This report benchmark the performance of colWeightedMedians() and rowWeightedMedians() against alternative methods.
Alternative methods
- apply() + weightedMedian()
Data
> rmatrix <- function(nrow, ncol, mode = c("logical", "double", "integer", "index"), range = c(-100,
+ +100), na_prob = 0) {
+ mode <- match.arg(mode)
+ n <- nrow * ncol
+ if (mode == "logical") {
+ x <- sample(c(FALSE, TRUE), size = n, replace = TRUE)
+ } else if (mode == "index") {
+ x <- seq_len(n)
+ mode <- "integer"
+ } else {
+ x <- runif(n, min = range[1], max = range[2])
+ }
+ storage.mode(x) <- mode
+ if (na_prob > 0)
+ x[sample(n, size = na_prob * n)] <- NA
+ dim(x) <- c(nrow, ncol)
+ x
+ }
> rmatrices <- function(scale = 10, seed = 1, ...) {
+ set.seed(seed)
+ data <- list()
+ data[[1]] <- rmatrix(nrow = scale * 1, ncol = scale * 1, ...)
+ data[[2]] <- rmatrix(nrow = scale * 10, ncol = scale * 10, ...)
+ data[[3]] <- rmatrix(nrow = scale * 100, ncol = scale * 1, ...)
+ data[[4]] <- t(data[[3]])
+ data[[5]] <- rmatrix(nrow = scale * 10, ncol = scale * 100, ...)
+ data[[6]] <- t(data[[5]])
+ names(data) <- sapply(data, FUN = function(x) paste(dim(x), collapse = "x"))
+ data
+ }
> data <- rmatrices(mode = "double")
Results
10x10 matrix
> X <- data[["10x10"]]
> w <- runif(nrow(X))
> gc()
used (Mb) gc trigger (Mb) max used (Mb)
Ncells 5338373 285.1 7916910 422.9 7916910 422.9
Vcells 10912022 83.3 33191153 253.3 53339345 407.0
> colStats <- microbenchmark(colWeightedMedians = colWeightedMedians(X, w = w, na.rm = FALSE), `apply+weigthedMedian` = apply(X,
+ MARGIN = 2L, FUN = weightedMedian, w = w, na.rm = FALSE), unit = "ms")
> X <- t(X)
> gc()
used (Mb) gc trigger (Mb) max used (Mb)
Ncells 5337341 285.1 7916910 422.9 7916910 422.9
Vcells 10909019 83.3 33191153 253.3 53339345 407.0
> rowStats <- microbenchmark(rowWeightedMedians = rowWeightedMedians(X, w = w, na.rm = FALSE), `apply+weigthedMedian` = apply(X,
+ MARGIN = 1L, FUN = weightedMedian, w = w, na.rm = FALSE), unit = "ms")
Table: Benchmarking of colWeightedMedians() and apply+weigthedMedian() on 10x10 data. The top panel shows times in milliseconds and the bottom panel shows relative times.
|
expr |
min |
lq |
mean |
median |
uq |
max |
2 |
apply+weigthedMedian |
0.058618 |
0.0649075 |
0.066852 |
0.0656505 |
0.0663995 |
0.155059 |
1 |
colWeightedMedians |
0.069945 |
0.0793620 |
0.080739 |
0.0805175 |
0.0817440 |
0.114797 |
|
expr |
min |
lq |
mean |
median |
uq |
max |
2 |
apply+weigthedMedian |
1.000000 |
1.000000 |
1.000000 |
1.000000 |
1.000000 |
1.000000 |
1 |
colWeightedMedians |
1.193234 |
1.222694 |
1.207728 |
1.226457 |
1.231094 |
0.740344 |
Table: Benchmarking of rowWeightedMedians() and apply+weigthedMedian() on 10x10 data (transposed). The top panel shows times in milliseconds and the bottom panel shows relative times.
|
expr |
min |
lq |
mean |
median |
uq |
max |
2 |
apply+weigthedMedian |
0.057798 |
0.0626545 |
0.0657444 |
0.0651835 |
0.0657595 |
0.149053 |
1 |
rowWeightedMedians |
0.071850 |
0.0772715 |
0.0803987 |
0.0796075 |
0.0811340 |
0.113690 |
|
expr |
min |
lq |
mean |
median |
uq |
max |
2 |
apply+weigthedMedian |
1.000000 |
1.000000 |
1.000000 |
1.000000 |
1.000000 |
1.0000000 |
1 |
rowWeightedMedians |
1.243123 |
1.233295 |
1.222897 |
1.221283 |
1.233799 |
0.7627488 |
Figure: Benchmarking of colWeightedMedians() and apply+weigthedMedian() on 10x10 data as well as rowWeightedMedians() and apply+weigthedMedian() on the same data transposed. Outliers are displayed as crosses. Times are in milliseconds.
