colWeightedMeans() and rowWeightedMeans() benchmarks
This report benchmark the performance of colWeightedMeans() and rowWeightedMeans() against alternative methods.
Alternative methods
- apply() + weighted.mean()
Data
> rmatrix <- function(nrow, ncol, mode = c("logical", "double", "integer", "index"), range = c(-100,
+ +100), na_prob = 0) {
+ mode <- match.arg(mode)
+ n <- nrow * ncol
+ if (mode == "logical") {
+ x <- sample(c(FALSE, TRUE), size = n, replace = TRUE)
+ } else if (mode == "index") {
+ x <- seq_len(n)
+ mode <- "integer"
+ } else {
+ x <- runif(n, min = range[1], max = range[2])
+ }
+ storage.mode(x) <- mode
+ if (na_prob > 0)
+ x[sample(n, size = na_prob * n)] <- NA
+ dim(x) <- c(nrow, ncol)
+ x
+ }
> rmatrices <- function(scale = 10, seed = 1, ...) {
+ set.seed(seed)
+ data <- list()
+ data[[1]] <- rmatrix(nrow = scale * 1, ncol = scale * 1, ...)
+ data[[2]] <- rmatrix(nrow = scale * 10, ncol = scale * 10, ...)
+ data[[3]] <- rmatrix(nrow = scale * 100, ncol = scale * 1, ...)
+ data[[4]] <- t(data[[3]])
+ data[[5]] <- rmatrix(nrow = scale * 10, ncol = scale * 100, ...)
+ data[[6]] <- t(data[[5]])
+ names(data) <- sapply(data, FUN = function(x) paste(dim(x), collapse = "x"))
+ data
+ }
> data <- rmatrices(mode = "double")
Results
10x10 matrix
> X <- data[["10x10"]]
> w <- runif(nrow(X))
> gc()
used (Mb) gc trigger (Mb) max used (Mb)
Ncells 5331809 284.8 7916910 422.9 7916910 422.9
Vcells 10856214 82.9 33191153 253.3 53339345 407.0
> colStats <- microbenchmark(colWeightedMeans = colWeightedMeans(X, w = w, na.rm = FALSE), `apply+weigthed.mean` = apply(X,
+ MARGIN = 2L, FUN = weighted.mean, w = w, na.rm = FALSE), unit = "ms")
> X <- t(X)
> gc()
used (Mb) gc trigger (Mb) max used (Mb)
Ncells 5331103 284.8 7916910 422.9 7916910 422.9
Vcells 10854208 82.9 33191153 253.3 53339345 407.0
> rowStats <- microbenchmark(rowWeightedMeans = rowWeightedMeans(X, w = w, na.rm = FALSE), `apply+weigthed.mean` = apply(X,
+ MARGIN = 1L, FUN = weighted.mean, w = w, na.rm = FALSE), unit = "ms")
Table: Benchmarking of colWeightedMeans() and apply+weigthed.mean() on 10x10 data. The top panel shows times in milliseconds and the bottom panel shows relative times.
|
expr |
min |
lq |
mean |
median |
uq |
max |
1 |
colWeightedMeans |
0.014514 |
0.0158945 |
0.0183756 |
0.0173735 |
0.0196270 |
0.051723 |
2 |
apply+weigthed.mean |
0.081258 |
0.0862375 |
0.0911114 |
0.0889395 |
0.0908155 |
0.306399 |
|
expr |
min |
lq |
mean |
median |
uq |
max |
1 |
colWeightedMeans |
1.000000 |
1.000000 |
1.000000 |
1.000000 |
1.00000 |
1.000000 |
2 |
apply+weigthed.mean |
5.598594 |
5.425619 |
4.958286 |
5.119262 |
4.62707 |
5.923844 |
Table: Benchmarking of rowWeightedMeans() and apply+weigthed.mean() on 10x10 data (transposed). The top panel shows times in milliseconds and the bottom panel shows relative times.
|
expr |
min |
lq |
mean |
median |
uq |
max |
1 |
rowWeightedMeans |
0.020235 |
0.0219355 |
0.0248860 |
0.0244995 |
0.0253815 |
0.066676 |
2 |
apply+weigthed.mean |
0.081370 |
0.0890960 |
0.0923169 |
0.0909045 |
0.0918575 |
0.171881 |
|
expr |
min |
lq |
mean |
median |
uq |
max |
1 |
rowWeightedMeans |
1.00000 |
1.000000 |
1.000000 |
1.000000 |
1.000000 |
1.000000 |
2 |
apply+weigthed.mean |
4.02125 |
4.061726 |
3.709588 |
3.710463 |
3.619073 |
2.577854 |
Figure: Benchmarking of colWeightedMeans() and apply+weigthed.mean() on 10x10 data as well as rowWeightedMeans() and apply+weigthed.mean() on the same data transposed. Outliers are displayed as crosses. Times are in milliseconds.
