colAnys() and rowAnys() benchmarks
This report benchmark the performance of colAnys() and rowAnys() against alternative methods.
Alternative methods
- apply() + any()
- colSums() > 0 or rowSums() > 0
Data
> rmatrix <- function(nrow, ncol, mode = c("logical", "double", "integer", "index"), range = c(-100,
+ +100), na_prob = 0) {
+ mode <- match.arg(mode)
+ n <- nrow * ncol
+ if (mode == "logical") {
+ x <- sample(c(FALSE, TRUE), size = n, replace = TRUE)
+ } else if (mode == "index") {
+ x <- seq_len(n)
+ mode <- "integer"
+ } else {
+ x <- runif(n, min = range[1], max = range[2])
+ }
+ storage.mode(x) <- mode
+ if (na_prob > 0)
+ x[sample(n, size = na_prob * n)] <- NA
+ dim(x) <- c(nrow, ncol)
+ x
+ }
> rmatrices <- function(scale = 10, seed = 1, ...) {
+ set.seed(seed)
+ data <- list()
+ data[[1]] <- rmatrix(nrow = scale * 1, ncol = scale * 1, ...)
+ data[[2]] <- rmatrix(nrow = scale * 10, ncol = scale * 10, ...)
+ data[[3]] <- rmatrix(nrow = scale * 100, ncol = scale * 1, ...)
+ data[[4]] <- t(data[[3]])
+ data[[5]] <- rmatrix(nrow = scale * 10, ncol = scale * 100, ...)
+ data[[6]] <- t(data[[5]])
+ names(data) <- sapply(data, FUN = function(x) paste(dim(x), collapse = "x"))
+ data
+ }
> data <- rmatrices(mode = "logical")
Results
10x10 matrix
> X <- data[["10x10"]]
> gc()
used (Mb) gc trigger (Mb) max used (Mb)
Ncells 5179746 276.7 8529671 455.6 8529671 455.6
Vcells 9512341 72.6 31876688 243.2 60562128 462.1
> colStats <- microbenchmark(colAnys = colAnys(X), `apply+any` = apply(X, MARGIN = 2L, FUN = any),
+ `colSums > 0` = (colSums(X) > 0L), unit = "ms")
> X <- t(X)
> gc()
used (Mb) gc trigger (Mb) max used (Mb)
Ncells 5171145 276.2 8529671 455.6 8529671 455.6
Vcells 9484279 72.4 31876688 243.2 60562128 462.1
> rowStats <- microbenchmark(rowAnys = rowAnys(X), `apply+any` = apply(X, MARGIN = 1L, FUN = any),
+ `rowSums > 0` = (rowSums(X) > 0L), unit = "ms")
Table: Benchmarking of colAnys(), apply+any() and colSums > 0() on 10x10 data. The top panel shows times in milliseconds and the bottom panel shows relative times.
|
expr |
min |
lq |
mean |
median |
uq |
max |
1 |
colAnys |
0.003369 |
0.0037380 |
0.0045488 |
0.0046250 |
0.0048980 |
0.019739 |
3 |
colSums > 0 |
0.006011 |
0.0063950 |
0.0076344 |
0.0072150 |
0.0077430 |
0.036104 |
2 |
apply+any |
0.033466 |
0.0343405 |
0.0360280 |
0.0350695 |
0.0358385 |
0.081195 |
|
expr |
min |
lq |
mean |
median |
uq |
max |
1 |
colAnys |
1.000000 |
1.000000 |
1.000000 |
1.000000 |
1.000000 |
1.000000 |
3 |
colSums > 0 |
1.784209 |
1.710808 |
1.678334 |
1.560000 |
1.580849 |
1.829069 |
2 |
apply+any |
9.933511 |
9.186865 |
7.920285 |
7.582595 |
7.316966 |
4.113430 |
Table: Benchmarking of rowAnys(), apply+any() and rowSums > 0() on 10x10 data (transposed). The top panel shows times in milliseconds and the bottom panel shows relative times.
|
expr |
min |
lq |
mean |
median |
uq |
max |
1 |
rowAnys |
0.003514 |
0.0038390 |
0.0047763 |
0.0049670 |
0.0052155 |
0.018834 |
3 |
rowSums > 0 |
0.006739 |
0.0070855 |
0.0079660 |
0.0076285 |
0.0082740 |
0.029251 |
2 |
apply+any |
0.033386 |
0.0343190 |
0.0356151 |
0.0348535 |
0.0355940 |
0.081946 |
|
expr |
min |
lq |
mean |
median |
uq |
max |
1 |
rowAnys |
1.000000 |
1.000000 |
1.000000 |
1.000000 |
1.000000 |
1.000000 |
3 |
rowSums > 0 |
1.917758 |
1.845663 |
1.667837 |
1.535837 |
1.586425 |
1.553095 |
2 |
apply+any |
9.500854 |
8.939568 |
7.456670 |
7.017012 |
6.824657 |
4.350961 |
Figure: Benchmarking of colAnys(), apply+any() and colSums > 0() on 10x10 data as well as rowAnys(), apply+any() and rowSums > 0() on the same data transposed. Outliers are displayed as crosses. Times are in milliseconds.
