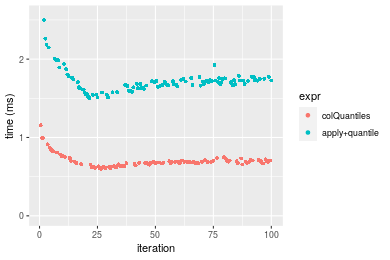
Table: Benchmarking of colQuantiles() and rowQuantiles() on 1000x10 data (original and transposed). The top panel shows times in milliseconds and the bottom panel shows relative times.
|
expr |
min |
lq |
mean |
median |
uq |
max |
1 |
colQuantiles |
601.190 |
656.2685 |
698.1146 |
686.2355 |
705.6630 |
1156.208 |
2 |
rowQuantiles |
643.236 |
694.2420 |
740.0125 |
719.4075 |
737.3645 |
1230.742 |
|
expr |
min |
lq |
mean |
median |
uq |
max |
1 |
colQuantiles |
1.000000 |
1.000000 |
1.000000 |
1.000000 |
1.000000 |
1.000000 |
2 |
rowQuantiles |
1.069938 |
1.057863 |
1.060016 |
1.048339 |
1.044924 |
1.064464 |
Figure: Benchmarking of colQuantiles() and rowQuantiles() on 1000x10 data (original and transposed). Outliers are displayed as crosses. Times are in milliseconds.
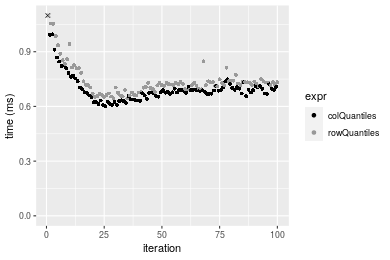
10x1000 matrix
> X <- data[["10x1000"]]
> gc()
used (Mb) gc trigger (Mb) max used (Mb)
Ncells 5271934 281.6 8529671 455.6 8529671 455.6
Vcells 10107214 77.2 31876688 243.2 60562128 462.1
> probs <- seq(from = 0, to = 1, by = 0.25)
> colStats <- microbenchmark(colQuantiles = colQuantiles(X, probs = probs, na.rm = FALSE), `apply+quantile` = apply(X,
+ MARGIN = 2L, FUN = quantile, probs = probs, na.rm = FALSE), unit = "ms")
> X <- t(X)
> gc()
used (Mb) gc trigger (Mb) max used (Mb)
Ncells 5271922 281.6 8529671 455.6 8529671 455.6
Vcells 10117247 77.2 31876688 243.2 60562128 462.1
> rowStats <- microbenchmark(rowQuantiles = rowQuantiles(X, probs = probs, na.rm = FALSE), `apply+quantile` = apply(X,
+ MARGIN = 1L, FUN = quantile, probs = probs, na.rm = FALSE), unit = "ms")
Table: Benchmarking of colQuantiles() and apply+quantile() on 10x1000 data. The top panel shows times in milliseconds and the bottom panel shows relative times.
|
expr |
min |
lq |
mean |
median |
uq |
max |
1 |
colQuantiles |
14.45429 |
15.83936 |
16.89861 |
16.25864 |
16.82524 |
46.84812 |
2 |
apply+quantile |
112.81877 |
116.21819 |
123.35627 |
119.25667 |
127.25195 |
161.59093 |
|
expr |
min |
lq |
mean |
median |
uq |
max |
1 |
colQuantiles |
1.000000 |
1.000000 |
1.000000 |
1.000000 |
1.000000 |
1.000000 |
2 |
apply+quantile |
7.805208 |
7.337304 |
7.299786 |
7.334974 |
7.563158 |
3.449251 |
Table: Benchmarking of rowQuantiles() and apply+quantile() on 10x1000 data (transposed). The top panel shows times in milliseconds and the bottom panel shows relative times.
