colAlls() and rowAlls() benchmarks
This report benchmark the performance of colAlls() and rowAlls() against alternative methods.
Alternative methods
- apply() + all()
- colSums() == n or rowSums() == n
Data
> rmatrix <- function(nrow, ncol, mode = c("logical", "double", "integer", "index"), range = c(-100,
+ +100), na_prob = 0) {
+ mode <- match.arg(mode)
+ n <- nrow * ncol
+ if (mode == "logical") {
+ x <- sample(c(FALSE, TRUE), size = n, replace = TRUE)
+ } else if (mode == "index") {
+ x <- seq_len(n)
+ mode <- "integer"
+ } else {
+ x <- runif(n, min = range[1], max = range[2])
+ }
+ storage.mode(x) <- mode
+ if (na_prob > 0)
+ x[sample(n, size = na_prob * n)] <- NA
+ dim(x) <- c(nrow, ncol)
+ x
+ }
> rmatrices <- function(scale = 10, seed = 1, ...) {
+ set.seed(seed)
+ data <- list()
+ data[[1]] <- rmatrix(nrow = scale * 1, ncol = scale * 1, ...)
+ data[[2]] <- rmatrix(nrow = scale * 10, ncol = scale * 10, ...)
+ data[[3]] <- rmatrix(nrow = scale * 100, ncol = scale * 1, ...)
+ data[[4]] <- t(data[[3]])
+ data[[5]] <- rmatrix(nrow = scale * 10, ncol = scale * 100, ...)
+ data[[6]] <- t(data[[5]])
+ names(data) <- sapply(data, FUN = function(x) paste(dim(x), collapse = "x"))
+ data
+ }
> data <- rmatrices(mode = "logical")
Results
10x10 matrix
> X <- data[["10x10"]]
> gc()
used (Mb) gc trigger (Mb) max used (Mb)
Ncells 5165349 275.9 8529671 455.6 8529671 455.6
Vcells 9403025 71.8 31876688 243.2 60562128 462.1
> colStats <- microbenchmark(colAlls = colAlls(X), `apply+all` = apply(X, MARGIN = 2L, FUN = all),
+ `colSums==n` = (colSums(X) == nrow(X)), unit = "ms")
> X <- t(X)
> gc()
used (Mb) gc trigger (Mb) max used (Mb)
Ncells 5156953 275.5 8529671 455.6 8529671 455.6
Vcells 9375641 71.6 31876688 243.2 60562128 462.1
> rowStats <- microbenchmark(rowAlls = rowAlls(X), `apply+all` = apply(X, MARGIN = 1L, FUN = all),
+ `rowSums==n` = (rowSums(X) == ncol(X)), unit = "ms")
Table: Benchmarking of colAlls(), apply+all() and colSums==n() on 10x10 data. The top panel shows times in milliseconds and the bottom panel shows relative times.
|
expr |
min |
lq |
mean |
median |
uq |
max |
1 |
colAlls |
0.003025 |
0.0032665 |
0.0040817 |
0.0041145 |
0.0043715 |
0.016839 |
3 |
colSums==n |
0.006548 |
0.0069500 |
0.0081327 |
0.0078040 |
0.0084610 |
0.041586 |
2 |
apply+all |
0.033170 |
0.0342715 |
0.0357029 |
0.0349825 |
0.0356310 |
0.084542 |
|
expr |
min |
lq |
mean |
median |
uq |
max |
1 |
colAlls |
1.000000 |
1.00000 |
1.000000 |
1.000000 |
1.000000 |
1.000000 |
3 |
colSums==n |
2.164628 |
2.12766 |
1.992471 |
1.896707 |
1.935491 |
2.469624 |
2 |
apply+all |
10.965289 |
10.49181 |
8.747059 |
8.502248 |
8.150749 |
5.020607 |
Table: Benchmarking of rowAlls(), apply+all() and rowSums==n() on 10x10 data (transposed). The top panel shows times in milliseconds and the bottom panel shows relative times.
|
expr |
min |
lq |
mean |
median |
uq |
max |
1 |
rowAlls |
0.002932 |
0.0032580 |
0.0040104 |
0.0040765 |
0.004377 |
0.015973 |
3 |
rowSums==n |
0.007202 |
0.0077930 |
0.0087189 |
0.0083520 |
0.008922 |
0.036586 |
2 |
apply+all |
0.032335 |
0.0341245 |
0.0354270 |
0.0346300 |
0.035332 |
0.080404 |
|
expr |
min |
lq |
mean |
median |
uq |
max |
1 |
rowAlls |
1.000000 |
1.000000 |
1.000000 |
1.000000 |
1.000000 |
1.000000 |
3 |
rowSums==n |
2.456344 |
2.391958 |
2.174094 |
2.048816 |
2.038382 |
2.290490 |
2 |
apply+all |
11.028308 |
10.474064 |
8.833892 |
8.495033 |
8.072196 |
5.033744 |
Figure: Benchmarking of colAlls(), apply+all() and colSums==n() on 10x10 data as well as rowAlls(), apply+all() and rowSums==n() on the same data transposed. Outliers are displayed as crosses. Times are in milliseconds.
