colLogSumExps() and rowLogSumExps() benchmarks
This report benchmark the performance of colLogSumExps() and rowLogSumExps() against alternative methods.
Alternative methods
- apply() + matrixStats::logSumExp()
- apply() + logSumExp0()
where
> logSumExp0 <- function(lx, ...) {
+ iMax <- which.max(lx)
+ log1p(sum(exp(lx[-iMax] - lx[iMax]))) + lx[iMax]
+ }
Data
> rmatrix <- function(nrow, ncol, mode = c("logical", "double", "integer", "index"), range = c(-100,
+ +100), na_prob = 0) {
+ mode <- match.arg(mode)
+ n <- nrow * ncol
+ if (mode == "logical") {
+ x <- sample(c(FALSE, TRUE), size = n, replace = TRUE)
+ } else if (mode == "index") {
+ x <- seq_len(n)
+ mode <- "integer"
+ } else {
+ x <- runif(n, min = range[1], max = range[2])
+ }
+ storage.mode(x) <- mode
+ if (na_prob > 0)
+ x[sample(n, size = na_prob * n)] <- NA
+ dim(x) <- c(nrow, ncol)
+ x
+ }
> rmatrices <- function(scale = 10, seed = 1, ...) {
+ set.seed(seed)
+ data <- list()
+ data[[1]] <- rmatrix(nrow = scale * 1, ncol = scale * 1, ...)
+ data[[2]] <- rmatrix(nrow = scale * 10, ncol = scale * 10, ...)
+ data[[3]] <- rmatrix(nrow = scale * 100, ncol = scale * 1, ...)
+ data[[4]] <- t(data[[3]])
+ data[[5]] <- rmatrix(nrow = scale * 10, ncol = scale * 100, ...)
+ data[[6]] <- t(data[[5]])
+ names(data) <- sapply(data, FUN = function(x) paste(dim(x), collapse = "x"))
+ data
+ }
> data <- rmatrices(mode = "double")
Results
10x10 matrix
> X <- data[["10x10"]]
> gc()
used (Mb) gc trigger (Mb) max used (Mb)
Ncells 5228971 279.3 8529671 455.6 8529671 455.6
Vcells 10151080 77.5 31876688 243.2 60562128 462.1
> colStats <- microbenchmark(colLogSumExps = colLogSumExps(X, na.rm = FALSE), `apply+logSumExp` = apply(X,
+ MARGIN = 2L, FUN = logSumExp, na.rm = FALSE), `apply+logSumExp0` = apply(X, MARGIN = 2L, FUN = logSumExp0,
+ na.rm = FALSE), unit = "ms")
> X <- t(X)
> gc()
used (Mb) gc trigger (Mb) max used (Mb)
Ncells 5219380 278.8 8529671 455.6 8529671 455.6
Vcells 10119728 77.3 31876688 243.2 60562128 462.1
> rowStats <- microbenchmark(rowLogSumExps = rowLogSumExps(X, na.rm = FALSE), `apply+logSumExp` = apply(X,
+ MARGIN = 1L, FUN = logSumExp, na.rm = FALSE), `apply+logSumExp0` = apply(X, MARGIN = 1L, FUN = logSumExp0,
+ na.rm = FALSE), unit = "ms")
Table: Benchmarking of colLogSumExps(), apply+logSumExp() and apply+logSumExp0() on 10x10 data. The top panel shows times in milliseconds and the bottom panel shows relative times.
|
expr |
min |
lq |
mean |
median |
uq |
max |
1 |
colLogSumExps |
0.004115 |
0.0047255 |
0.0053071 |
0.0051590 |
0.0055450 |
0.018341 |
2 |
apply+logSumExp |
0.043589 |
0.0460690 |
0.0493210 |
0.0475195 |
0.0500855 |
0.142218 |
3 |
apply+logSumExp0 |
0.061030 |
0.0631095 |
0.0672718 |
0.0643335 |
0.0690305 |
0.166412 |
|
expr |
min |
lq |
mean |
median |
uq |
max |
1 |
colLogSumExps |
1.00000 |
1.000000 |
1.00000 |
1.00000 |
1.000000 |
1.000000 |
2 |
apply+logSumExp |
10.59271 |
9.749021 |
9.29344 |
9.21099 |
9.032552 |
7.754103 |
3 |
apply+logSumExp0 |
14.83111 |
13.355095 |
12.67586 |
12.47015 |
12.449143 |
9.073224 |
Table: Benchmarking of rowLogSumExps(), apply+logSumExp() and apply+logSumExp0() on 10x10 data (transposed). The top panel shows times in milliseconds and the bottom panel shows relative times.