|
expr |
min |
lq |
mean |
median |
uq |
max |
1 |
rowQuantiles |
14.45924 |
15.9970 |
20.28669 |
16.20631 |
16.56712 |
381.9050 |
2 |
apply+quantile |
110.53220 |
118.0066 |
121.22556 |
119.13909 |
125.02020 |
144.3018 |
|
expr |
min |
lq |
mean |
median |
uq |
max |
1 |
rowQuantiles |
1.000000 |
1.000000 |
1.00000 |
1.000000 |
1.000000 |
1.0000000 |
2 |
apply+quantile |
7.644397 |
7.376794 |
5.97562 |
7.351401 |
7.546282 |
0.3778473 |
Figure: Benchmarking of colQuantiles() and apply+quantile() on 10x1000 data as well as rowQuantiles() and apply+quantile() on the same data transposed. Outliers are displayed as crosses. Times are in milliseconds.
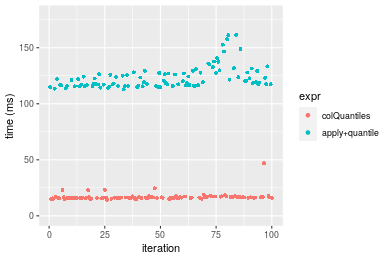
Table: Benchmarking of colQuantiles() and rowQuantiles() on 10x1000 data (original and transposed). The top panel shows times in milliseconds and the bottom panel shows relative times.
|
expr |
min |
lq |
mean |
median |
uq |
max |
2 |
rowQuantiles |
14.45924 |
15.99700 |
20.28669 |
16.20631 |
16.56712 |
381.90503 |
1 |
colQuantiles |
14.45429 |
15.83936 |
16.89861 |
16.25864 |
16.82524 |
46.84812 |
|
expr |
min |
lq |
mean |
median |
uq |
max |
2 |
rowQuantiles |
1.0000000 |
1.0000000 |
1.0000000 |
1.000000 |
1.00000 |
1.0000000 |
1 |
colQuantiles |
0.9996576 |
0.9901455 |
0.8329901 |
1.003229 |
1.01558 |
0.1226696 |
Figure: Benchmarking of colQuantiles() and rowQuantiles() on 10x1000 data (original and transposed). Outliers are displayed as crosses. Times are in milliseconds.
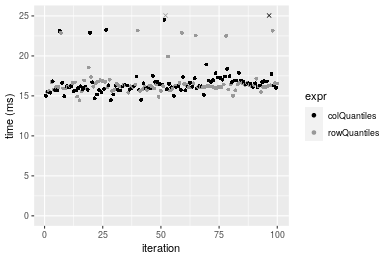
100x1000 matrix
> X <- data[["100x1000"]]
> gc()
used (Mb) gc trigger (Mb) max used (Mb)
Ncells 5272118 281.6 8529671 455.6 8529671 455.6
Vcells 10107704 77.2 31876688 243.2 60562128 462.1
> probs <- seq(from = 0, to = 1, by = 0.25)
> colStats <- microbenchmark(colQuantiles = colQuantiles(X, probs = probs, na.rm = FALSE), `apply+quantile` = apply(X,
+ MARGIN = 2L, FUN = quantile, probs = probs, na.rm = FALSE), unit = "ms")
> X <- t(X)
> gc()
used (Mb) gc trigger (Mb) max used (Mb)
Ncells 5272106 281.6 8529671 455.6 8529671 455.6
Vcells 10207737 77.9 31876688 243.2 60562128 462.1
> rowStats <- microbenchmark(rowQuantiles = rowQuantiles(X, probs = probs, na.rm = FALSE), `apply+quantile` = apply(X,
+ MARGIN = 1L, FUN = quantile, probs = probs, na.rm = FALSE), unit = "ms")
Table: Benchmarking of colQuantiles() and apply+quantile() on 100x1000 data. The top panel shows times in milliseconds and the bottom panel shows relative times.