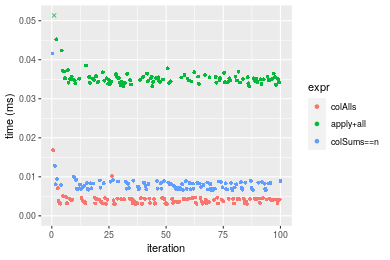
Table: Benchmarking of colAlls() and rowAlls() on 10x10 data (original and transposed). The top panel shows times in milliseconds and the bottom panel shows relative times.
|
expr |
min |
lq |
mean |
median |
uq |
max |
2 |
rowAlls |
2.932 |
3.2580 |
4.01035 |
4.0765 |
4.3770 |
15.973 |
1 |
colAlls |
3.025 |
3.2665 |
4.08170 |
4.1145 |
4.3715 |
16.839 |
|
expr |
min |
lq |
mean |
median |
uq |
max |
2 |
rowAlls |
1.000000 |
1.000000 |
1.000000 |
1.000000 |
1.0000000 |
1.000000 |
1 |
colAlls |
1.031719 |
1.002609 |
1.017791 |
1.009322 |
0.9987434 |
1.054216 |
Figure: Benchmarking of colAlls() and rowAlls() on 10x10 data (original and transposed). Outliers are displayed as crosses. Times are in milliseconds.
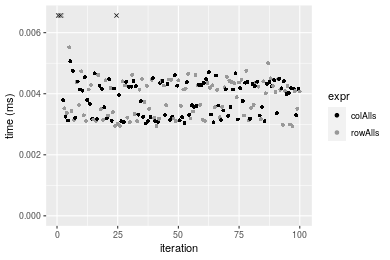
100x100 matrix
> X <- data[["100x100"]]
> gc()
used (Mb) gc trigger (Mb) max used (Mb)
Ncells 5155534 275.4 8529671 455.6 8529671 455.6
Vcells 9181870 70.1 31876688 243.2 60562128 462.1
> colStats <- microbenchmark(colAlls = colAlls(X), `apply+all` = apply(X, MARGIN = 2L, FUN = all),
+ `colSums==n` = (colSums(X) == nrow(X)), unit = "ms")
> X <- t(X)
> gc()
used (Mb) gc trigger (Mb) max used (Mb)
Ncells 5155510 275.4 8529671 455.6 8529671 455.6
Vcells 9186883 70.1 31876688 243.2 60562128 462.1
> rowStats <- microbenchmark(rowAlls = rowAlls(X), `apply+all` = apply(X, MARGIN = 1L, FUN = all),
+ `rowSums==n` = (rowSums(X) == ncol(X)), unit = "ms")
Table: Benchmarking of colAlls(), apply+all() and colSums==n() on 100x100 data. The top panel shows times in milliseconds and the bottom panel shows relative times.
|
expr |
min |
lq |
mean |
median |
uq |
max |
1 |
colAlls |
0.003316 |
0.0040280 |
0.0050496 |
0.0047005 |
0.0052810 |
0.023880 |
3 |
colSums==n |
0.015972 |
0.0174195 |
0.0194266 |
0.0187190 |
0.0206390 |
0.046336 |
2 |
apply+all |
0.194263 |
0.1984080 |
0.2214525 |
0.2145185 |
0.2345875 |
0.329061 |
|
expr |
min |
lq |
mean |
median |
uq |
max |
1 |
colAlls |
1.000000 |
1.000000 |
1.000000 |
1.000000 |
1.000000 |
1.000000 |
3 |
colSums==n |
4.816647 |
4.324603 |
3.847191 |
3.982342 |
3.908161 |
1.940368 |
2 |
apply+all |
58.583534 |
49.257200 |
43.855795 |
45.637379 |
44.421038 |
13.779774 |
Table: Benchmarking of rowAlls(), apply+all() and rowSums==n() on 100x100 data (transposed). The top panel shows times in milliseconds and the bottom panel shows relative times.