|
expr |
min |
lq |
mean |
median |
uq |
max |
1 |
rowLogSumExps |
0.004288 |
0.0047920 |
0.0054875 |
0.0053690 |
0.0057515 |
0.018216 |
2 |
apply+logSumExp |
0.043553 |
0.0463765 |
0.0488194 |
0.0480420 |
0.0497345 |
0.104476 |
3 |
apply+logSumExp0 |
0.058941 |
0.0627360 |
0.0676865 |
0.0657705 |
0.0689620 |
0.156978 |
|
expr |
min |
lq |
mean |
median |
uq |
max |
1 |
rowLogSumExps |
1.00000 |
1.000000 |
1.000000 |
1.000000 |
1.000000 |
1.000000 |
2 |
apply+logSumExp |
10.15695 |
9.677901 |
8.896548 |
8.948035 |
8.647222 |
5.735398 |
3 |
apply+logSumExp0 |
13.74557 |
13.091820 |
12.334767 |
12.250047 |
11.990263 |
8.617589 |
Figure: Benchmarking of colLogSumExps(), apply+logSumExp() and apply+logSumExp0() on 10x10 data as well as rowLogSumExps(), apply+logSumExp() and apply+logSumExp0() on the same data transposed. Outliers are displayed as crosses. Times are in milliseconds.
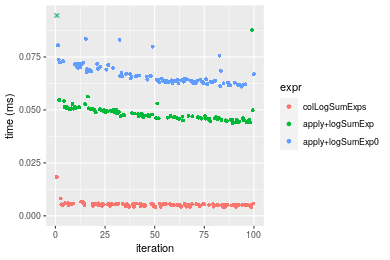
Table: Benchmarking of colLogSumExps() and rowLogSumExps() on 10x10 data (original and transposed). The top panel shows times in milliseconds and the bottom panel shows relative times.
|
expr |
min |
lq |
mean |
median |
uq |
max |
1 |
colLogSumExps |
4.115 |
4.7255 |
5.30708 |
5.159 |
5.5450 |
18.341 |
2 |
rowLogSumExps |
4.288 |
4.7920 |
5.48746 |
5.369 |
5.7515 |
18.216 |
|
expr |
min |
lq |
mean |
median |
uq |
max |
1 |
colLogSumExps |
1.000000 |
1.000000 |
1.000000 |
1.000000 |
1.000000 |
1.0000000 |
2 |
rowLogSumExps |
1.042041 |
1.014073 |
1.033989 |
1.040706 |
1.037241 |
0.9931847 |
Figure: Benchmarking of colLogSumExps() and rowLogSumExps() on 10x10 data (original and transposed). Outliers are displayed as crosses. Times are in milliseconds.
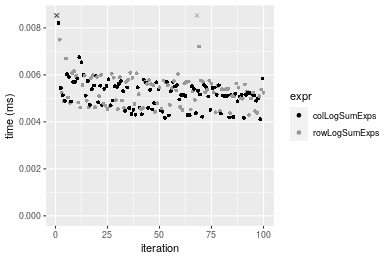
100x100 matrix
> X <- data[["100x100"]]
> gc()
used (Mb) gc trigger (Mb) max used (Mb)
Ncells 5217958 278.7 8529671 455.6 8529671 455.6
Vcells 9736343 74.3 31876688 243.2 60562128 462.1
> colStats <- microbenchmark(colLogSumExps = colLogSumExps(X, na.rm = FALSE), `apply+logSumExp` = apply(X,
+ MARGIN = 2L, FUN = logSumExp, na.rm = FALSE), `apply+logSumExp0` = apply(X, MARGIN = 2L, FUN = logSumExp0,
+ na.rm = FALSE), unit = "ms")
> X <- t(X)
> gc()
used (Mb) gc trigger (Mb) max used (Mb)
Ncells 5217934 278.7 8529671 455.6 8529671 455.6
Vcells 9746356 74.4 31876688 243.2 60562128 462.1
> rowStats <- microbenchmark(rowLogSumExps = rowLogSumExps(X, na.rm = FALSE), `apply+logSumExp` = apply(X,
+ MARGIN = 1L, FUN = logSumExp, na.rm = FALSE), `apply+logSumExp0` = apply(X, MARGIN = 1L, FUN = logSumExp0,
+ na.rm = FALSE), unit = "ms")
Table: Benchmarking of colLogSumExps(), apply+logSumExp() and apply+logSumExp0() on 100x100 data. The top panel shows times in milliseconds and the bottom panel shows relative times.
|
expr |
min |
lq |
mean |
median |
uq |
max |
1 |
colLogSumExps |
0.157921 |
0.1673080 |
0.1806528 |
0.1727235 |
0.1869930 |
0.289930 |
2 |
apply+logSumExp |
0.382036 |
0.3999075 |
0.4347645 |
0.4173075 |
0.4505250 |
0.644744 |
3 |
apply+logSumExp0 |
0.543190 |
0.5626970 |
0.6222792 |
0.5895445 |
0.6450365 |
1.022007 |
|
expr |
min |
lq |
mean |
median |
uq |
max |
1 |
colLogSumExps |
1.000000 |
1.000000 |
1.000000 |
1.000000 |
1.000000 |
1.000000 |
2 |
apply+logSumExp |
2.419159 |
2.390247 |
2.406630 |
2.416044 |
2.409315 |
2.223792 |
3 |
apply+logSumExp0 |
3.439631 |
3.363240 |
3.444615 |
3.413227 |
3.449522 |
3.525013 |
Table: Benchmarking of rowLogSumExps(), apply+logSumExp() and apply+logSumExp0() on 100x100 data (transposed). The top panel shows times in milliseconds and the bottom panel shows relative times.