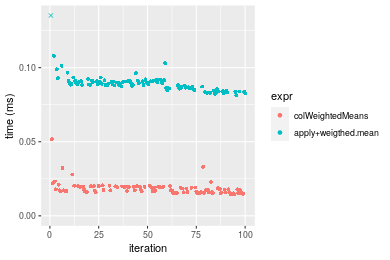
Table: Benchmarking of colWeightedMeans() and rowWeightedMeans() on 10x10 data (original and transposed). The top panel shows times in milliseconds and the bottom panel shows relative times.
|
expr |
min |
lq |
mean |
median |
uq |
max |
1 |
colWeightedMeans |
14.514 |
15.8945 |
18.37558 |
17.3735 |
19.6270 |
51.723 |
2 |
rowWeightedMeans |
20.235 |
21.9355 |
24.88602 |
24.4995 |
25.3815 |
66.676 |
|
expr |
min |
lq |
mean |
median |
uq |
max |
1 |
colWeightedMeans |
1.000000 |
1.000000 |
1.000000 |
1.000000 |
1.000000 |
1.000000 |
2 |
rowWeightedMeans |
1.394171 |
1.380069 |
1.354299 |
1.410165 |
1.293193 |
1.289098 |
Figure: Benchmarking of colWeightedMeans() and rowWeightedMeans() on 10x10 data (original and transposed). Outliers are displayed as crosses. Times are in milliseconds.
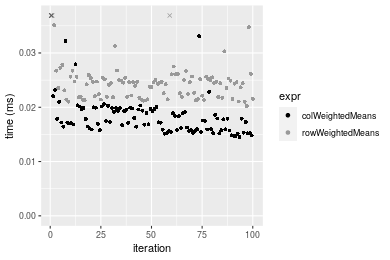
100x100 matrix
> X <- data[["100x100"]]
> w <- runif(nrow(X))
> gc()
used (Mb) gc trigger (Mb) max used (Mb)
Ncells 5329664 284.7 7916910 422.9 7916910 422.9
Vcells 10469040 79.9 33191153 253.3 53339345 407.0
> colStats <- microbenchmark(colWeightedMeans = colWeightedMeans(X, w = w, na.rm = FALSE), `apply+weigthed.mean` = apply(X,
+ MARGIN = 2L, FUN = weighted.mean, w = w, na.rm = FALSE), unit = "ms")
> X <- t(X)
> gc()
used (Mb) gc trigger (Mb) max used (Mb)
Ncells 5329658 284.7 7916910 422.9 7916910 422.9
Vcells 10479083 80.0 33191153 253.3 53339345 407.0
> rowStats <- microbenchmark(rowWeightedMeans = rowWeightedMeans(X, w = w, na.rm = FALSE), `apply+weigthed.mean` = apply(X,
+ MARGIN = 1L, FUN = weighted.mean, w = w, na.rm = FALSE), unit = "ms")
Table: Benchmarking of colWeightedMeans() and apply+weigthed.mean() on 100x100 data. The top panel shows times in milliseconds and the bottom panel shows relative times.
|
expr |
min |
lq |
mean |
median |
uq |
max |
1 |
colWeightedMeans |
0.030195 |
0.032921 |
0.0382298 |
0.03661 |
0.0418240 |
0.084657 |
2 |
apply+weigthed.mean |
0.534847 |
0.554290 |
0.6471003 |
0.63325 |
0.7064345 |
1.068205 |
|
expr |
min |
lq |
mean |
median |
uq |
max |
1 |
colWeightedMeans |
1.0000 |
1.00000 |
1.00000 |
1.00000 |
1.00000 |
1.00000 |
2 |
apply+weigthed.mean |
17.7131 |
16.83697 |
16.92659 |
17.29719 |
16.89065 |
12.61804 |
Table: Benchmarking of rowWeightedMeans() and apply+weigthed.mean() on 100x100 data (transposed). The top panel shows times in milliseconds and the bottom panel shows relative times.
|
expr |
min |
lq |
mean |
median |
uq |
max |
1 |
rowWeightedMeans |
0.108045 |
0.114521 |
0.1378237 |
0.1341905 |
0.1548515 |
0.224745 |
2 |
apply+weigthed.mean |
0.535381 |
0.558136 |
0.6648594 |
0.6451205 |
0.7158065 |
1.127485 |
|
expr |
min |
lq |
mean |
median |
uq |
max |
1 |
rowWeightedMeans |
1.000000 |
1.000000 |
1.000000 |
1.000000 |
1.000000 |
1.00000 |
2 |
apply+weigthed.mean |
4.955167 |
4.873656 |
4.823984 |
4.807498 |
4.622535 |
5.01673 |
Figure: Benchmarking of colWeightedMeans() and apply+weigthed.mean() on 100x100 data as well as rowWeightedMeans() and apply+weigthed.mean() on the same data transposed. Outliers are displayed as crosses. Times are in milliseconds.