|
expr |
min |
lq |
mean |
median |
uq |
max |
1 |
rowAlls |
0.007901 |
0.0091755 |
0.0104472 |
0.0099110 |
0.0111075 |
0.026171 |
3 |
rowSums==n |
0.045504 |
0.0479895 |
0.0529970 |
0.0502815 |
0.0571440 |
0.081814 |
2 |
apply+all |
0.189569 |
0.1997645 |
0.2229071 |
0.2135790 |
0.2412965 |
0.329688 |
|
expr |
min |
lq |
mean |
median |
uq |
max |
1 |
rowAlls |
1.000000 |
1.000000 |
1.000000 |
1.000000 |
1.000000 |
1.000000 |
3 |
rowSums==n |
5.759271 |
5.230178 |
5.072857 |
5.073302 |
5.144632 |
3.126132 |
2 |
apply+all |
23.993039 |
21.771511 |
21.336619 |
21.549692 |
21.723745 |
12.597455 |
Figure: Benchmarking of colAlls(), apply+all() and colSums==n() on 100x100 data as well as rowAlls(), apply+all() and rowSums==n() on the same data transposed. Outliers are displayed as crosses. Times are in milliseconds.
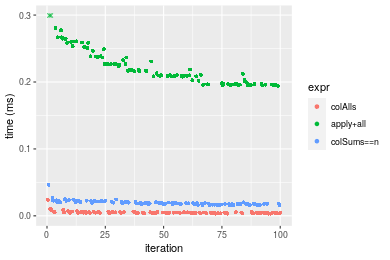
Table: Benchmarking of colAlls() and rowAlls() on 100x100 data (original and transposed). The top panel shows times in milliseconds and the bottom panel shows relative times.
|
expr |
min |
lq |
mean |
median |
uq |
max |
1 |
colAlls |
3.316 |
4.0280 |
5.04956 |
4.7005 |
5.2810 |
23.880 |
2 |
rowAlls |
7.901 |
9.1755 |
10.44716 |
9.9110 |
11.1075 |
26.171 |
|
expr |
min |
lq |
mean |
median |
uq |
max |
1 |
colAlls |
1.00000 |
1.000000 |
1.000000 |
1.000000 |
1.000000 |
1.000000 |
2 |
rowAlls |
2.38269 |
2.277929 |
2.068925 |
2.108499 |
2.103295 |
1.095938 |
Figure: Benchmarking of colAlls() and rowAlls() on 100x100 data (original and transposed). Outliers are displayed as crosses. Times are in milliseconds.
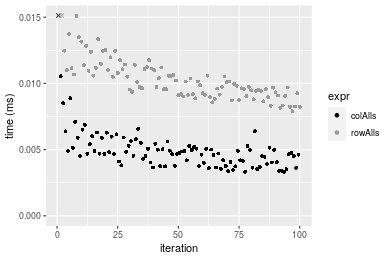
1000x10 matrix
> X <- data[["1000x10"]]
> gc()
used (Mb) gc trigger (Mb) max used (Mb)
Ncells 5156266 275.4 8529671 455.6 8529671 455.6
Vcells 9185359 70.1 31876688 243.2 60562128 462.1
> colStats <- microbenchmark(colAlls = colAlls(X), `apply+all` = apply(X, MARGIN = 2L, FUN = all),
+ `colSums==n` = (colSums(X) == nrow(X)), unit = "ms")
> X <- t(X)
> gc()
used (Mb) gc trigger (Mb) max used (Mb)
Ncells 5156260 275.4 8529671 455.6 8529671 455.6
Vcells 9190402 70.2 31876688 243.2 60562128 462.1
> rowStats <- microbenchmark(rowAlls = rowAlls(X), `apply+all` = apply(X, MARGIN = 1L, FUN = all),
+ `rowSums==n` = (rowSums(X) == ncol(X)), unit = "ms")
Table: Benchmarking of colAlls(), apply+all() and colSums==n() on 1000x10 data. The top panel shows times in milliseconds and the bottom panel shows relative times.
|
expr |
min |
lq |
mean |
median |
uq |
max |
1 |
colAlls |
0.002794 |
0.0034840 |
0.0042300 |
0.0041140 |
0.0046325 |
0.016881 |
3 |
colSums==n |
0.017402 |
0.0195220 |
0.0204640 |
0.0201180 |
0.0213370 |
0.038035 |
2 |
apply+all |
0.110305 |
0.1125515 |
0.1233028 |
0.1228605 |
0.1288965 |
0.207250 |
|
expr |
min |
lq |
mean |
median |
uq |
max |
1 |
colAlls |
1.000000 |
1.00000 |
1.000000 |
1.000000 |
1.000000 |
1.000000 |
3 |
colSums==n |
6.228346 |
5.60333 |
4.837852 |
4.890131 |
4.605936 |
2.253125 |
2 |
apply+all |
39.479241 |
32.30525 |
29.149800 |
29.864001 |
27.824393 |
12.277116 |
Table: Benchmarking of rowAlls(), apply+all() and rowSums==n() on 1000x10 data (transposed). The top panel shows times in milliseconds and the bottom panel shows relative times.