|
expr |
min |
lq |
mean |
median |
uq |
max |
1 |
colQuantiles |
19.78046 |
21.08175 |
21.71354 |
21.32056 |
21.61416 |
32.44358 |
2 |
apply+quantile |
124.22724 |
127.17620 |
131.48661 |
128.56766 |
136.34522 |
150.63818 |
|
expr |
min |
lq |
mean |
median |
uq |
max |
1 |
colQuantiles |
1.000000 |
1.000000 |
1.000000 |
1.000000 |
1.000000 |
1.000000 |
2 |
apply+quantile |
6.280301 |
6.032525 |
6.055512 |
6.030219 |
6.308144 |
4.643082 |
Table: Benchmarking of rowQuantiles() and apply+quantile() on 100x1000 data (transposed). The top panel shows times in milliseconds and the bottom panel shows relative times.
|
expr |
min |
lq |
mean |
median |
uq |
max |
1 |
rowQuantiles |
20.02987 |
21.71925 |
22.67224 |
21.97428 |
22.16405 |
44.66161 |
2 |
apply+quantile |
123.55330 |
127.41662 |
131.43956 |
128.76763 |
136.82947 |
146.98970 |
|
expr |
min |
lq |
mean |
median |
uq |
max |
1 |
rowQuantiles |
1.000000 |
1.000000 |
1.000000 |
1.000000 |
1.000000 |
1.000000 |
2 |
apply+quantile |
6.168454 |
5.866531 |
5.797378 |
5.859925 |
6.173487 |
3.291187 |
Figure: Benchmarking of colQuantiles() and apply+quantile() on 100x1000 data as well as rowQuantiles() and apply+quantile() on the same data transposed. Outliers are displayed as crosses. Times are in milliseconds.
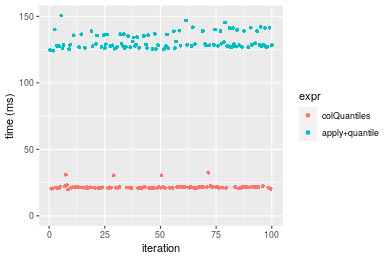
Table: Benchmarking of colQuantiles() and rowQuantiles() on 100x1000 data (original and transposed). The top panel shows times in milliseconds and the bottom panel shows relative times.
|
expr |
min |
lq |
mean |
median |
uq |
max |
1 |
colQuantiles |
19.78046 |
21.08175 |
21.71354 |
21.32056 |
21.61416 |
32.44358 |
2 |
rowQuantiles |
20.02987 |
21.71925 |
22.67224 |
21.97428 |
22.16405 |
44.66161 |
|
expr |
min |
lq |
mean |
median |
uq |
max |
1 |
colQuantiles |
1.000000 |
1.000000 |
1.000000 |
1.000000 |
1.000000 |
1.000000 |
2 |
rowQuantiles |
1.012609 |
1.030239 |
1.044152 |
1.030661 |
1.025441 |
1.376593 |
Figure: Benchmarking of colQuantiles() and rowQuantiles() on 100x1000 data (original and transposed). Outliers are displayed as crosses. Times are in milliseconds.
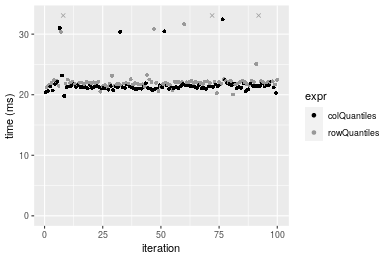
1000x100 matrix
> X <- data[["1000x100"]]
> gc()
used (Mb) gc trigger (Mb) max used (Mb)
Ncells 5272310 281.6 8529671 455.6 8529671 455.6
Vcells 10108335 77.2 31876688 243.2 60562128 462.1
> probs <- seq(from = 0, to = 1, by = 0.25)
> colStats <- microbenchmark(colQuantiles = colQuantiles(X, probs = probs, na.rm = FALSE), `apply+quantile` = apply(X,
+ MARGIN = 2L, FUN = quantile, probs = probs, na.rm = FALSE), unit = "ms")
> X <- t(X)
> gc()
used (Mb) gc trigger (Mb) max used (Mb)
Ncells 5272298 281.6 8529671 455.6 8529671 455.6
Vcells 10208368 77.9 31876688 243.2 60562128 462.1
> rowStats <- microbenchmark(rowQuantiles = rowQuantiles(X, probs = probs, na.rm = FALSE), `apply+quantile` = apply(X,
+ MARGIN = 1L, FUN = quantile, probs = probs, na.rm = FALSE), unit = "ms")
Table: Benchmarking of colQuantiles() and apply+quantile() on 1000x100 data. The top panel shows times in milliseconds and the bottom panel shows relative times.