|
expr |
min |
lq |
mean |
median |
uq |
max |
1 |
rowLogSumExps |
0.165548 |
0.1703945 |
0.1902944 |
0.1811930 |
0.1951185 |
0.294609 |
2 |
apply+logSumExp |
0.380418 |
0.4002895 |
0.4395488 |
0.4142425 |
0.4495775 |
0.687410 |
3 |
apply+logSumExp0 |
0.542032 |
0.5688875 |
0.6197588 |
0.5908095 |
0.6360910 |
1.152939 |
|
expr |
min |
lq |
mean |
median |
uq |
max |
1 |
rowLogSumExps |
1.000000 |
1.000000 |
1.000000 |
1.000000 |
1.000000 |
1.000000 |
2 |
apply+logSumExp |
2.297932 |
2.349193 |
2.309836 |
2.286195 |
2.304125 |
2.333296 |
3 |
apply+logSumExp0 |
3.274168 |
3.338649 |
3.256842 |
3.260664 |
3.260024 |
3.913455 |
Figure: Benchmarking of colLogSumExps(), apply+logSumExp() and apply+logSumExp0() on 100x100 data as well as rowLogSumExps(), apply+logSumExp() and apply+logSumExp0() on the same data transposed. Outliers are displayed as crosses. Times are in milliseconds.
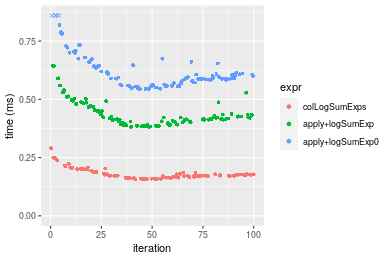
Table: Benchmarking of colLogSumExps() and rowLogSumExps() on 100x100 data (original and transposed). The top panel shows times in milliseconds and the bottom panel shows relative times.
|
expr |
min |
lq |
mean |
median |
uq |
max |
1 |
colLogSumExps |
157.921 |
167.3080 |
180.6528 |
172.7235 |
186.9930 |
289.930 |
2 |
rowLogSumExps |
165.548 |
170.3945 |
190.2944 |
181.1930 |
195.1185 |
294.609 |
|
expr |
min |
lq |
mean |
median |
uq |
max |
1 |
colLogSumExps |
1.000000 |
1.000000 |
1.000000 |
1.000000 |
1.000000 |
1.000000 |
2 |
rowLogSumExps |
1.048296 |
1.018448 |
1.053371 |
1.049035 |
1.043454 |
1.016138 |
Figure: Benchmarking of colLogSumExps() and rowLogSumExps() on 100x100 data (original and transposed). Outliers are displayed as crosses. Times are in milliseconds.
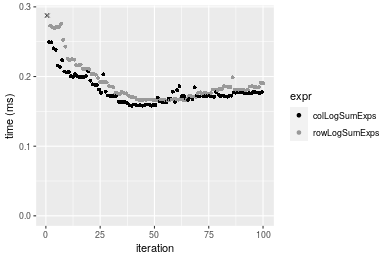
1000x10 matrix
> X <- data[["1000x10"]]
> gc()
used (Mb) gc trigger (Mb) max used (Mb)
Ncells 5218689 278.8 8529671 455.6 8529671 455.6
Vcells 9740091 74.4 31876688 243.2 60562128 462.1
> colStats <- microbenchmark(colLogSumExps = colLogSumExps(X, na.rm = FALSE), `apply+logSumExp` = apply(X,
+ MARGIN = 2L, FUN = logSumExp, na.rm = FALSE), `apply+logSumExp0` = apply(X, MARGIN = 2L, FUN = logSumExp0,
+ na.rm = FALSE), unit = "ms")
> X <- t(X)
> gc()
used (Mb) gc trigger (Mb) max used (Mb)
Ncells 5218683 278.8 8529671 455.6 8529671 455.6
Vcells 9750134 74.4 31876688 243.2 60562128 462.1
> rowStats <- microbenchmark(rowLogSumExps = rowLogSumExps(X, na.rm = FALSE), `apply+logSumExp` = apply(X,
+ MARGIN = 1L, FUN = logSumExp, na.rm = FALSE), `apply+logSumExp0` = apply(X, MARGIN = 1L, FUN = logSumExp0,
+ na.rm = FALSE), unit = "ms")
Table: Benchmarking of colLogSumExps(), apply+logSumExp() and apply+logSumExp0() on 1000x10 data. The top panel shows times in milliseconds and the bottom panel shows relative times.