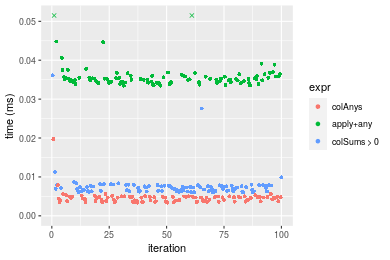
Table: Benchmarking of colAnys() and rowAnys() on 10x10 data (original and transposed). The top panel shows times in milliseconds and the bottom panel shows relative times.
|
expr |
min |
lq |
mean |
median |
uq |
max |
1 |
colAnys |
3.369 |
3.738 |
4.54882 |
4.625 |
4.8980 |
19.739 |
2 |
rowAnys |
3.514 |
3.839 |
4.77627 |
4.967 |
5.2155 |
18.834 |
|
expr |
min |
lq |
mean |
median |
uq |
max |
1 |
colAnys |
1.000000 |
1.00000 |
1.000000 |
1.000000 |
1.000000 |
1.0000000 |
2 |
rowAnys |
1.043039 |
1.02702 |
1.050002 |
1.073946 |
1.064822 |
0.9541517 |
Figure: Benchmarking of colAnys() and rowAnys() on 10x10 data (original and transposed). Outliers are displayed as crosses. Times are in milliseconds.
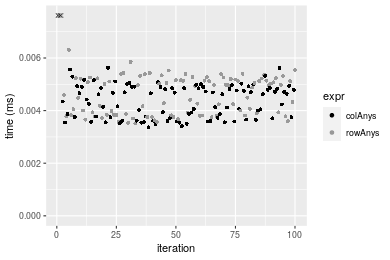
100x100 matrix
> X <- data[["100x100"]]
> gc()
used (Mb) gc trigger (Mb) max used (Mb)
Ncells 5169723 276.1 8529671 455.6 8529671 455.6
Vcells 9290506 70.9 31876688 243.2 60562128 462.1
> colStats <- microbenchmark(colAnys = colAnys(X), `apply+any` = apply(X, MARGIN = 2L, FUN = any),
+ `colSums > 0` = (colSums(X) > 0L), unit = "ms")
> X <- t(X)
> gc()
used (Mb) gc trigger (Mb) max used (Mb)
Ncells 5169699 276.1 8529671 455.6 8529671 455.6
Vcells 9295519 71.0 31876688 243.2 60562128 462.1
> rowStats <- microbenchmark(rowAnys = rowAnys(X), `apply+any` = apply(X, MARGIN = 1L, FUN = any),
+ `rowSums > 0` = (rowSums(X) > 0L), unit = "ms")
Table: Benchmarking of colAnys(), apply+any() and colSums > 0() on 100x100 data. The top panel shows times in milliseconds and the bottom panel shows relative times.
|
expr |
min |
lq |
mean |
median |
uq |
max |
1 |
colAnys |
0.003781 |
0.0046570 |
0.0058371 |
0.0055565 |
0.0061375 |
0.027023 |
3 |
colSums > 0 |
0.015332 |
0.0170265 |
0.0188621 |
0.0180650 |
0.0200460 |
0.041649 |
2 |
apply+any |
0.198164 |
0.2068690 |
0.2289510 |
0.2231090 |
0.2528385 |
0.328815 |
|
expr |
min |
lq |
mean |
median |
uq |
max |
1 |
colAnys |
1.000000 |
1.000000 |
1.000000 |
1.000000 |
1.000000 |
1.000000 |
3 |
colSums > 0 |
4.055012 |
3.656109 |
3.231403 |
3.251147 |
3.266151 |
1.541243 |
2 |
apply+any |
52.410473 |
44.421087 |
39.223278 |
40.152794 |
41.195682 |
12.167968 |
Table: Benchmarking of rowAnys(), apply+any() and rowSums > 0() on 100x100 data (transposed). The top panel shows times in milliseconds and the bottom panel shows relative times.