|
expr |
min |
lq |
mean |
median |
uq |
max |
1 |
colQuantiles |
5.636397 |
6.027108 |
6.135147 |
6.116474 |
6.188172 |
8.237741 |
2 |
apply+quantile |
15.165356 |
17.037116 |
17.544969 |
17.240510 |
17.492228 |
28.635703 |
|
expr |
min |
lq |
mean |
median |
uq |
max |
1 |
colQuantiles |
1.000000 |
1.000000 |
1.000000 |
1.000000 |
1.00000 |
1.00000 |
2 |
apply+quantile |
2.690612 |
2.826748 |
2.859747 |
2.818701 |
2.82672 |
3.47616 |
Table: Benchmarking of rowQuantiles() and apply+quantile() on 1000x100 data (transposed). The top panel shows times in milliseconds and the bottom panel shows relative times.
|
expr |
min |
lq |
mean |
median |
uq |
max |
1 |
rowQuantiles |
5.665498 |
6.479869 |
6.509218 |
6.53708 |
6.612499 |
7.358592 |
2 |
apply+quantile |
15.444699 |
17.088696 |
17.574659 |
17.28185 |
17.448529 |
28.096502 |
|
expr |
min |
lq |
mean |
median |
uq |
max |
1 |
rowQuantiles |
1.000000 |
1.000000 |
1.000000 |
1.000000 |
1.00000 |
1.00000 |
2 |
apply+quantile |
2.726097 |
2.637198 |
2.699965 |
2.643664 |
2.63872 |
3.81819 |
Figure: Benchmarking of colQuantiles() and apply+quantile() on 1000x100 data as well as rowQuantiles() and apply+quantile() on the same data transposed. Outliers are displayed as crosses. Times are in milliseconds.
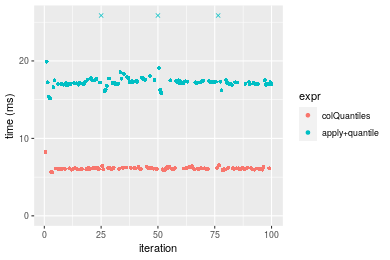
Table: Benchmarking of colQuantiles() and rowQuantiles() on 1000x100 data (original and transposed). The top panel shows times in milliseconds and the bottom panel shows relative times.
|
expr |
min |
lq |
mean |
median |
uq |
max |
1 |
colQuantiles |
5.636397 |
6.027108 |
6.135147 |
6.116474 |
6.188172 |
8.237741 |
2 |
rowQuantiles |
5.665498 |
6.479869 |
6.509218 |
6.537080 |
6.612499 |
7.358592 |
|
expr |
min |
lq |
mean |
median |
uq |
max |
1 |
colQuantiles |
1.000000 |
1.000000 |
1.000000 |
1.000000 |
1.000000 |
1.0000000 |
2 |
rowQuantiles |
1.005163 |
1.075121 |
1.060972 |
1.068766 |
1.068571 |
0.8932779 |
Figure: Benchmarking of colQuantiles() and rowQuantiles() on 1000x100 data (original and transposed). Outliers are displayed as crosses. Times are in milliseconds.