|
expr |
min |
lq |
mean |
median |
uq |
max |
1 |
rowAlls |
0.007931 |
0.0092260 |
0.0102171 |
0.0100165 |
0.0108175 |
0.023882 |
2 |
apply+all |
0.096265 |
0.1006470 |
0.1124996 |
0.1095850 |
0.1262765 |
0.194379 |
3 |
rowSums==n |
0.168196 |
0.1744085 |
0.1890403 |
0.1860320 |
0.2027455 |
0.232226 |
|
expr |
min |
lq |
mean |
median |
uq |
max |
1 |
rowAlls |
1.00000 |
1.00000 |
1.00000 |
1.00000 |
1.00000 |
1.000000 |
2 |
apply+all |
12.13781 |
10.90906 |
11.01093 |
10.94045 |
11.67335 |
8.139143 |
3 |
rowSums==n |
21.20741 |
18.90402 |
18.50238 |
18.57256 |
18.74236 |
9.723892 |
Figure: Benchmarking of colAlls(), apply+all() and colSums==n() on 1000x10 data as well as rowAlls(), apply+all() and rowSums==n() on the same data transposed. Outliers are displayed as crosses. Times are in milliseconds.
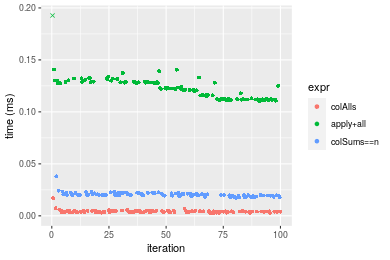
Table: Benchmarking of colAlls() and rowAlls() on 1000x10 data (original and transposed). The top panel shows times in milliseconds and the bottom panel shows relative times.
|
expr |
min |
lq |
mean |
median |
uq |
max |
1 |
colAlls |
2.794 |
3.484 |
4.22997 |
4.1140 |
4.6325 |
16.881 |
2 |
rowAlls |
7.931 |
9.226 |
10.21708 |
10.0165 |
10.8175 |
23.882 |
|
expr |
min |
lq |
mean |
median |
uq |
max |
1 |
colAlls |
1.000000 |
1.000000 |
1.000000 |
1.000000 |
1.000000 |
1.000000 |
2 |
rowAlls |
2.838583 |
2.648106 |
2.415402 |
2.434735 |
2.335132 |
1.414727 |
Figure: Benchmarking of colAlls() and rowAlls() on 1000x10 data (original and transposed). Outliers are displayed as crosses. Times are in milliseconds.
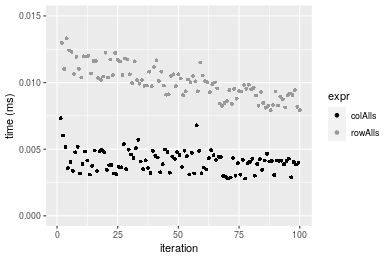
10x1000 matrix
> X <- data[["10x1000"]]
> gc()
used (Mb) gc trigger (Mb) max used (Mb)
Ncells 5156499 275.4 8529671 455.6 8529671 455.6
Vcells 9186143 70.1 31876688 243.2 60562128 462.1
> colStats <- microbenchmark(colAlls = colAlls(X), `apply+all` = apply(X, MARGIN = 2L, FUN = all),
+ `colSums==n` = (colSums(X) == nrow(X)), unit = "ms")
> X <- t(X)
> gc()
used (Mb) gc trigger (Mb) max used (Mb)
Ncells 5156475 275.4 8529671 455.6 8529671 455.6
Vcells 9191156 70.2 31876688 243.2 60562128 462.1
> rowStats <- microbenchmark(rowAlls = rowAlls(X), `apply+all` = apply(X, MARGIN = 1L, FUN = all),
+ `rowSums==n` = (rowSums(X) == ncol(X)), unit = "ms")
Table: Benchmarking of colAlls(), apply+all() and colSums==n() on 10x1000 data. The top panel shows times in milliseconds and the bottom panel shows relative times.