|
expr |
min |
lq |
mean |
median |
uq |
max |
1 |
rowAnys |
0.010892 |
0.012502 |
0.0140718 |
0.0134200 |
0.0150110 |
0.032720 |
3 |
rowSums > 0 |
0.044789 |
0.047577 |
0.0527260 |
0.0512480 |
0.0580985 |
0.082739 |
2 |
apply+any |
0.188202 |
0.196207 |
0.2221017 |
0.2157725 |
0.2457590 |
0.327130 |
|
expr |
min |
lq |
mean |
median |
uq |
max |
1 |
rowAnys |
1.000000 |
1.000000 |
1.000000 |
1.000000 |
1.000000 |
1.000000 |
3 |
rowSums > 0 |
4.112101 |
3.805551 |
3.746918 |
3.818778 |
3.870395 |
2.528698 |
2 |
apply+any |
17.278920 |
15.694049 |
15.783439 |
16.078428 |
16.371927 |
9.997861 |
Figure: Benchmarking of colAnys(), apply+any() and colSums > 0() on 100x100 data as well as rowAnys(), apply+any() and rowSums > 0() on the same data transposed. Outliers are displayed as crosses. Times are in milliseconds.
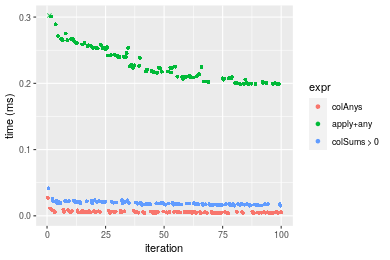
Table: Benchmarking of colAnys() and rowAnys() on 100x100 data (original and transposed). The top panel shows times in milliseconds and the bottom panel shows relative times.
|
expr |
min |
lq |
mean |
median |
uq |
max |
1 |
colAnys |
3.781 |
4.657 |
5.83712 |
5.5565 |
6.1375 |
27.023 |
2 |
rowAnys |
10.892 |
12.502 |
14.07182 |
13.4200 |
15.0110 |
32.720 |
|
expr |
min |
lq |
mean |
median |
uq |
max |
1 |
colAnys |
1.000000 |
1.000000 |
1.000000 |
1.000000 |
1.000000 |
1.00000 |
2 |
rowAnys |
2.880719 |
2.684561 |
2.410747 |
2.415189 |
2.445784 |
1.21082 |
Figure: Benchmarking of colAnys() and rowAnys() on 100x100 data (original and transposed). Outliers are displayed as crosses. Times are in milliseconds.
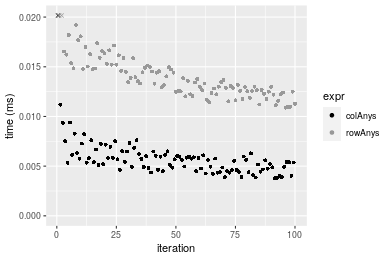
1000x10 matrix
> X <- data[["1000x10"]]
> gc()
used (Mb) gc trigger (Mb) max used (Mb)
Ncells 5170455 276.2 8529671 455.6 8529671 455.6
Vcells 9294000 71.0 31876688 243.2 60562128 462.1
> colStats <- microbenchmark(colAnys = colAnys(X), `apply+any` = apply(X, MARGIN = 2L, FUN = any),
+ `colSums > 0` = (colSums(X) > 0L), unit = "ms")
> X <- t(X)
> gc()
used (Mb) gc trigger (Mb) max used (Mb)
Ncells 5170449 276.2 8529671 455.6 8529671 455.6
Vcells 9299043 71.0 31876688 243.2 60562128 462.1
> rowStats <- microbenchmark(rowAnys = rowAnys(X), `apply+any` = apply(X, MARGIN = 1L, FUN = any),
+ `rowSums > 0` = (rowSums(X) > 0L), unit = "ms")
Table: Benchmarking of colAnys(), apply+any() and colSums > 0() on 1000x10 data. The top panel shows times in milliseconds and the bottom panel shows relative times.
|
expr |
min |
lq |
mean |
median |
uq |
max |
1 |
colAnys |
0.003171 |
0.0038475 |
0.0048026 |
0.0045420 |
0.0050840 |
0.018961 |
3 |
colSums > 0 |
0.017068 |
0.0187995 |
0.0199878 |
0.0196230 |
0.0207900 |
0.035880 |
2 |
apply+any |
0.110909 |
0.1163095 |
0.1237920 |
0.1261835 |
0.1293025 |
0.192247 |
|
expr |
min |
lq |
mean |
median |
uq |
max |
1 |
colAnys |
1.000000 |
1.00000 |
1.000000 |
1.000000 |
1.00000 |
1.000000 |
3 |
colSums > 0 |
5.382529 |
4.88616 |
4.161841 |
4.320343 |
4.08930 |
1.892305 |
2 |
apply+any |
34.976033 |
30.22989 |
25.775869 |
27.781484 |
25.43322 |
10.139075 |
Table: Benchmarking of rowAnys(), apply+any() and rowSums > 0() on 1000x10 data (transposed). The top panel shows times in milliseconds and the bottom panel shows relative times.