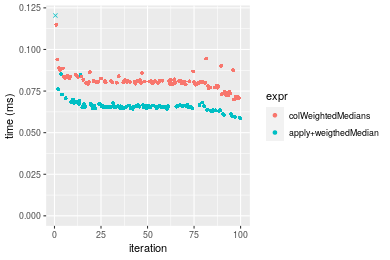
Table: Benchmarking of colWeightedMedians() and rowWeightedMedians() on 10x10 data (original and transposed). The top panel shows times in milliseconds and the bottom panel shows relative times.
|
expr |
min |
lq |
mean |
median |
uq |
max |
2 |
rowWeightedMedians |
71.850 |
77.2715 |
80.39870 |
79.6075 |
81.134 |
113.690 |
1 |
colWeightedMedians |
69.945 |
79.3620 |
80.73899 |
80.5175 |
81.744 |
114.797 |
|
expr |
min |
lq |
mean |
median |
uq |
max |
2 |
rowWeightedMedians |
1.0000000 |
1.000000 |
1.000000 |
1.000000 |
1.000000 |
1.000000 |
1 |
colWeightedMedians |
0.9734864 |
1.027054 |
1.004233 |
1.011431 |
1.007518 |
1.009737 |
Figure: Benchmarking of colWeightedMedians() and rowWeightedMedians() on 10x10 data (original and transposed). Outliers are displayed as crosses. Times are in milliseconds.
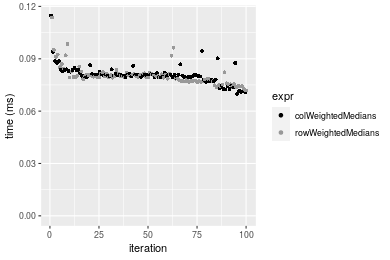
100x100 matrix
> X <- data[["100x100"]]
> w <- runif(nrow(X))
> gc()
used (Mb) gc trigger (Mb) max used (Mb)
Ncells 5335902 285.0 7916910 422.9 7916910 422.9
Vcells 10523851 80.3 33191153 253.3 53339345 407.0
> colStats <- microbenchmark(colWeightedMedians = colWeightedMedians(X, w = w, na.rm = FALSE), `apply+weigthedMedian` = apply(X,
+ MARGIN = 2L, FUN = weightedMedian, w = w, na.rm = FALSE), unit = "ms")
> X <- t(X)
> gc()
used (Mb) gc trigger (Mb) max used (Mb)
Ncells 5335896 285.0 7916910 422.9 7916910 422.9
Vcells 10533894 80.4 33191153 253.3 53339345 407.0
> rowStats <- microbenchmark(rowWeightedMedians = rowWeightedMedians(X, w = w, na.rm = FALSE), `apply+weigthedMedian` = apply(X,
+ MARGIN = 1L, FUN = weightedMedian, w = w, na.rm = FALSE), unit = "ms")
Table: Benchmarking of colWeightedMedians() and apply+weigthedMedian() on 100x100 data. The top panel shows times in milliseconds and the bottom panel shows relative times.
|
expr |
min |
lq |
mean |
median |
uq |
max |
2 |
apply+weigthedMedian |
0.666541 |
0.6816655 |
0.7668511 |
0.7063770 |
0.8279125 |
1.268180 |
1 |
colWeightedMedians |
0.723570 |
0.7469600 |
0.8814077 |
0.8105625 |
0.9538670 |
1.617107 |
|
expr |
min |
lq |
mean |
median |
uq |
max |
2 |
apply+weigthedMedian |
1.00000 |
1.000000 |
1.000000 |
1.000000 |
1.000000 |
1.00000 |
1 |
colWeightedMedians |
1.08556 |
1.095787 |
1.149386 |
1.147493 |
1.152135 |
1.27514 |
Table: Benchmarking of rowWeightedMedians() and apply+weigthedMedian() on 100x100 data (transposed). The top panel shows times in milliseconds and the bottom panel shows relative times.