|
expr |
min |
lq |
mean |
median |
uq |
max |
1 |
colLogSumExps |
0.154186 |
0.158505 |
0.1814515 |
0.172183 |
0.2007125 |
0.265225 |
2 |
apply+logSumExp |
0.228059 |
0.233735 |
0.2634239 |
0.247757 |
0.2804950 |
0.469100 |
3 |
apply+logSumExp0 |
0.270533 |
0.277889 |
0.3147685 |
0.298207 |
0.3446450 |
0.448955 |
|
expr |
min |
lq |
mean |
median |
uq |
max |
1 |
colLogSumExps |
1.000000 |
1.000000 |
1.000000 |
1.000000 |
1.000000 |
1.000000 |
2 |
apply+logSumExp |
1.479116 |
1.474622 |
1.451759 |
1.438917 |
1.397496 |
1.768687 |
3 |
apply+logSumExp0 |
1.754589 |
1.753188 |
1.734725 |
1.731919 |
1.717108 |
1.692733 |
Table: Benchmarking of rowLogSumExps(), apply+logSumExp() and apply+logSumExp0() on 1000x10 data (transposed). The top panel shows times in milliseconds and the bottom panel shows relative times.
|
expr |
min |
lq |
mean |
median |
uq |
max |
1 |
rowLogSumExps |
0.161690 |
0.1670955 |
0.1943217 |
0.1870175 |
0.2125375 |
0.270339 |
2 |
apply+logSumExp |
0.228044 |
0.2328070 |
0.2652763 |
0.2485140 |
0.2892600 |
0.459792 |
3 |
apply+logSumExp0 |
0.271421 |
0.2770635 |
0.3082615 |
0.2944350 |
0.3266250 |
0.434325 |
|
expr |
min |
lq |
mean |
median |
uq |
max |
1 |
rowLogSumExps |
1.000000 |
1.000000 |
1.000000 |
1.000000 |
1.000000 |
1.000000 |
2 |
apply+logSumExp |
1.410378 |
1.393257 |
1.365140 |
1.328828 |
1.360983 |
1.700798 |
3 |
apply+logSumExp0 |
1.678651 |
1.658115 |
1.586346 |
1.574371 |
1.536788 |
1.606594 |
Figure: Benchmarking of colLogSumExps(), apply+logSumExp() and apply+logSumExp0() on 1000x10 data as well as rowLogSumExps(), apply+logSumExp() and apply+logSumExp0() on the same data transposed. Outliers are displayed as crosses. Times are in milliseconds.
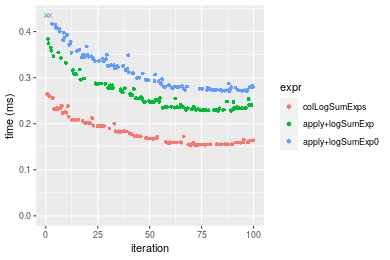
Table: Benchmarking of colLogSumExps() and rowLogSumExps() on 1000x10 data (original and transposed). The top panel shows times in milliseconds and the bottom panel shows relative times.
|
expr |
min |
lq |
mean |
median |
uq |
max |
1 |
colLogSumExps |
154.186 |
158.5050 |
181.4515 |
172.1830 |
200.7125 |
265.225 |
2 |
rowLogSumExps |
161.690 |
167.0955 |
194.3217 |
187.0175 |
212.5375 |
270.339 |
|
expr |
min |
lq |
mean |
median |
uq |
max |
1 |
colLogSumExps |
1.000000 |
1.000000 |
1.000000 |
1.000000 |
1.000000 |
1.000000 |
2 |
rowLogSumExps |
1.048669 |
1.054197 |
1.070929 |
1.086155 |
1.058915 |
1.019282 |
Figure: Benchmarking of colLogSumExps() and rowLogSumExps() on 1000x10 data (original and transposed). Outliers are displayed as crosses. Times are in milliseconds.
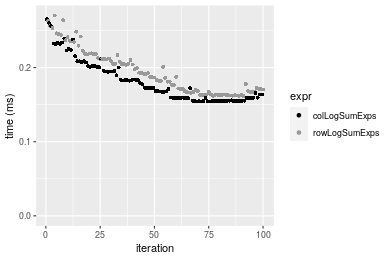
10x1000 matrix
> X <- data[["10x1000"]]
> gc()
used (Mb) gc trigger (Mb) max used (Mb)
Ncells 5218920 278.8 8529671 455.6 8529671 455.6
Vcells 9740933 74.4 31876688 243.2 60562128 462.1
> colStats <- microbenchmark(colLogSumExps = colLogSumExps(X, na.rm = FALSE), `apply+logSumExp` = apply(X,
+ MARGIN = 2L, FUN = logSumExp, na.rm = FALSE), `apply+logSumExp0` = apply(X, MARGIN = 2L, FUN = logSumExp0,
+ na.rm = FALSE), unit = "ms")
> X <- t(X)
> gc()
used (Mb) gc trigger (Mb) max used (Mb)
Ncells 5218896 278.8 8529671 455.6 8529671 455.6
Vcells 9750946 74.4 31876688 243.2 60562128 462.1
> rowStats <- microbenchmark(rowLogSumExps = rowLogSumExps(X, na.rm = FALSE), `apply+logSumExp` = apply(X,
+ MARGIN = 1L, FUN = logSumExp, na.rm = FALSE), `apply+logSumExp0` = apply(X, MARGIN = 1L, FUN = logSumExp0,
+ na.rm = FALSE), unit = "ms")
Table: Benchmarking of colLogSumExps(), apply+logSumExp() and apply+logSumExp0() on 10x1000 data. The top panel shows times in milliseconds and the bottom panel shows relative times.