|
expr |
min |
lq |
mean |
median |
uq |
max |
1 |
rowAnys |
0.011827 |
0.0140215 |
0.0160236 |
0.0152930 |
0.0170775 |
0.030842 |
2 |
apply+any |
0.097301 |
0.1010985 |
0.1194628 |
0.1143040 |
0.1299365 |
0.195430 |
3 |
rowSums > 0 |
0.167845 |
0.1742380 |
0.1923458 |
0.1928465 |
0.2093100 |
0.230652 |
|
expr |
min |
lq |
mean |
median |
uq |
max |
1 |
rowAnys |
1.000000 |
1.000000 |
1.000000 |
1.000000 |
1.000000 |
1.000000 |
2 |
apply+any |
8.227023 |
7.210248 |
7.455427 |
7.474269 |
7.608637 |
6.336489 |
3 |
rowSums > 0 |
14.191680 |
12.426488 |
12.003899 |
12.610116 |
12.256478 |
7.478503 |
Figure: Benchmarking of colAnys(), apply+any() and colSums > 0() on 1000x10 data as well as rowAnys(), apply+any() and rowSums > 0() on the same data transposed. Outliers are displayed as crosses. Times are in milliseconds.
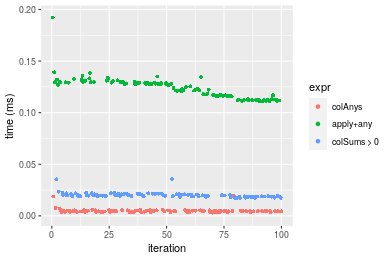
Table: Benchmarking of colAnys() and rowAnys() on 1000x10 data (original and transposed). The top panel shows times in milliseconds and the bottom panel shows relative times.
|
expr |
min |
lq |
mean |
median |
uq |
max |
1 |
colAnys |
3.171 |
3.8475 |
4.80263 |
4.542 |
5.0840 |
18.961 |
2 |
rowAnys |
11.827 |
14.0215 |
16.02361 |
15.293 |
17.0775 |
30.842 |
|
expr |
min |
lq |
mean |
median |
uq |
max |
1 |
colAnys |
1.000000 |
1.000000 |
1.000000 |
1.000000 |
1.000000 |
1.000000 |
2 |
rowAnys |
3.729738 |
3.644315 |
3.336424 |
3.367019 |
3.359068 |
1.626602 |
Figure: Benchmarking of colAnys() and rowAnys() on 1000x10 data (original and transposed). Outliers are displayed as crosses. Times are in milliseconds.
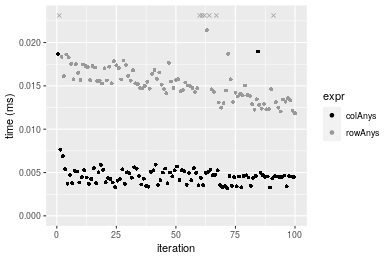
10x1000 matrix
> X <- data[["10x1000"]]
> gc()
used (Mb) gc trigger (Mb) max used (Mb)
Ncells 5170687 276.2 8529671 455.6 8529671 455.6
Vcells 9294767 71.0 31876688 243.2 60562128 462.1
> colStats <- microbenchmark(colAnys = colAnys(X), `apply+any` = apply(X, MARGIN = 2L, FUN = any),
+ `colSums > 0` = (colSums(X) > 0L), unit = "ms")
> X <- t(X)
> gc()
used (Mb) gc trigger (Mb) max used (Mb)
Ncells 5170663 276.2 8529671 455.6 8529671 455.6
Vcells 9299780 71.0 31876688 243.2 60562128 462.1
> rowStats <- microbenchmark(rowAnys = rowAnys(X), `apply+any` = apply(X, MARGIN = 1L, FUN = any),
+ `rowSums > 0` = (rowSums(X) > 0L), unit = "ms")
Table: Benchmarking of colAnys(), apply+any() and colSums > 0() on 10x1000 data. The top panel shows times in milliseconds and the bottom panel shows relative times.