|
expr |
min |
lq |
mean |
median |
uq |
max |
2 |
apply+weigthedMedian |
0.662943 |
0.6722950 |
0.7338995 |
0.679953 |
0.737635 |
1.254176 |
1 |
rowWeightedMedians |
0.721027 |
0.7318395 |
0.8291074 |
0.743418 |
0.885740 |
1.276855 |
|
expr |
min |
lq |
mean |
median |
uq |
max |
2 |
apply+weigthedMedian |
1.000000 |
1.000000 |
1.000000 |
1.000000 |
1.000000 |
1.000000 |
1 |
rowWeightedMedians |
1.087615 |
1.088569 |
1.129729 |
1.093337 |
1.200784 |
1.018083 |
Figure: Benchmarking of colWeightedMedians() and apply+weigthedMedian() on 100x100 data as well as rowWeightedMedians() and apply+weigthedMedian() on the same data transposed. Outliers are displayed as crosses. Times are in milliseconds.
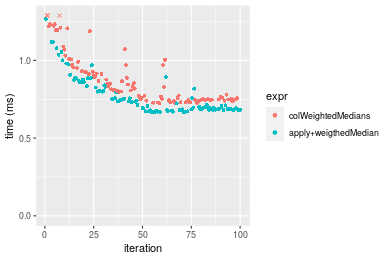
Table: Benchmarking of colWeightedMedians() and rowWeightedMedians() on 100x100 data (original and transposed). The top panel shows times in milliseconds and the bottom panel shows relative times.
|
expr |
min |
lq |
mean |
median |
uq |
max |
2 |
rowWeightedMedians |
721.027 |
731.8395 |
829.1074 |
743.4180 |
885.740 |
1276.855 |
1 |
colWeightedMedians |
723.570 |
746.9600 |
881.4077 |
810.5625 |
953.867 |
1617.107 |
|
expr |
min |
lq |
mean |
median |
uq |
max |
2 |
rowWeightedMedians |
1.000000 |
1.000000 |
1.00000 |
1.000000 |
1.000000 |
1.000000 |
1 |
colWeightedMedians |
1.003527 |
1.020661 |
1.06308 |
1.090319 |
1.076915 |
1.266477 |
Figure: Benchmarking of colWeightedMedians() and rowWeightedMedians() on 100x100 data (original and transposed). Outliers are displayed as crosses. Times are in milliseconds.
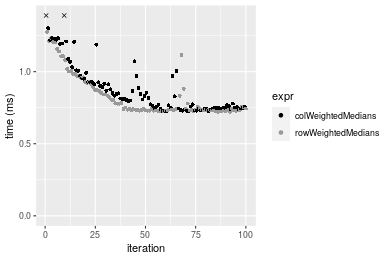
1000x10 matrix
> X <- data[["1000x10"]]
> w <- runif(nrow(X))
> gc()
used (Mb) gc trigger (Mb) max used (Mb)
Ncells 5336627 285.1 7916910 422.9 7916910 422.9
Vcells 10528265 80.4 33191153 253.3 53339345 407.0
> colStats <- microbenchmark(colWeightedMedians = colWeightedMedians(X, w = w, na.rm = FALSE), `apply+weigthedMedian` = apply(X,
+ MARGIN = 2L, FUN = weightedMedian, w = w, na.rm = FALSE), unit = "ms")
> X <- t(X)
> gc()
used (Mb) gc trigger (Mb) max used (Mb)
Ncells 5336615 285.1 7916910 422.9 7916910 422.9
Vcells 10538298 80.5 33191153 253.3 53339345 407.0
> rowStats <- microbenchmark(rowWeightedMedians = rowWeightedMedians(X, w = w, na.rm = FALSE), `apply+weigthedMedian` = apply(X,
+ MARGIN = 1L, FUN = weightedMedian, w = w, na.rm = FALSE), unit = "ms")
Table: Benchmarking of colWeightedMedians() and apply+weigthedMedian() on 1000x10 data. The top panel shows times in milliseconds and the bottom panel shows relative times.
|
expr |
min |
lq |
mean |
median |
uq |
max |
2 |
apply+weigthedMedian |
0.558770 |
0.6110875 |
0.6854559 |
0.684275 |
0.7321420 |
0.964007 |
1 |
colWeightedMedians |
0.574385 |
0.6210990 |
0.7070122 |
0.699900 |
0.7542415 |
1.130088 |
|
expr |
min |
lq |
mean |
median |
uq |
max |
2 |
apply+weigthedMedian |
1.000000 |
1.000000 |
1.000000 |
1.000000 |
1.000000 |
1.000000 |
1 |
colWeightedMedians |
1.027945 |
1.016383 |
1.031448 |
1.022834 |
1.030185 |
1.172282 |
Table: Benchmarking of rowWeightedMedians() and apply+weigthedMedian() on 1000x10 data (transposed). The top panel shows times in milliseconds and the bottom panel shows relative times.