|
expr |
min |
lq |
mean |
median |
uq |
max |
1 |
colLogSumExps |
0.190061 |
0.211368 |
0.2199596 |
0.217669 |
0.2249845 |
0.283629 |
2 |
apply+logSumExp |
1.764354 |
1.991289 |
2.1139157 |
2.046578 |
2.1101505 |
6.844011 |
3 |
apply+logSumExp0 |
2.993370 |
3.385412 |
3.5302578 |
3.515979 |
3.6096990 |
8.362414 |
|
expr |
min |
lq |
mean |
median |
uq |
max |
1 |
colLogSumExps |
1.000000 |
1.000000 |
1.000000 |
1.000000 |
1.000000 |
1.00000 |
2 |
apply+logSumExp |
9.283093 |
9.420955 |
9.610475 |
9.402248 |
9.379093 |
24.13015 |
3 |
apply+logSumExp0 |
15.749522 |
16.016670 |
16.049577 |
16.152867 |
16.044212 |
29.48364 |
Table: Benchmarking of rowLogSumExps(), apply+logSumExp() and apply+logSumExp0() on 10x1000 data (transposed). The top panel shows times in milliseconds and the bottom panel shows relative times.
|
expr |
min |
lq |
mean |
median |
uq |
max |
1 |
rowLogSumExps |
0.194062 |
0.2169925 |
0.2291399 |
0.224315 |
0.231641 |
0.335443 |
2 |
apply+logSumExp |
1.777240 |
1.9722690 |
2.0639333 |
2.024551 |
2.115266 |
3.554903 |
3 |
apply+logSumExp0 |
3.024545 |
3.3911160 |
3.5965822 |
3.481692 |
3.643680 |
8.478938 |
|
expr |
min |
lq |
mean |
median |
uq |
max |
1 |
rowLogSumExps |
1.000000 |
1.000000 |
1.000000 |
1.000000 |
1.000000 |
1.00000 |
2 |
apply+logSumExp |
9.158104 |
9.089111 |
9.007306 |
9.025482 |
9.131656 |
10.59764 |
3 |
apply+logSumExp0 |
15.585457 |
15.627803 |
15.696009 |
15.521443 |
15.729856 |
25.27684 |
Figure: Benchmarking of colLogSumExps(), apply+logSumExp() and apply+logSumExp0() on 10x1000 data as well as rowLogSumExps(), apply+logSumExp() and apply+logSumExp0() on the same data transposed. Outliers are displayed as crosses. Times are in milliseconds.
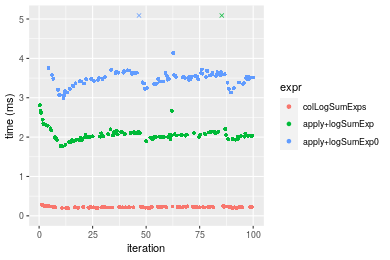
Table: Benchmarking of colLogSumExps() and rowLogSumExps() on 10x1000 data (original and transposed). The top panel shows times in milliseconds and the bottom panel shows relative times.
|
expr |
min |
lq |
mean |
median |
uq |
max |
1 |
colLogSumExps |
190.061 |
211.3680 |
219.9596 |
217.669 |
224.9845 |
283.629 |
2 |
rowLogSumExps |
194.062 |
216.9925 |
229.1399 |
224.315 |
231.6410 |
335.443 |
|
expr |
min |
lq |
mean |
median |
uq |
max |
1 |
colLogSumExps |
1.000000 |
1.00000 |
1.000000 |
1.000000 |
1.000000 |
1.000000 |
2 |
rowLogSumExps |
1.021051 |
1.02661 |
1.041737 |
1.030533 |
1.029586 |
1.182682 |
Figure: Benchmarking of colLogSumExps() and rowLogSumExps() on 10x1000 data (original and transposed). Outliers are displayed as crosses. Times are in milliseconds.