|
expr |
min |
lq |
mean |
median |
uq |
max |
3 |
colSums > 0 |
0.013500 |
0.0148425 |
0.0169421 |
0.0157770 |
0.0177990 |
0.038084 |
1 |
colAnys |
0.015037 |
0.0169750 |
0.0196664 |
0.0184330 |
0.0205435 |
0.043810 |
2 |
apply+any |
0.863858 |
0.9135615 |
1.0252330 |
0.9707885 |
1.0985930 |
1.429867 |
|
expr |
min |
lq |
mean |
median |
uq |
max |
3 |
colSums > 0 |
1.000000 |
1.000000 |
1.00000 |
1.000000 |
1.000000 |
1.000000 |
1 |
colAnys |
1.113852 |
1.143675 |
1.16080 |
1.168346 |
1.154194 |
1.150352 |
2 |
apply+any |
63.989481 |
61.550379 |
60.51389 |
61.531882 |
61.722175 |
37.545085 |
Table: Benchmarking of rowAnys(), apply+any() and rowSums > 0() on 10x1000 data (transposed). The top panel shows times in milliseconds and the bottom panel shows relative times.
|
expr |
min |
lq |
mean |
median |
uq |
max |
1 |
rowAnys |
0.025629 |
0.0292995 |
0.0344592 |
0.031665 |
0.038367 |
0.073215 |
3 |
rowSums > 0 |
0.028577 |
0.0308205 |
0.0357424 |
0.032762 |
0.038074 |
0.067021 |
2 |
apply+any |
0.870325 |
0.9096820 |
1.0249298 |
0.947311 |
1.108584 |
1.530552 |
|
expr |
min |
lq |
mean |
median |
uq |
max |
1 |
rowAnys |
1.000000 |
1.000000 |
1.000000 |
1.000000 |
1.0000000 |
1.0000000 |
3 |
rowSums > 0 |
1.115026 |
1.051912 |
1.037236 |
1.034644 |
0.9923632 |
0.9153998 |
2 |
apply+any |
33.958602 |
31.047697 |
29.743259 |
29.916659 |
28.8942060 |
20.9048965 |
Figure: Benchmarking of colAnys(), apply+any() and colSums > 0() on 10x1000 data as well as rowAnys(), apply+any() and rowSums > 0() on the same data transposed. Outliers are displayed as crosses. Times are in milliseconds.
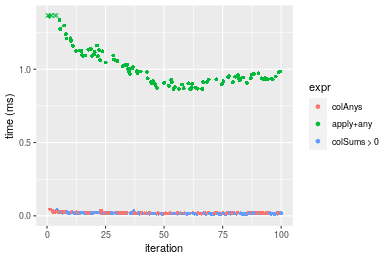
Table: Benchmarking of colAnys() and rowAnys() on 10x1000 data (original and transposed). The top panel shows times in milliseconds and the bottom panel shows relative times.
|
expr |
min |
lq |
mean |
median |
uq |
max |
1 |
colAnys |
15.037 |
16.9750 |
19.66641 |
18.433 |
20.5435 |
43.810 |
2 |
rowAnys |
25.629 |
29.2995 |
34.45923 |
31.665 |
38.3670 |
73.215 |
|
expr |
min |
lq |
mean |
median |
uq |
max |
1 |
colAnys |
1.000000 |
1.000000 |
1.000000 |
1.000000 |
1.000000 |
1.000000 |
2 |
rowAnys |
1.704396 |
1.726038 |
1.752187 |
1.717843 |
1.867598 |
1.671194 |
Figure: Benchmarking of colAnys() and rowAnys() on 10x1000 data (original and transposed). Outliers are displayed as crosses. Times are in milliseconds.
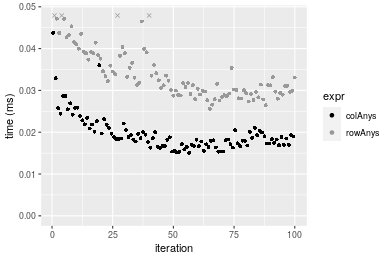
100x1000 matrix
> X <- data[["100x1000"]]
> gc()
used (Mb) gc trigger (Mb) max used (Mb)
Ncells 5170877 276.2 8529671 455.6 8529671 455.6
Vcells 9295254 71.0 31876688 243.2 60562128 462.1
> colStats <- microbenchmark(colAnys = colAnys(X), `apply+any` = apply(X, MARGIN = 2L, FUN = any),
+ `colSums > 0` = (colSums(X) > 0L), unit = "ms")
> X <- t(X)
> gc()
used (Mb) gc trigger (Mb) max used (Mb)
Ncells 5170865 276.2 8529671 455.6 8529671 455.6
Vcells 9345287 71.3 31876688 243.2 60562128 462.1
> rowStats <- microbenchmark(rowAnys = rowAnys(X), `apply+any` = apply(X, MARGIN = 1L, FUN = any),
+ `rowSums > 0` = (rowSums(X) > 0L), unit = "ms")
Table: Benchmarking of colAnys(), apply+any() and colSums > 0() on 100x1000 data. The top panel shows times in milliseconds and the bottom panel shows relative times.