|
expr |
min |
lq |
mean |
median |
uq |
max |
2 |
apply+weigthedMedian |
0.557595 |
0.5607195 |
0.6269260 |
0.5652330 |
0.6619825 |
0.937099 |
1 |
rowWeightedMedians |
0.575496 |
0.5814740 |
0.6520714 |
0.5866085 |
0.7192995 |
1.051900 |
|
expr |
min |
lq |
mean |
median |
uq |
max |
2 |
apply+weigthedMedian |
1.000000 |
1.000000 |
1.000000 |
1.000000 |
1.000000 |
1.000000 |
1 |
rowWeightedMedians |
1.032104 |
1.037014 |
1.040109 |
1.037817 |
1.086584 |
1.122507 |
Figure: Benchmarking of colWeightedMedians() and apply+weigthedMedian() on 1000x10 data as well as rowWeightedMedians() and apply+weigthedMedian() on the same data transposed. Outliers are displayed as crosses. Times are in milliseconds.
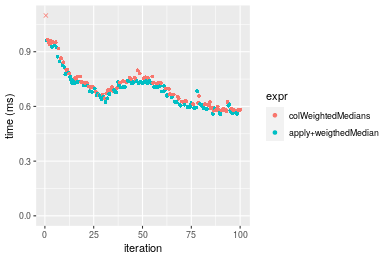
Table: Benchmarking of colWeightedMedians() and rowWeightedMedians() on 1000x10 data (original and transposed). The top panel shows times in milliseconds and the bottom panel shows relative times.
|
expr |
min |
lq |
mean |
median |
uq |
max |
2 |
rowWeightedMedians |
575.496 |
581.474 |
652.0714 |
586.6085 |
719.2995 |
1051.900 |
1 |
colWeightedMedians |
574.385 |
621.099 |
707.0122 |
699.9000 |
754.2415 |
1130.088 |
|
expr |
min |
lq |
mean |
median |
uq |
max |
2 |
rowWeightedMedians |
1.0000000 |
1.000000 |
1.000000 |
1.00000 |
1.000000 |
1.00000 |
1 |
colWeightedMedians |
0.9980695 |
1.068146 |
1.084256 |
1.19313 |
1.048578 |
1.07433 |
Figure: Benchmarking of colWeightedMedians() and rowWeightedMedians() on 1000x10 data (original and transposed). Outliers are displayed as crosses. Times are in milliseconds.
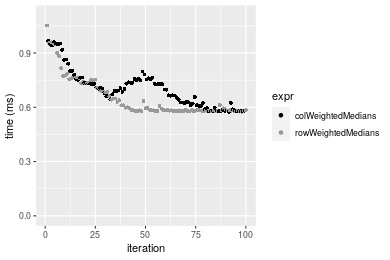
10x1000 matrix
> X <- data[["10x1000"]]
> w <- runif(nrow(X))
> gc()
used (Mb) gc trigger (Mb) max used (Mb)
Ncells 5336819 285.1 7916910 422.9 7916910 422.9
Vcells 10528074 80.4 33191153 253.3 53339345 407.0
> colStats <- microbenchmark(colWeightedMedians = colWeightedMedians(X, w = w, na.rm = FALSE), `apply+weigthedMedian` = apply(X,
+ MARGIN = 2L, FUN = weightedMedian, w = w, na.rm = FALSE), unit = "ms")
> X <- t(X)
> gc()
used (Mb) gc trigger (Mb) max used (Mb)
Ncells 5336813 285.1 7916910 422.9 7916910 422.9
Vcells 10538117 80.4 33191153 253.3 53339345 407.0
> rowStats <- microbenchmark(rowWeightedMedians = rowWeightedMedians(X, w = w, na.rm = FALSE), `apply+weigthedMedian` = apply(X,
+ MARGIN = 1L, FUN = weightedMedian, w = w, na.rm = FALSE), unit = "ms")
Table: Benchmarking of colWeightedMedians() and apply+weigthedMedian() on 10x1000 data. The top panel shows times in milliseconds and the bottom panel shows relative times.