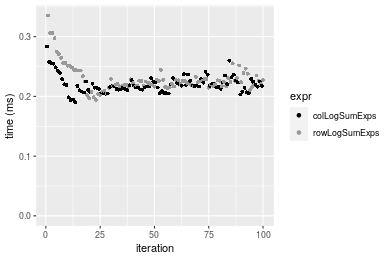
100x1000 matrix
> X <- data[["100x1000"]]
> gc()
used (Mb) gc trigger (Mb) max used (Mb)
Ncells 5219106 278.8 8529671 455.6 8529671 455.6
Vcells 9741475 74.4 31876688 243.2 60562128 462.1
> colStats <- microbenchmark(colLogSumExps = colLogSumExps(X, na.rm = FALSE), `apply+logSumExp` = apply(X,
+ MARGIN = 2L, FUN = logSumExp, na.rm = FALSE), `apply+logSumExp0` = apply(X, MARGIN = 2L, FUN = logSumExp0,
+ na.rm = FALSE), unit = "ms")
> X <- t(X)
> gc()
used (Mb) gc trigger (Mb) max used (Mb)
Ncells 5219100 278.8 8529671 455.6 8529671 455.6
Vcells 9841518 75.1 31876688 243.2 60562128 462.1
> rowStats <- microbenchmark(rowLogSumExps = rowLogSumExps(X, na.rm = FALSE), `apply+logSumExp` = apply(X,
+ MARGIN = 1L, FUN = logSumExp, na.rm = FALSE), `apply+logSumExp0` = apply(X, MARGIN = 1L, FUN = logSumExp0,
+ na.rm = FALSE), unit = "ms")
Table: Benchmarking of colLogSumExps(), apply+logSumExp() and apply+logSumExp0() on 100x1000 data. The top panel shows times in milliseconds and the bottom panel shows relative times.
|
expr |
min |
lq |
mean |
median |
uq |
max |
1 |
colLogSumExps |
1.624391 |
1.801557 |
1.831439 |
1.812264 |
1.841316 |
2.302175 |
2 |
apply+logSumExp |
3.776501 |
4.174838 |
4.484965 |
4.217352 |
4.271068 |
25.059164 |
3 |
apply+logSumExp0 |
5.371786 |
5.919493 |
6.429257 |
5.985990 |
6.074941 |
27.184728 |
|
expr |
min |
lq |
mean |
median |
uq |
max |
1 |
colLogSumExps |
1.000000 |
1.000000 |
1.000000 |
1.000000 |
1.000000 |
1.00000 |
2 |
apply+logSumExp |
2.324872 |
2.317349 |
2.448875 |
2.327118 |
2.319573 |
10.88500 |
3 |
apply+logSumExp0 |
3.306954 |
3.285764 |
3.510495 |
3.303046 |
3.299238 |
11.80828 |
Table: Benchmarking of rowLogSumExps(), apply+logSumExp() and apply+logSumExp0() on 100x1000 data (transposed). The top panel shows times in milliseconds and the bottom panel shows relative times.
|
expr |
min |
lq |
mean |
median |
uq |
max |
1 |
rowLogSumExps |
1.752514 |
1.911463 |
1.957864 |
1.923861 |
1.958441 |
2.867753 |
2 |
apply+logSumExp |
3.780918 |
4.187303 |
4.473450 |
4.231286 |
4.299059 |
25.509167 |
3 |
apply+logSumExp0 |
5.396677 |
5.923896 |
6.441987 |
5.986849 |
6.092803 |
26.030105 |
|
expr |
min |
lq |
mean |
median |
uq |
max |
1 |
rowLogSumExps |
1.000000 |
1.000000 |
1.000000 |
1.000000 |
1.000000 |
1.000000 |
2 |
apply+logSumExp |
2.157425 |
2.190627 |
2.284862 |
2.199371 |
2.195143 |
8.895176 |
3 |
apply+logSumExp0 |
3.079392 |
3.099142 |
3.290314 |
3.111892 |
3.111047 |
9.076830 |
Figure: Benchmarking of colLogSumExps(), apply+logSumExp() and apply+logSumExp0() on 100x1000 data as well as rowLogSumExps(), apply+logSumExp() and apply+logSumExp0() on the same data transposed. Outliers are displayed as crosses. Times are in milliseconds.
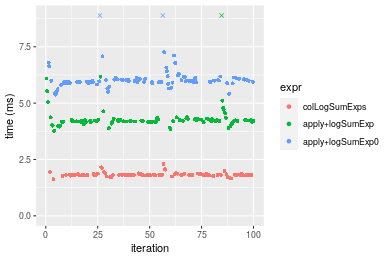
Table: Benchmarking of colLogSumExps() and rowLogSumExps() on 100x1000 data (original and transposed). The top panel shows times in milliseconds and the bottom panel shows relative times.
|
expr |
min |
lq |
mean |
median |
uq |
max |
1 |
colLogSumExps |
1.624391 |
1.801557 |
1.831439 |
1.812264 |
1.841316 |
2.302175 |
2 |
rowLogSumExps |
1.752514 |
1.911463 |
1.957864 |
1.923861 |
1.958441 |
2.867753 |
|
expr |
min |
lq |
mean |
median |
uq |
max |
1 |
colLogSumExps |
1.000000 |
1.000000 |
1.000000 |
1.000000 |
1.00000 |
1.000000 |
2 |
rowLogSumExps |
1.078874 |
1.061006 |
1.069031 |
1.061579 |
1.06361 |
1.245671 |
Figure: Benchmarking of colLogSumExps() and rowLogSumExps() on 100x1000 data (original and transposed). Outliers are displayed as crosses. Times are in milliseconds.