|
expr |
min |
lq |
mean |
median |
uq |
max |
1 |
colAnys |
0.016351 |
0.0184375 |
0.0205998 |
0.0196440 |
0.021219 |
0.044673 |
3 |
colSums > 0 |
0.081160 |
0.0879225 |
0.0936510 |
0.0907155 |
0.094624 |
0.143238 |
2 |
apply+any |
1.371886 |
1.4840435 |
1.5837545 |
1.5252055 |
1.567319 |
2.514333 |
|
expr |
min |
lq |
mean |
median |
uq |
max |
1 |
colAnys |
1.000000 |
1.000000 |
1.000000 |
1.000000 |
1.00000 |
1.000000 |
3 |
colSums > 0 |
4.963611 |
4.768678 |
4.546215 |
4.617975 |
4.45940 |
3.206366 |
2 |
apply+any |
83.902269 |
80.490495 |
76.882146 |
77.642308 |
73.86394 |
56.283057 |
Table: Benchmarking of rowAnys(), apply+any() and rowSums > 0() on 100x1000 data (transposed). The top panel shows times in milliseconds and the bottom panel shows relative times.
|
expr |
min |
lq |
mean |
median |
uq |
max |
1 |
rowAnys |
0.072548 |
0.0807825 |
0.0865152 |
0.082799 |
0.087472 |
0.152590 |
3 |
rowSums > 0 |
0.230758 |
0.2500765 |
0.2709630 |
0.253867 |
0.277809 |
0.395284 |
2 |
apply+any |
1.374199 |
1.4937530 |
1.5805760 |
1.531343 |
1.588420 |
2.482158 |
|
expr |
min |
lq |
mean |
median |
uq |
max |
1 |
rowAnys |
1.000000 |
1.000000 |
1.00000 |
1.000000 |
1.000000 |
1.000000 |
3 |
rowSums > 0 |
3.180763 |
3.095677 |
3.13197 |
3.066064 |
3.175976 |
2.590497 |
2 |
apply+any |
18.941928 |
18.491047 |
18.26934 |
18.494704 |
18.159177 |
16.266846 |
Figure: Benchmarking of colAnys(), apply+any() and colSums > 0() on 100x1000 data as well as rowAnys(), apply+any() and rowSums > 0() on the same data transposed. Outliers are displayed as crosses. Times are in milliseconds.
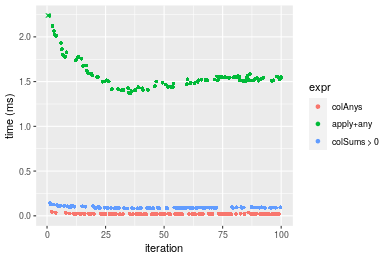
Table: Benchmarking of colAnys() and rowAnys() on 100x1000 data (original and transposed). The top panel shows times in milliseconds and the bottom panel shows relative times.
|
expr |
min |
lq |
mean |
median |
uq |
max |
1 |
colAnys |
16.351 |
18.4375 |
20.59977 |
19.644 |
21.219 |
44.673 |
2 |
rowAnys |
72.548 |
80.7825 |
86.51521 |
82.799 |
87.472 |
152.590 |
|
expr |
min |
lq |
mean |
median |
uq |
max |
1 |
colAnys |
1.000000 |
1.000000 |
1.000000 |
1.000000 |
1.000000 |
1.00000 |
2 |
rowAnys |
4.436915 |
4.381424 |
4.199814 |
4.214977 |
4.122343 |
3.41571 |
Figure: Benchmarking of colAnys() and rowAnys() on 100x1000 data (original and transposed). Outliers are displayed as crosses. Times are in milliseconds.