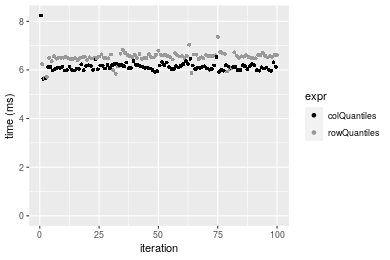
Appendix
R version 4.1.1 Patched (2021-08-10 r80727)
Platform: x86_64-pc-linux-gnu (64-bit)
Running under: Ubuntu 18.04.5 LTS
Matrix products: default
BLAS: /home/hb/software/R-devel/R-4-1-branch/lib/R/lib/libRblas.so
LAPACK: /home/hb/software/R-devel/R-4-1-branch/lib/R/lib/libRlapack.so
locale:
[1] LC_CTYPE=en_US.UTF-8 LC_NUMERIC=C
[3] LC_TIME=en_US.UTF-8 LC_COLLATE=en_US.UTF-8
[5] LC_MONETARY=en_US.UTF-8 LC_MESSAGES=en_US.UTF-8
[7] LC_PAPER=en_US.UTF-8 LC_NAME=C
[9] LC_ADDRESS=C LC_TELEPHONE=C
[11] LC_MEASUREMENT=en_US.UTF-8 LC_IDENTIFICATION=C
attached base packages:
[1] stats graphics grDevices utils datasets methods base
other attached packages:
[1] microbenchmark_1.4-7 matrixStats_0.60.1 ggplot2_3.3.5
[4] knitr_1.33 R.devices_2.17.0 R.utils_2.10.1
[7] R.oo_1.24.0 R.methodsS3_1.8.1-9001 history_0.0.1-9000
loaded via a namespace (and not attached):
[1] Biobase_2.52.0 httr_1.4.2 splines_4.1.1
[4] bit64_4.0.5 network_1.17.1 assertthat_0.2.1
[7] highr_0.9 stats4_4.1.1 blob_1.2.2
[10] GenomeInfoDbData_1.2.6 robustbase_0.93-8 pillar_1.6.2
[13] RSQLite_2.2.8 lattice_0.20-44 glue_1.4.2
[16] digest_0.6.27 XVector_0.32.0 colorspace_2.0-2
[19] Matrix_1.3-4 XML_3.99-0.7 pkgconfig_2.0.3
[22] zlibbioc_1.38.0 genefilter_1.74.0 purrr_0.3.4
[25] ergm_4.1.2 xtable_1.8-4 scales_1.1.1
[28] tibble_3.1.4 annotate_1.70.0 KEGGREST_1.32.0
[31] farver_2.1.0 generics_0.1.0 IRanges_2.26.0
[34] ellipsis_0.3.2 cachem_1.0.6 withr_2.4.2
[37] BiocGenerics_0.38.0 mime_0.11 survival_3.2-13
[40] magrittr_2.0.1 crayon_1.4.1 statnet.common_4.5.0
[43] memoise_2.0.0 laeken_0.5.1 fansi_0.5.0
[46] R.cache_0.15.0 MASS_7.3-54 R.rsp_0.44.0
[49] progressr_0.8.0 tools_4.1.1 lifecycle_1.0.0
[52] S4Vectors_0.30.0 trust_0.1-8 munsell_0.5.0
[55] tabby_0.0.1-9001 AnnotationDbi_1.54.1 Biostrings_2.60.2
[58] compiler_4.1.1 GenomeInfoDb_1.28.1 rlang_0.4.11
[61] grid_4.1.1 RCurl_1.98-1.4 cwhmisc_6.6
[64] rappdirs_0.3.3 startup_0.15.0 labeling_0.4.2
[67] bitops_1.0-7 base64enc_0.1-3 boot_1.3-28
[70] gtable_0.3.0 DBI_1.1.1 markdown_1.1
[73] R6_2.5.1 lpSolveAPI_5.5.2.0-17.7 rle_0.9.2
[76] dplyr_1.0.7 fastmap_1.1.0 bit_4.0.4
[79] utf8_1.2.2 parallel_4.1.1 Rcpp_1.0.7
[82] vctrs_0.3.8 png_0.1-7 DEoptimR_1.0-9
[85] tidyselect_1.1.1 xfun_0.25 coda_0.19-4
Total processing time was 1.32 mins.
Reproducibility
To reproduce this report, do:
html <- matrixStats:::benchmark('colQuantiles')
Copyright Henrik Bengtsson. Last updated on 2021-08-25 19:06:31 (+0200 UTC). Powered by RSP.