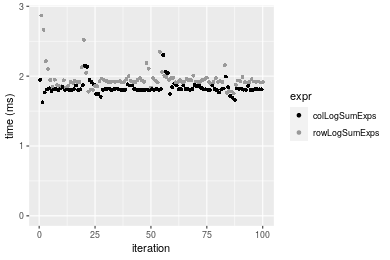
1000x100 matrix
> X <- data[["1000x100"]]
> gc()
used (Mb) gc trigger (Mb) max used (Mb)
Ncells 5219312 278.8 8529671 455.6 8529671 455.6
Vcells 9742136 74.4 31876688 243.2 60562128 462.1
> colStats <- microbenchmark(colLogSumExps = colLogSumExps(X, na.rm = FALSE), `apply+logSumExp` = apply(X,
+ MARGIN = 2L, FUN = logSumExp, na.rm = FALSE), `apply+logSumExp0` = apply(X, MARGIN = 2L, FUN = logSumExp0,
+ na.rm = FALSE), unit = "ms")
> X <- t(X)
> gc()
used (Mb) gc trigger (Mb) max used (Mb)
Ncells 5219306 278.8 8529671 455.6 8529671 455.6
Vcells 9842179 75.1 31876688 243.2 60562128 462.1
> rowStats <- microbenchmark(rowLogSumExps = rowLogSumExps(X, na.rm = FALSE), `apply+logSumExp` = apply(X,
+ MARGIN = 1L, FUN = logSumExp, na.rm = FALSE), `apply+logSumExp0` = apply(X, MARGIN = 1L, FUN = logSumExp0,
+ na.rm = FALSE), unit = "ms")
Table: Benchmarking of colLogSumExps(), apply+logSumExp() and apply+logSumExp0() on 1000x100 data. The top panel shows times in milliseconds and the bottom panel shows relative times.
|
expr |
min |
lq |
mean |
median |
uq |
max |
1 |
colLogSumExps |
1.517303 |
1.733855 |
1.773318 |
1.779090 |
1.800228 |
2.310471 |
2 |
apply+logSumExp |
2.216948 |
2.432158 |
2.553887 |
2.474226 |
2.508740 |
10.567098 |
3 |
apply+logSumExp0 |
2.592625 |
2.825159 |
3.043045 |
2.875790 |
2.923199 |
11.135143 |
|
expr |
min |
lq |
mean |
median |
uq |
max |
1 |
colLogSumExps |
1.000000 |
1.000000 |
1.000000 |
1.000000 |
1.000000 |
1.000000 |
2 |
apply+logSumExp |
1.461111 |
1.402746 |
1.440174 |
1.390725 |
1.393568 |
4.573569 |
3 |
apply+logSumExp0 |
1.708706 |
1.629409 |
1.716017 |
1.616438 |
1.623794 |
4.819426 |
Table: Benchmarking of rowLogSumExps(), apply+logSumExp() and apply+logSumExp0() on 1000x100 data (transposed). The top panel shows times in milliseconds and the bottom panel shows relative times.
|
expr |
min |
lq |
mean |
median |
uq |
max |
1 |
rowLogSumExps |
1.610505 |
1.838343 |
1.878647 |
1.893433 |
1.907017 |
2.480900 |
2 |
apply+logSumExp |
2.181577 |
2.453104 |
2.513237 |
2.514174 |
2.556699 |
3.612257 |
3 |
apply+logSumExp0 |
2.651415 |
2.857989 |
3.184226 |
2.938034 |
3.005631 |
11.197049 |
|
expr |
min |
lq |
mean |
median |
uq |
max |
1 |
rowLogSumExps |
1.000000 |
1.000000 |
1.000000 |
1.000000 |
1.00000 |
1.000000 |
2 |
apply+logSumExp |
1.354592 |
1.334411 |
1.337791 |
1.327839 |
1.34068 |
1.456027 |
3 |
apply+logSumExp0 |
1.646325 |
1.554655 |
1.694957 |
1.551697 |
1.57609 |
4.513301 |
Figure: Benchmarking of colLogSumExps(), apply+logSumExp() and apply+logSumExp0() on 1000x100 data as well as rowLogSumExps(), apply+logSumExp() and apply+logSumExp0() on the same data transposed. Outliers are displayed as crosses. Times are in milliseconds.
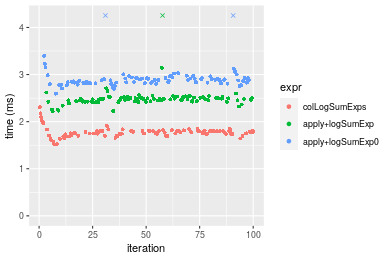
Table: Benchmarking of colLogSumExps() and rowLogSumExps() on 1000x100 data (original and transposed). The top panel shows times in milliseconds and the bottom panel shows relative times.
|
expr |
min |
lq |
mean |
median |
uq |
max |
1 |
colLogSumExps |
1.517303 |
1.733855 |
1.773318 |
1.779090 |
1.800228 |
2.310471 |
2 |
rowLogSumExps |
1.610505 |
1.838343 |
1.878647 |
1.893433 |
1.907017 |
2.480900 |
|
expr |
min |
lq |
mean |
median |
uq |
max |
1 |
colLogSumExps |
1.000000 |
1.000000 |
1.000000 |
1.00000 |
1.00000 |
1.000000 |
2 |
rowLogSumExps |
1.061426 |
1.060263 |
1.059396 |
1.06427 |
1.05932 |
1.073764 |
Figure: Benchmarking of colLogSumExps() and rowLogSumExps() on 1000x100 data (original and transposed). Outliers are displayed as crosses. Times are in milliseconds.