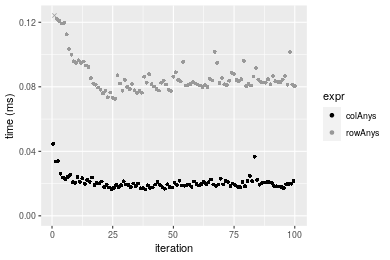
1000x100 matrix
> X <- data[["1000x100"]]
> gc()
used (Mb) gc trigger (Mb) max used (Mb)
Ncells 5171083 276.2 8529671 455.6 8529671 455.6
Vcells 9295919 71.0 31876688 243.2 60562128 462.1
> colStats <- microbenchmark(colAnys = colAnys(X), `apply+any` = apply(X, MARGIN = 2L, FUN = any),
+ `colSums > 0` = (colSums(X) > 0L), unit = "ms")
> X <- t(X)
> gc()
used (Mb) gc trigger (Mb) max used (Mb)
Ncells 5171071 276.2 8529671 455.6 8529671 455.6
Vcells 9345952 71.4 31876688 243.2 60562128 462.1
> rowStats <- microbenchmark(rowAnys = rowAnys(X), `apply+any` = apply(X, MARGIN = 1L, FUN = any),
+ `rowSums > 0` = (rowSums(X) > 0L), unit = "ms")
Table: Benchmarking of colAnys(), apply+any() and colSums > 0() on 1000x100 data. The top panel shows times in milliseconds and the bottom panel shows relative times.
|
expr |
min |
lq |
mean |
median |
uq |
max |
1 |
colAnys |
0.003413 |
0.0045435 |
0.0060280 |
0.0054805 |
0.0067385 |
0.030601 |
3 |
colSums > 0 |
0.082798 |
0.0877285 |
0.1015405 |
0.0931570 |
0.1120050 |
0.165285 |
2 |
apply+any |
0.634206 |
0.6663190 |
0.7640796 |
0.7152840 |
0.8382390 |
1.114133 |
|
expr |
min |
lq |
mean |
median |
uq |
max |
1 |
colAnys |
1.0000 |
1.00000 |
1.00000 |
1.0000 |
1.00000 |
1.000000 |
3 |
colSums > 0 |
24.2596 |
19.30857 |
16.84487 |
16.9979 |
16.62165 |
5.401294 |
2 |
apply+any |
185.8207 |
146.65324 |
126.75549 |
130.5144 |
124.39549 |
36.408385 |
Table: Benchmarking of rowAnys(), apply+any() and rowSums > 0() on 1000x100 data (transposed). The top panel shows times in milliseconds and the bottom panel shows relative times.
|
expr |
min |
lq |
mean |
median |
uq |
max |
1 |
rowAnys |
0.060689 |
0.0652140 |
0.0730753 |
0.0679255 |
0.0793035 |
0.116305 |
3 |
rowSums > 0 |
0.324218 |
0.3425475 |
0.3810645 |
0.3611855 |
0.4070895 |
0.537751 |
2 |
apply+any |
0.641484 |
0.6636305 |
0.7398351 |
0.6933770 |
0.7514955 |
1.161896 |
|
expr |
min |
lq |
mean |
median |
uq |
max |
1 |
rowAnys |
1.000000 |
1.000000 |
1.00000 |
1.000000 |
1.000000 |
1.000000 |
3 |
rowSums > 0 |
5.342286 |
5.252668 |
5.21468 |
5.317377 |
5.133311 |
4.623627 |
2 |
apply+any |
10.570021 |
10.176197 |
10.12428 |
10.207904 |
9.476196 |
9.990078 |
Figure: Benchmarking of colAnys(), apply+any() and colSums > 0() on 1000x100 data as well as rowAnys(), apply+any() and rowSums > 0() on the same data transposed. Outliers are displayed as crosses. Times are in milliseconds.
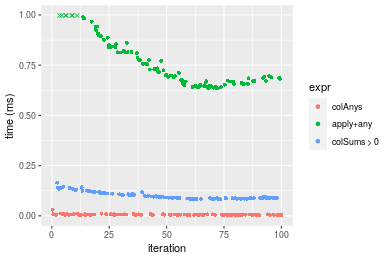
Table: Benchmarking of colAnys() and rowAnys() on 1000x100 data (original and transposed). The top panel shows times in milliseconds and the bottom panel shows relative times.
|
expr |
min |
lq |
mean |
median |
uq |
max |
1 |
colAnys |
3.413 |
4.5435 |
6.02798 |
5.4805 |
6.7385 |
30.601 |
2 |
rowAnys |
60.689 |
65.2140 |
73.07533 |
67.9255 |
79.3035 |
116.305 |
|
expr |
min |
lq |
mean |
median |
uq |
max |
1 |
colAnys |
1.00000 |
1.00000 |
1.00000 |
1.00000 |
1.00000 |
1.000000 |
2 |
rowAnys |
17.78172 |
14.35325 |
12.12269 |
12.39403 |
11.76872 |
3.800693 |
Figure: Benchmarking of colAnys() and rowAnys() on 1000x100 data (original and transposed). Outliers are displayed as crosses. Times are in milliseconds.