|
expr |
min |
lq |
mean |
median |
uq |
max |
3 |
colSums==n |
0.014091 |
0.0152290 |
0.0170517 |
0.0161795 |
0.0178900 |
0.037235 |
1 |
colAlls |
0.014461 |
0.0161340 |
0.0179866 |
0.0170280 |
0.0182545 |
0.039181 |
2 |
apply+all |
0.861692 |
0.9203145 |
1.0139202 |
0.9529525 |
1.0794300 |
1.434210 |
|
expr |
min |
lq |
mean |
median |
uq |
max |
3 |
colSums==n |
1.000000 |
1.000000 |
1.000000 |
1.000000 |
1.000000 |
1.000000 |
1 |
colAlls |
1.026258 |
1.059426 |
1.054825 |
1.052443 |
1.020374 |
1.052263 |
2 |
apply+all |
61.151941 |
60.431709 |
59.461428 |
58.898761 |
60.337060 |
38.517792 |
Table: Benchmarking of rowAlls(), apply+all() and rowSums==n() on 10x1000 data (transposed). The top panel shows times in milliseconds and the bottom panel shows relative times.
|
expr |
min |
lq |
mean |
median |
uq |
max |
1 |
rowAlls |
0.018529 |
0.0234715 |
0.0265038 |
0.0254230 |
0.0273330 |
0.066533 |
3 |
rowSums==n |
0.029118 |
0.0315485 |
0.0358700 |
0.0335410 |
0.0373885 |
0.069098 |
2 |
apply+all |
0.873644 |
0.9240285 |
1.0197045 |
0.9694025 |
1.0901125 |
1.423477 |
|
expr |
min |
lq |
mean |
median |
uq |
max |
1 |
rowAlls |
1.000000 |
1.000000 |
1.000000 |
1.000000 |
1.000000 |
1.000000 |
3 |
rowSums==n |
1.571482 |
1.344119 |
1.353392 |
1.319317 |
1.367889 |
1.038552 |
2 |
apply+all |
47.150089 |
39.368106 |
38.473955 |
38.130925 |
39.882651 |
21.395052 |
Figure: Benchmarking of colAlls(), apply+all() and colSums==n() on 10x1000 data as well as rowAlls(), apply+all() and rowSums==n() on the same data transposed. Outliers are displayed as crosses. Times are in milliseconds.
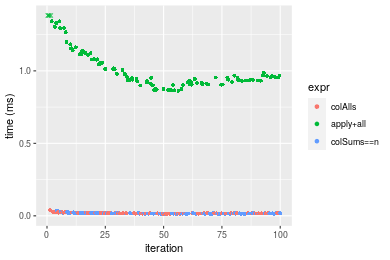
Table: Benchmarking of colAlls() and rowAlls() on 10x1000 data (original and transposed). The top panel shows times in milliseconds and the bottom panel shows relative times.
|
expr |
min |
lq |
mean |
median |
uq |
max |
1 |
colAlls |
14.461 |
16.1340 |
17.98660 |
17.028 |
18.2545 |
39.181 |
2 |
rowAlls |
18.529 |
23.4715 |
26.50376 |
25.423 |
27.3330 |
66.533 |
|
expr |
min |
lq |
mean |
median |
uq |
max |
1 |
colAlls |
1.000000 |
1.000000 |
1.000000 |
1.000000 |
1.000000 |
1.000000 |
2 |
rowAlls |
1.281308 |
1.454785 |
1.473528 |
1.493011 |
1.497329 |
1.698093 |
Figure: Benchmarking of colAlls() and rowAlls() on 10x1000 data (original and transposed). Outliers are displayed as crosses. Times are in milliseconds.
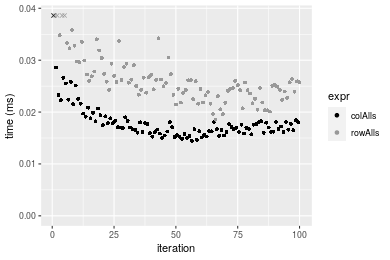
100x1000 matrix
> X <- data[["100x1000"]]
> gc()
used (Mb) gc trigger (Mb) max used (Mb)
Ncells 5156690 275.4 8529671 455.6 8529671 455.6
Vcells 9186633 70.1 31876688 243.2 60562128 462.1
> colStats <- microbenchmark(colAlls = colAlls(X), `apply+all` = apply(X, MARGIN = 2L, FUN = all),
+ `colSums==n` = (colSums(X) == nrow(X)), unit = "ms")
> X <- t(X)
> gc()
used (Mb) gc trigger (Mb) max used (Mb)
Ncells 5156678 275.4 8529671 455.6 8529671 455.6
Vcells 9236666 70.5 31876688 243.2 60562128 462.1
> rowStats <- microbenchmark(rowAlls = rowAlls(X), `apply+all` = apply(X, MARGIN = 1L, FUN = all),
+ `rowSums==n` = (rowSums(X) == ncol(X)), unit = "ms")
Table: Benchmarking of colAlls(), apply+all() and colSums==n() on 100x1000 data. The top panel shows times in milliseconds and the bottom panel shows relative times.