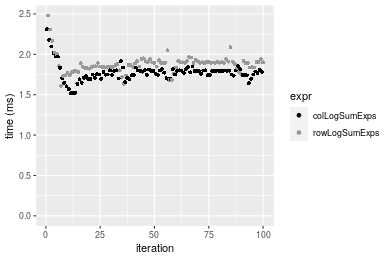
Appendix
R version 4.1.1 Patched (2021-08-10 r80727)
Platform: x86_64-pc-linux-gnu (64-bit)
Running under: Ubuntu 18.04.5 LTS
Matrix products: default
BLAS: /home/hb/software/R-devel/R-4-1-branch/lib/R/lib/libRblas.so
LAPACK: /home/hb/software/R-devel/R-4-1-branch/lib/R/lib/libRlapack.so
locale:
[1] LC_CTYPE=en_US.UTF-8 LC_NUMERIC=C
[3] LC_TIME=en_US.UTF-8 LC_COLLATE=en_US.UTF-8
[5] LC_MONETARY=en_US.UTF-8 LC_MESSAGES=en_US.UTF-8
[7] LC_PAPER=en_US.UTF-8 LC_NAME=C
[9] LC_ADDRESS=C LC_TELEPHONE=C
[11] LC_MEASUREMENT=en_US.UTF-8 LC_IDENTIFICATION=C
attached base packages:
[1] stats graphics grDevices utils datasets methods base
other attached packages:
[1] microbenchmark_1.4-7 matrixStats_0.60.1 ggplot2_3.3.5
[4] knitr_1.33 R.devices_2.17.0 R.utils_2.10.1
[7] R.oo_1.24.0 R.methodsS3_1.8.1-9001 history_0.0.1-9000
loaded via a namespace (and not attached):
[1] Biobase_2.52.0 httr_1.4.2 splines_4.1.1
[4] bit64_4.0.5 network_1.17.1 assertthat_0.2.1
[7] highr_0.9 stats4_4.1.1 blob_1.2.2
[10] GenomeInfoDbData_1.2.6 robustbase_0.93-8 pillar_1.6.2
[13] RSQLite_2.2.8 lattice_0.20-44 glue_1.4.2
[16] digest_0.6.27 XVector_0.32.0 colorspace_2.0-2
[19] Matrix_1.3-4 XML_3.99-0.7 pkgconfig_2.0.3
[22] zlibbioc_1.38.0 genefilter_1.74.0 purrr_0.3.4
[25] ergm_4.1.2 xtable_1.8-4 scales_1.1.1
[28] tibble_3.1.4 annotate_1.70.0 KEGGREST_1.32.0
[31] farver_2.1.0 generics_0.1.0 IRanges_2.26.0
[34] ellipsis_0.3.2 cachem_1.0.6 withr_2.4.2
[37] BiocGenerics_0.38.0 mime_0.11 survival_3.2-13
[40] magrittr_2.0.1 crayon_1.4.1 statnet.common_4.5.0
[43] memoise_2.0.0 laeken_0.5.1 fansi_0.5.0
[46] R.cache_0.15.0 MASS_7.3-54 R.rsp_0.44.0
[49] progressr_0.8.0 tools_4.1.1 lifecycle_1.0.0
[52] S4Vectors_0.30.0 trust_0.1-8 munsell_0.5.0
[55] tabby_0.0.1-9001 AnnotationDbi_1.54.1 Biostrings_2.60.2
[58] compiler_4.1.1 GenomeInfoDb_1.28.1 rlang_0.4.11
[61] grid_4.1.1 RCurl_1.98-1.4 cwhmisc_6.6
[64] rappdirs_0.3.3 startup_0.15.0 labeling_0.4.2
[67] bitops_1.0-7 base64enc_0.1-3 boot_1.3-28
[70] gtable_0.3.0 DBI_1.1.1 markdown_1.1
[73] R6_2.5.1 lpSolveAPI_5.5.2.0-17.7 rle_0.9.2
[76] dplyr_1.0.7 fastmap_1.1.0 bit_4.0.4
[79] utf8_1.2.2 parallel_4.1.1 Rcpp_1.0.7
[82] vctrs_0.3.8 png_0.1-7 DEoptimR_1.0-9
[85] tidyselect_1.1.1 xfun_0.25 coda_0.19-4
Total processing time was 16.57 secs.
Reproducibility
To reproduce this report, do:
html <- matrixStats:::benchmark('colLogSumExps')
Copyright Henrik Bengtsson. Last updated on 2021-08-25 18:56:13 (+0200 UTC). Powered by RSP.