|
expr |
min |
lq |
mean |
median |
uq |
max |
2 |
apply+weigthedMedian |
2.638999 |
2.811646 |
3.145791 |
2.922781 |
3.055063 |
9.457205 |
1 |
colWeightedMedians |
3.171310 |
3.395751 |
3.607023 |
3.513560 |
3.689402 |
5.259681 |
|
expr |
min |
lq |
mean |
median |
uq |
max |
2 |
apply+weigthedMedian |
1.000000 |
1.000000 |
1.000000 |
1.000000 |
1.000000 |
1.000000 |
1 |
colWeightedMedians |
1.201709 |
1.207745 |
1.146619 |
1.202129 |
1.207636 |
0.556156 |
Table: Benchmarking of rowWeightedMedians() and apply+weigthedMedian() on 10x1000 data (transposed). The top panel shows times in milliseconds and the bottom panel shows relative times.
|
expr |
min |
lq |
mean |
median |
uq |
max |
2 |
apply+weigthedMedian |
2.632101 |
2.873942 |
3.086753 |
2.957736 |
3.065488 |
9.281187 |
1 |
rowWeightedMedians |
3.181512 |
3.416350 |
3.716918 |
3.491299 |
3.641147 |
10.598747 |
|
expr |
min |
lq |
mean |
median |
uq |
max |
2 |
apply+weigthedMedian |
1.000000 |
1.000000 |
1.000000 |
1.000000 |
1.000000 |
1.00000 |
1 |
rowWeightedMedians |
1.208735 |
1.188733 |
1.204151 |
1.180396 |
1.187787 |
1.14196 |
Figure: Benchmarking of colWeightedMedians() and apply+weigthedMedian() on 10x1000 data as well as rowWeightedMedians() and apply+weigthedMedian() on the same data transposed. Outliers are displayed as crosses. Times are in milliseconds.
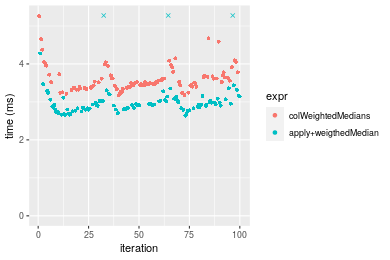
Table: Benchmarking of colWeightedMedians() and rowWeightedMedians() on 10x1000 data (original and transposed). The top panel shows times in milliseconds and the bottom panel shows relative times.
|
expr |
min |
lq |
mean |
median |
uq |
max |
2 |
rowWeightedMedians |
3.181512 |
3.416350 |
3.716918 |
3.491299 |
3.641147 |
10.598747 |
1 |
colWeightedMedians |
3.171310 |
3.395751 |
3.607023 |
3.513560 |
3.689402 |
5.259681 |
|
expr |
min |
lq |
mean |
median |
uq |
max |
2 |
rowWeightedMedians |
1.0000000 |
1.0000000 |
1.0000000 |
1.000000 |
1.000000 |
1.000000 |
1 |
colWeightedMedians |
0.9967933 |
0.9939703 |
0.9704339 |
1.006376 |
1.013253 |
0.496255 |
Figure: Benchmarking of colWeightedMedians() and rowWeightedMedians() on 10x1000 data (original and transposed). Outliers are displayed as crosses. Times are in milliseconds.
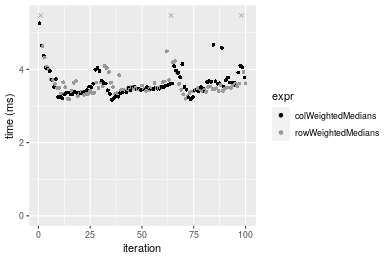
100x1000 matrix
> X <- data[["100x1000"]]
> w <- runif(nrow(X))
> gc()
used (Mb) gc trigger (Mb) max used (Mb)
Ncells 5337009 285.1 7916910 422.9 7916910 422.9
Vcells 10528669 80.4 33191153 253.3 53339345 407.0
> colStats <- microbenchmark(colWeightedMedians = colWeightedMedians(X, w = w, na.rm = FALSE), `apply+weigthedMedian` = apply(X,
+ MARGIN = 2L, FUN = weightedMedian, w = w, na.rm = FALSE), unit = "ms")
> X <- t(X)
> gc()
used (Mb) gc trigger (Mb) max used (Mb)
Ncells 5337003 285.1 7916910 422.9 7916910 422.9
Vcells 10628712 81.1 33191153 253.3 53339345 407.0
> rowStats <- microbenchmark(rowWeightedMedians = rowWeightedMedians(X, w = w, na.rm = FALSE), `apply+weigthedMedian` = apply(X,
+ MARGIN = 1L, FUN = weightedMedian, w = w, na.rm = FALSE), unit = "ms")
Table: Benchmarking of colWeightedMedians() and apply+weigthedMedian() on 100x1000 data. The top panel shows times in milliseconds and the bottom panel shows relative times.