|
expr |
min |
lq |
mean |
median |
uq |
max |
1 |
colAlls |
0.016503 |
0.018137 |
0.0200962 |
0.0194905 |
0.0206635 |
0.041491 |
3 |
colSums==n |
0.081474 |
0.088031 |
0.0951526 |
0.0916770 |
0.0998565 |
0.153938 |
2 |
apply+all |
1.367004 |
1.506773 |
1.5948512 |
1.5471875 |
1.6063595 |
2.526367 |
|
expr |
min |
lq |
mean |
median |
uq |
max |
1 |
colAlls |
1.000000 |
1.000000 |
1.000000 |
1.000000 |
1.000000 |
1.000000 |
3 |
colSums==n |
4.936921 |
4.853669 |
4.734855 |
4.703676 |
4.832507 |
3.710154 |
2 |
apply+all |
82.833667 |
83.077301 |
79.360873 |
79.381622 |
77.738984 |
60.889518 |
Table: Benchmarking of rowAlls(), apply+all() and rowSums==n() on 100x1000 data (transposed). The top panel shows times in milliseconds and the bottom panel shows relative times.
|
expr |
min |
lq |
mean |
median |
uq |
max |
1 |
rowAlls |
0.042957 |
0.0492940 |
0.0539950 |
0.0518085 |
0.0559535 |
0.109683 |
3 |
rowSums==n |
0.232280 |
0.2505305 |
0.2703589 |
0.2580990 |
0.2763755 |
0.399680 |
2 |
apply+all |
1.364724 |
1.4836375 |
1.5768114 |
1.5557460 |
1.5938430 |
2.508230 |
|
expr |
min |
lq |
mean |
median |
uq |
max |
1 |
rowAlls |
1.000000 |
1.000000 |
1.000000 |
1.000000 |
1.000000 |
1.000000 |
3 |
rowSums==n |
5.407268 |
5.082373 |
5.007113 |
4.981789 |
4.939378 |
3.643956 |
2 |
apply+all |
31.769537 |
30.097730 |
29.202932 |
30.028779 |
28.485135 |
22.867992 |
Figure: Benchmarking of colAlls(), apply+all() and colSums==n() on 100x1000 data as well as rowAlls(), apply+all() and rowSums==n() on the same data transposed. Outliers are displayed as crosses. Times are in milliseconds.
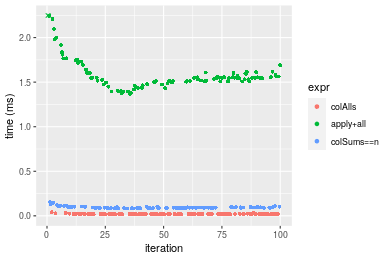
Table: Benchmarking of colAlls() and rowAlls() on 100x1000 data (original and transposed). The top panel shows times in milliseconds and the bottom panel shows relative times.
|
expr |
min |
lq |
mean |
median |
uq |
max |
1 |
colAlls |
16.503 |
18.137 |
20.09619 |
19.4905 |
20.6635 |
41.491 |
2 |
rowAlls |
42.957 |
49.294 |
53.99497 |
51.8085 |
55.9535 |
109.683 |
|
expr |
min |
lq |
mean |
median |
uq |
max |
1 |
colAlls |
1.000000 |
1.000000 |
1.000000 |
1.000000 |
1.000000 |
1.000000 |
2 |
rowAlls |
2.602981 |
2.717869 |
2.686826 |
2.658141 |
2.707842 |
2.643537 |
Figure: Benchmarking of colAlls() and rowAlls() on 100x1000 data (original and transposed). Outliers are displayed as crosses. Times are in milliseconds.