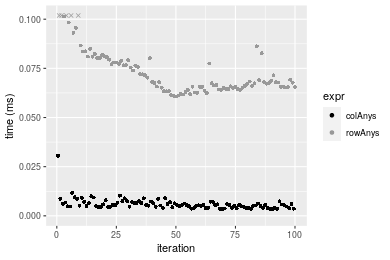
Appendix
R version 4.1.1 Patched (2021-08-10 r80727)
Platform: x86_64-pc-linux-gnu (64-bit)
Running under: Ubuntu 18.04.5 LTS
Matrix products: default
BLAS: /home/hb/software/R-devel/R-4-1-branch/lib/R/lib/libRblas.so
LAPACK: /home/hb/software/R-devel/R-4-1-branch/lib/R/lib/libRlapack.so
locale:
[1] LC_CTYPE=en_US.UTF-8 LC_NUMERIC=C
[3] LC_TIME=en_US.UTF-8 LC_COLLATE=en_US.UTF-8
[5] LC_MONETARY=en_US.UTF-8 LC_MESSAGES=en_US.UTF-8
[7] LC_PAPER=en_US.UTF-8 LC_NAME=C
[9] LC_ADDRESS=C LC_TELEPHONE=C
[11] LC_MEASUREMENT=en_US.UTF-8 LC_IDENTIFICATION=C
attached base packages:
[1] stats graphics grDevices utils datasets methods base
other attached packages:
[1] microbenchmark_1.4-7 matrixStats_0.60.1 ggplot2_3.3.5
[4] knitr_1.33 R.devices_2.17.0 R.utils_2.10.1
[7] R.oo_1.24.0 R.methodsS3_1.8.1-9001 history_0.0.1-9000
loaded via a namespace (and not attached):
[1] Biobase_2.52.0 httr_1.4.2 splines_4.1.1
[4] bit64_4.0.5 network_1.17.1 assertthat_0.2.1
[7] highr_0.9 stats4_4.1.1 blob_1.2.2
[10] GenomeInfoDbData_1.2.6 robustbase_0.93-8 pillar_1.6.2
[13] RSQLite_2.2.8 lattice_0.20-44 glue_1.4.2
[16] digest_0.6.27 XVector_0.32.0 colorspace_2.0-2
[19] Matrix_1.3-4 XML_3.99-0.7 pkgconfig_2.0.3
[22] zlibbioc_1.38.0 genefilter_1.74.0 purrr_0.3.4
[25] ergm_4.1.2 xtable_1.8-4 scales_1.1.1
[28] tibble_3.1.4 annotate_1.70.0 KEGGREST_1.32.0
[31] farver_2.1.0 generics_0.1.0 IRanges_2.26.0
[34] ellipsis_0.3.2 cachem_1.0.6 withr_2.4.2
[37] BiocGenerics_0.38.0 mime_0.11 survival_3.2-13
[40] magrittr_2.0.1 crayon_1.4.1 statnet.common_4.5.0
[43] memoise_2.0.0 laeken_0.5.1 fansi_0.5.0
[46] R.cache_0.15.0 MASS_7.3-54 R.rsp_0.44.0
[49] progressr_0.8.0 tools_4.1.1 lifecycle_1.0.0
[52] S4Vectors_0.30.0 trust_0.1-8 munsell_0.5.0
[55] tabby_0.0.1-9001 AnnotationDbi_1.54.1 Biostrings_2.60.2
[58] compiler_4.1.1 GenomeInfoDb_1.28.1 rlang_0.4.11
[61] grid_4.1.1 RCurl_1.98-1.4 cwhmisc_6.6
[64] rappdirs_0.3.3 startup_0.15.0 labeling_0.4.2
[67] bitops_1.0-7 base64enc_0.1-3 boot_1.3-28
[70] gtable_0.3.0 DBI_1.1.1 markdown_1.1
[73] R6_2.5.1 lpSolveAPI_5.5.2.0-17.7 rle_0.9.2
[76] dplyr_1.0.7 fastmap_1.1.0 bit_4.0.4
[79] utf8_1.2.2 parallel_4.1.1 Rcpp_1.0.7
[82] vctrs_0.3.8 png_0.1-7 DEoptimR_1.0-9
[85] tidyselect_1.1.1 xfun_0.25 coda_0.19-4
Total processing time was 11.92 secs.
Reproducibility
To reproduce this report, do:
html <- matrixStats:::benchmark('colAnys')
Copyright Henrik Bengtsson. Last updated on 2021-08-25 18:51:03 (+0200 UTC). Powered by RSP.