|
expr |
min |
lq |
mean |
median |
uq |
max |
2 |
apply+weigthedMedian |
6.520031 |
6.851844 |
7.541683 |
7.009149 |
7.251044 |
24.63903 |
1 |
colWeightedMedians |
7.072074 |
7.387505 |
7.805397 |
7.489342 |
7.701308 |
24.78461 |
|
expr |
min |
lq |
mean |
median |
uq |
max |
2 |
apply+weigthedMedian |
1.000000 |
1.000000 |
1.000000 |
1.00000 |
1.000000 |
1.000000 |
1 |
colWeightedMedians |
1.084669 |
1.078178 |
1.034968 |
1.06851 |
1.062096 |
1.005908 |
Table: Benchmarking of rowWeightedMedians() and apply+weigthedMedian() on 100x1000 data (transposed). The top panel shows times in milliseconds and the bottom panel shows relative times.
|
expr |
min |
lq |
mean |
median |
uq |
max |
2 |
apply+weigthedMedian |
6.601629 |
6.941376 |
7.357366 |
7.084605 |
7.260865 |
22.26618 |
1 |
rowWeightedMedians |
7.124855 |
7.522170 |
8.146047 |
7.674877 |
7.864806 |
25.21561 |
|
expr |
min |
lq |
mean |
median |
uq |
max |
2 |
apply+weigthedMedian |
1.000000 |
1.000000 |
1.000000 |
1.000000 |
1.000000 |
1.000000 |
1 |
rowWeightedMedians |
1.079257 |
1.083671 |
1.107196 |
1.083318 |
1.083178 |
1.132462 |
Figure: Benchmarking of colWeightedMedians() and apply+weigthedMedian() on 100x1000 data as well as rowWeightedMedians() and apply+weigthedMedian() on the same data transposed. Outliers are displayed as crosses. Times are in milliseconds.
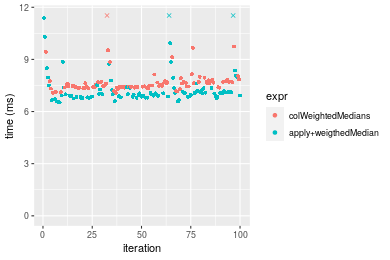
Table: Benchmarking of colWeightedMedians() and rowWeightedMedians() on 100x1000 data (original and transposed). The top panel shows times in milliseconds and the bottom panel shows relative times.
|
expr |
min |
lq |
mean |
median |
uq |
max |
1 |
colWeightedMedians |
7.072074 |
7.387505 |
7.805397 |
7.489342 |
7.701308 |
24.78461 |
2 |
rowWeightedMedians |
7.124855 |
7.522170 |
8.146047 |
7.674877 |
7.864806 |
25.21561 |
|
expr |
min |
lq |
mean |
median |
uq |
max |
1 |
colWeightedMedians |
1.000000 |
1.000000 |
1.000000 |
1.000000 |
1.00000 |
1.00000 |
2 |
rowWeightedMedians |
1.007463 |
1.018229 |
1.043643 |
1.024773 |
1.02123 |
1.01739 |
Figure: Benchmarking of colWeightedMedians() and rowWeightedMedians() on 100x1000 data (original and transposed). Outliers are displayed as crosses. Times are in milliseconds.