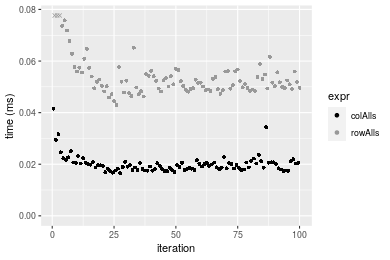
1000x100 matrix
> X <- data[["1000x100"]]
> gc()
used (Mb) gc trigger (Mb) max used (Mb)
Ncells 5156896 275.5 8529671 455.6 8529671 455.6
Vcells 9187285 70.1 31876688 243.2 60562128 462.1
> colStats <- microbenchmark(colAlls = colAlls(X), `apply+all` = apply(X, MARGIN = 2L, FUN = all),
+ `colSums==n` = (colSums(X) == nrow(X)), unit = "ms")
> X <- t(X)
> gc()
used (Mb) gc trigger (Mb) max used (Mb)
Ncells 5156884 275.5 8529671 455.6 8529671 455.6
Vcells 9237318 70.5 31876688 243.2 60562128 462.1
> rowStats <- microbenchmark(rowAlls = rowAlls(X), `apply+all` = apply(X, MARGIN = 1L, FUN = all),
+ `rowSums==n` = (rowSums(X) == ncol(X)), unit = "ms")
Table: Benchmarking of colAlls(), apply+all() and colSums==n() on 1000x100 data. The top panel shows times in milliseconds and the bottom panel shows relative times.
|
expr |
min |
lq |
mean |
median |
uq |
max |
1 |
colAlls |
0.003105 |
0.004097 |
0.0055423 |
0.0049490 |
0.0060700 |
0.026122 |
3 |
colSums==n |
0.084499 |
0.088823 |
0.1018254 |
0.0935520 |
0.1094800 |
0.173184 |
2 |
apply+all |
0.635825 |
0.671165 |
0.7549770 |
0.7020235 |
0.8062205 |
1.114587 |
|
expr |
min |
lq |
mean |
median |
uq |
max |
1 |
colAlls |
1.00000 |
1.00000 |
1.00000 |
1.00000 |
1.00000 |
1.000000 |
3 |
colSums==n |
27.21385 |
21.68001 |
18.37235 |
18.90321 |
18.03624 |
6.629814 |
2 |
apply+all |
204.77456 |
163.81865 |
136.22040 |
141.85159 |
132.82051 |
42.668517 |
Table: Benchmarking of rowAlls(), apply+all() and rowSums==n() on 1000x100 data (transposed). The top panel shows times in milliseconds and the bottom panel shows relative times.
|
expr |
min |
lq |
mean |
median |
uq |
max |
1 |
rowAlls |
0.042237 |
0.0455145 |
0.0514634 |
0.0478220 |
0.0536615 |
0.104833 |
3 |
rowSums==n |
0.324287 |
0.3433275 |
0.3810341 |
0.3621710 |
0.4081510 |
0.542330 |
2 |
apply+all |
0.636731 |
0.6672355 |
0.7344137 |
0.6960165 |
0.7531320 |
1.165268 |
|
expr |
min |
lq |
mean |
median |
uq |
max |
1 |
rowAlls |
1.000000 |
1.000000 |
1.00000 |
1.000000 |
1.00000 |
1.000000 |
3 |
rowSums==n |
7.677794 |
7.543255 |
7.40398 |
7.573314 |
7.60603 |
5.173276 |
2 |
apply+all |
15.075195 |
14.659845 |
14.27060 |
14.554316 |
14.03487 |
11.115469 |
Figure: Benchmarking of colAlls(), apply+all() and colSums==n() on 1000x100 data as well as rowAlls(), apply+all() and rowSums==n() on the same data transposed. Outliers are displayed as crosses. Times are in milliseconds.
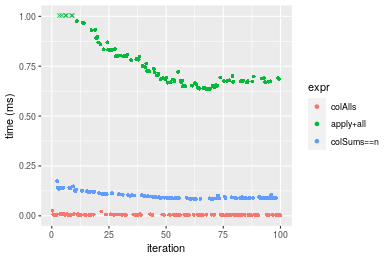
Table: Benchmarking of colAlls() and rowAlls() on 1000x100 data (original and transposed). The top panel shows times in milliseconds and the bottom panel shows relative times.
|
expr |
min |
lq |
mean |
median |
uq |
max |
1 |
colAlls |
3.105 |
4.0970 |
5.54232 |
4.949 |
6.0700 |
26.122 |
2 |
rowAlls |
42.237 |
45.5145 |
51.46341 |
47.822 |
53.6615 |
104.833 |
|
expr |
min |
lq |
mean |
median |
uq |
max |
1 |
colAlls |
1.0000 |
1.00000 |
1.000000 |
1.000000 |
1.000000 |
1.000000 |
2 |
rowAlls |
13.6029 |
11.10923 |
9.285536 |
9.662962 |
8.840445 |
4.013207 |
Figure: Benchmarking of colAlls() and rowAlls() on 1000x100 data (original and transposed). Outliers are displayed as crosses. Times are in milliseconds.