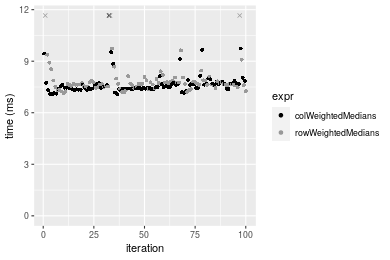
1000x100 matrix
> X <- data[["1000x100"]]
> w <- runif(nrow(X))
> gc()
used (Mb) gc trigger (Mb) max used (Mb)
Ncells 5337196 285.1 7916910 422.9 7916910 422.9
Vcells 10530235 80.4 33191153 253.3 53339345 407.0
> colStats <- microbenchmark(colWeightedMedians = colWeightedMedians(X, w = w, na.rm = FALSE), `apply+weigthedMedian` = apply(X,
+ MARGIN = 2L, FUN = weightedMedian, w = w, na.rm = FALSE), unit = "ms")
> X <- t(X)
> gc()
used (Mb) gc trigger (Mb) max used (Mb)
Ncells 5337190 285.1 7916910 422.9 7916910 422.9
Vcells 10630278 81.2 33191153 253.3 53339345 407.0
> rowStats <- microbenchmark(rowWeightedMedians = rowWeightedMedians(X, w = w, na.rm = FALSE), `apply+weigthedMedian` = apply(X,
+ MARGIN = 1L, FUN = weightedMedian, w = w, na.rm = FALSE), unit = "ms")
Table: Benchmarking of colWeightedMedians() and apply+weigthedMedian() on 1000x100 data. The top panel shows times in milliseconds and the bottom panel shows relative times.
|
expr |
min |
lq |
mean |
median |
uq |
max |
2 |
apply+weigthedMedian |
5.402375 |
5.643393 |
5.845511 |
5.670750 |
5.723949 |
15.49405 |
1 |
colWeightedMedians |
5.484320 |
5.708277 |
5.979079 |
5.750507 |
5.839806 |
14.18323 |
|
expr |
min |
lq |
mean |
median |
uq |
max |
2 |
apply+weigthedMedian |
1.000000 |
1.000000 |
1.00000 |
1.000000 |
1.000000 |
1.0000000 |
1 |
colWeightedMedians |
1.015168 |
1.011497 |
1.02285 |
1.014065 |
1.020241 |
0.9153984 |
Table: Benchmarking of rowWeightedMedians() and apply+weigthedMedian() on 1000x100 data (transposed). The top panel shows times in milliseconds and the bottom panel shows relative times.
|
expr |
min |
lq |
mean |
median |
uq |
max |
2 |
apply+weigthedMedian |
5.409707 |
5.660353 |
5.975777 |
5.687883 |
5.770017 |
15.738789 |
1 |
rowWeightedMedians |
5.479955 |
5.721429 |
5.949951 |
5.765739 |
5.817060 |
9.323769 |
|
expr |
min |
lq |
mean |
median |
uq |
max |
2 |
apply+weigthedMedian |
1.000000 |
1.00000 |
1.0000000 |
1.000000 |
1.000000 |
1.000000 |
1 |
rowWeightedMedians |
1.012986 |
1.01079 |
0.9956781 |
1.013688 |
1.008153 |
0.592407 |
Figure: Benchmarking of colWeightedMedians() and apply+weigthedMedian() on 1000x100 data as well as rowWeightedMedians() and apply+weigthedMedian() on the same data transposed. Outliers are displayed as crosses. Times are in milliseconds.
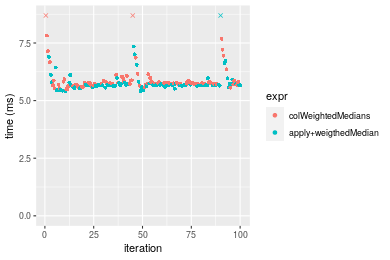
Table: Benchmarking of colWeightedMedians() and rowWeightedMedians() on 1000x100 data (original and transposed). The top panel shows times in milliseconds and the bottom panel shows relative times.
|
expr |
min |
lq |
mean |
median |
uq |
max |
1 |
colWeightedMedians |
5.484320 |
5.708277 |
5.979079 |
5.750507 |
5.839806 |
14.183225 |
2 |
rowWeightedMedians |
5.479955 |
5.721429 |
5.949951 |
5.765739 |
5.817060 |
9.323769 |
|
expr |
min |
lq |
mean |
median |
uq |
max |
1 |
colWeightedMedians |
1.0000000 |
1.000000 |
1.0000000 |
1.000000 |
1.0000000 |
1.00000 |
2 |
rowWeightedMedians |
0.9992041 |
1.002304 |
0.9951283 |
1.002649 |
0.9961049 |
0.65738 |
Figure: Benchmarking of colWeightedMedians() and rowWeightedMedians() on 1000x100 data (original and transposed). Outliers are displayed as crosses. Times are in milliseconds.