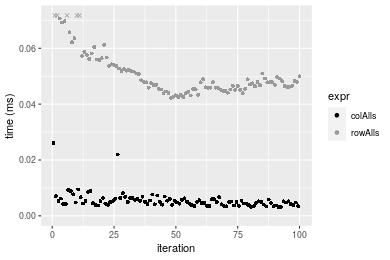
Appendix
R version 4.1.1 Patched (2021-08-10 r80727)
Platform: x86_64-pc-linux-gnu (64-bit)
Running under: Ubuntu 18.04.5 LTS
Matrix products: default
BLAS: /home/hb/software/R-devel/R-4-1-branch/lib/R/lib/libRblas.so
LAPACK: /home/hb/software/R-devel/R-4-1-branch/lib/R/lib/libRlapack.so
locale:
[1] LC_CTYPE=en_US.UTF-8 LC_NUMERIC=C
[3] LC_TIME=en_US.UTF-8 LC_COLLATE=en_US.UTF-8
[5] LC_MONETARY=en_US.UTF-8 LC_MESSAGES=en_US.UTF-8
[7] LC_PAPER=en_US.UTF-8 LC_NAME=C
[9] LC_ADDRESS=C LC_TELEPHONE=C
[11] LC_MEASUREMENT=en_US.UTF-8 LC_IDENTIFICATION=C
attached base packages:
[1] stats graphics grDevices utils datasets methods base
other attached packages:
[1] microbenchmark_1.4-7 matrixStats_0.60.1 ggplot2_3.3.5
[4] knitr_1.33 R.devices_2.17.0 R.utils_2.10.1
[7] R.oo_1.24.0 R.methodsS3_1.8.1-9001 history_0.0.1-9000
loaded via a namespace (and not attached):
[1] Biobase_2.52.0 httr_1.4.2 splines_4.1.1
[4] bit64_4.0.5 network_1.17.1 assertthat_0.2.1
[7] highr_0.9 stats4_4.1.1 blob_1.2.2
[10] GenomeInfoDbData_1.2.6 robustbase_0.93-8 pillar_1.6.2
[13] RSQLite_2.2.8 lattice_0.20-44 glue_1.4.2
[16] digest_0.6.27 XVector_0.32.0 colorspace_2.0-2
[19] Matrix_1.3-4 XML_3.99-0.7 pkgconfig_2.0.3
[22] zlibbioc_1.38.0 genefilter_1.74.0 purrr_0.3.4
[25] ergm_4.1.2 xtable_1.8-4 scales_1.1.1
[28] tibble_3.1.4 annotate_1.70.0 KEGGREST_1.32.0
[31] farver_2.1.0 generics_0.1.0 IRanges_2.26.0
[34] ellipsis_0.3.2 cachem_1.0.6 withr_2.4.2
[37] BiocGenerics_0.38.0 mime_0.11 survival_3.2-13
[40] magrittr_2.0.1 crayon_1.4.1 statnet.common_4.5.0
[43] memoise_2.0.0 laeken_0.5.1 fansi_0.5.0
[46] R.cache_0.15.0 MASS_7.3-54 R.rsp_0.44.0
[49] progressr_0.8.0 tools_4.1.1 lifecycle_1.0.0
[52] S4Vectors_0.30.0 trust_0.1-8 munsell_0.5.0
[55] tabby_0.0.1-9001 AnnotationDbi_1.54.1 Biostrings_2.60.2
[58] compiler_4.1.1 GenomeInfoDb_1.28.1 rlang_0.4.11
[61] grid_4.1.1 RCurl_1.98-1.4 cwhmisc_6.6
[64] rappdirs_0.3.3 startup_0.15.0 labeling_0.4.2
[67] bitops_1.0-7 base64enc_0.1-3 boot_1.3-28
[70] gtable_0.3.0 DBI_1.1.1 markdown_1.1
[73] R6_2.5.1 lpSolveAPI_5.5.2.0-17.7 rle_0.9.2
[76] dplyr_1.0.7 fastmap_1.1.0 bit_4.0.4
[79] utf8_1.2.2 parallel_4.1.1 Rcpp_1.0.7
[82] vctrs_0.3.8 png_0.1-7 DEoptimR_1.0-9
[85] tidyselect_1.1.1 xfun_0.25 coda_0.19-4
Total processing time was 11.79 secs.
Reproducibility
To reproduce this report, do:
html <- matrixStats:::benchmark('colAlls')
Copyright Henrik Bengtsson. Last updated on 2021-08-25 18:49:49 (+0200 UTC). Powered by RSP.