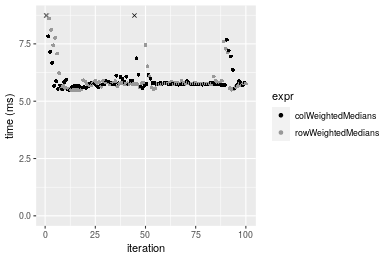
Appendix
R version 4.1.1 Patched (2021-08-10 r80727)
Platform: x86_64-pc-linux-gnu (64-bit)
Running under: Ubuntu 18.04.5 LTS
Matrix products: default
BLAS: /home/hb/software/R-devel/R-4-1-branch/lib/R/lib/libRblas.so
LAPACK: /home/hb/software/R-devel/R-4-1-branch/lib/R/lib/libRlapack.so
locale:
[1] LC_CTYPE=en_US.UTF-8 LC_NUMERIC=C
[3] LC_TIME=en_US.UTF-8 LC_COLLATE=en_US.UTF-8
[5] LC_MONETARY=en_US.UTF-8 LC_MESSAGES=en_US.UTF-8
[7] LC_PAPER=en_US.UTF-8 LC_NAME=C
[9] LC_ADDRESS=C LC_TELEPHONE=C
[11] LC_MEASUREMENT=en_US.UTF-8 LC_IDENTIFICATION=C
attached base packages:
[1] stats graphics grDevices utils datasets methods base
other attached packages:
[1] microbenchmark_1.4-7 matrixStats_0.60.0 ggplot2_3.3.5
[4] knitr_1.33 R.devices_2.17.0 R.utils_2.10.1
[7] R.oo_1.24.0 R.methodsS3_1.8.1-9001 history_0.0.1-9000
loaded via a namespace (and not attached):
[1] Biobase_2.52.0 httr_1.4.2 splines_4.1.1
[4] bit64_4.0.5 network_1.17.1 assertthat_0.2.1
[7] highr_0.9 stats4_4.1.1 blob_1.2.2
[10] GenomeInfoDbData_1.2.6 robustbase_0.93-8 pillar_1.6.2
[13] RSQLite_2.2.8 lattice_0.20-44 glue_1.4.2
[16] digest_0.6.27 XVector_0.32.0 colorspace_2.0-2
[19] Matrix_1.3-4 XML_3.99-0.7 pkgconfig_2.0.3
[22] zlibbioc_1.38.0 genefilter_1.74.0 purrr_0.3.4
[25] ergm_4.1.2 xtable_1.8-4 scales_1.1.1
[28] tibble_3.1.4 annotate_1.70.0 KEGGREST_1.32.0
[31] farver_2.1.0 generics_0.1.0 IRanges_2.26.0
[34] ellipsis_0.3.2 cachem_1.0.6 withr_2.4.2
[37] BiocGenerics_0.38.0 mime_0.11 survival_3.2-13
[40] magrittr_2.0.1 crayon_1.4.1 statnet.common_4.5.0
[43] memoise_2.0.0 laeken_0.5.1 fansi_0.5.0
[46] R.cache_0.15.0 MASS_7.3-54 R.rsp_0.44.0
[49] progressr_0.8.0 tools_4.1.1 lifecycle_1.0.0
[52] S4Vectors_0.30.0 trust_0.1-8 munsell_0.5.0
[55] tabby_0.0.1-9001 AnnotationDbi_1.54.1 Biostrings_2.60.2
[58] compiler_4.1.1 GenomeInfoDb_1.28.1 rlang_0.4.11
[61] grid_4.1.1 RCurl_1.98-1.4 cwhmisc_6.6
[64] rstudioapi_0.13 rappdirs_0.3.3 startup_0.15.0
[67] labeling_0.4.2 bitops_1.0-7 base64enc_0.1-3
[70] boot_1.3-28 gtable_0.3.0 DBI_1.1.1
[73] markdown_1.1 R6_2.5.1 lpSolveAPI_5.5.2.0-17.7
[76] rle_0.9.2 dplyr_1.0.7 fastmap_1.1.0
[79] bit_4.0.4 utf8_1.2.2 parallel_4.1.1
[82] Rcpp_1.0.7 vctrs_0.3.8 png_0.1-7
[85] DEoptimR_1.0-9 tidyselect_1.1.1 xfun_0.25
[88] coda_0.19-4
Total processing time was 19.7 secs.
Reproducibility
To reproduce this report, do:
html <- matrixStats:::benchmark('colWeightedMedians')
Copyright Henrik Bengtsson. Last updated on 2021-08-25 22:32:51 (+0200 UTC). Powered by RSP.