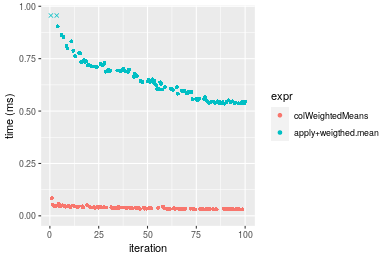
Table: Benchmarking of colWeightedMeans() and rowWeightedMeans() on 100x100 data (original and transposed). The top panel shows times in milliseconds and the bottom panel shows relative times.
|
expr |
min |
lq |
mean |
median |
uq |
max |
1 |
colWeightedMeans |
30.195 |
32.921 |
38.2298 |
36.6100 |
41.8240 |
84.657 |
2 |
rowWeightedMeans |
108.045 |
114.521 |
137.8237 |
134.1905 |
154.8515 |
224.745 |
|
expr |
min |
lq |
mean |
median |
uq |
max |
1 |
colWeightedMeans |
1.000000 |
1.000000 |
1.000000 |
1.000000 |
1.000000 |
1.000000 |
2 |
rowWeightedMeans |
3.578241 |
3.478661 |
3.605138 |
3.665406 |
3.702456 |
2.654772 |
Figure: Benchmarking of colWeightedMeans() and rowWeightedMeans() on 100x100 data (original and transposed). Outliers are displayed as crosses. Times are in milliseconds.
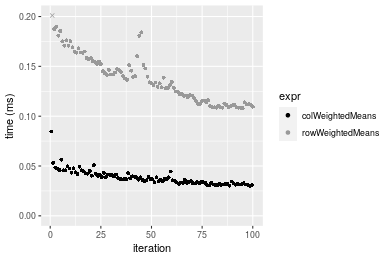
1000x10 matrix
> X <- data[["1000x10"]]
> w <- runif(nrow(X))
> gc()
used (Mb) gc trigger (Mb) max used (Mb)
Ncells 5330389 284.7 7916910 422.9 7916910 422.9
Vcells 10473445 80.0 33191153 253.3 53339345 407.0
> colStats <- microbenchmark(colWeightedMeans = colWeightedMeans(X, w = w, na.rm = FALSE), `apply+weigthed.mean` = apply(X,
+ MARGIN = 2L, FUN = weighted.mean, w = w, na.rm = FALSE), unit = "ms")
> X <- t(X)
> gc()
used (Mb) gc trigger (Mb) max used (Mb)
Ncells 5330377 284.7 7916910 422.9 7916910 422.9
Vcells 10483478 80.0 33191153 253.3 53339345 407.0
> rowStats <- microbenchmark(rowWeightedMeans = rowWeightedMeans(X, w = w, na.rm = FALSE), `apply+weigthed.mean` = apply(X,
+ MARGIN = 1L, FUN = weighted.mean, w = w, na.rm = FALSE), unit = "ms")
Table: Benchmarking of colWeightedMeans() and apply+weigthed.mean() on 1000x10 data. The top panel shows times in milliseconds and the bottom panel shows relative times.
|
expr |
min |
lq |
mean |
median |
uq |
max |
1 |
colWeightedMeans |
0.050207 |
0.052973 |
0.0616090 |
0.058177 |
0.0663215 |
0.159488 |
2 |
apply+weigthed.mean |
0.207594 |
0.215316 |
0.2434847 |
0.231619 |
0.2656575 |
0.379112 |
|
expr |
min |
lq |
mean |
median |
uq |
max |
1 |
colWeightedMeans |
1.000000 |
1.000000 |
1.000000 |
1.000000 |
1.000000 |
1.000000 |
2 |
apply+weigthed.mean |
4.134762 |
4.064637 |
3.952096 |
3.981281 |
4.005602 |
2.377057 |
Table: Benchmarking of rowWeightedMeans() and apply+weigthed.mean() on 1000x10 data (transposed). The top panel shows times in milliseconds and the bottom panel shows relative times.
|
expr |
min |
lq |
mean |
median |
uq |
max |
1 |
rowWeightedMeans |
0.142004 |
0.1511195 |
0.1673899 |
0.160325 |
0.179218 |
0.262431 |
2 |
apply+weigthed.mean |
0.202047 |
0.2183060 |
0.2437231 |
0.236016 |
0.261967 |
0.353293 |
|
expr |
min |
lq |
mean |
median |
uq |
max |
1 |
rowWeightedMeans |
1.000000 |
1.000000 |
1.00000 |
1.00000 |
1.000000 |
1.000000 |
2 |
apply+weigthed.mean |
1.422826 |
1.444592 |
1.45602 |
1.47211 |
1.461723 |
1.346232 |
Figure: Benchmarking of colWeightedMeans() and apply+weigthed.mean() on 1000x10 data as well as rowWeightedMeans() and apply+weigthed.mean() on the same data transposed. Outliers are displayed as crosses. Times are in milliseconds.
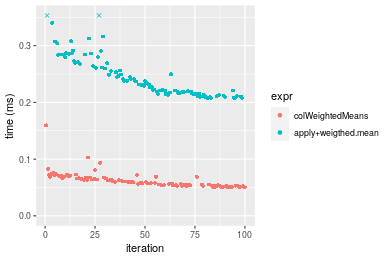
Table: Benchmarking of colWeightedMeans() and rowWeightedMeans() on 1000x10 data (original and transposed). The top panel shows times in milliseconds and the bottom panel shows relative times.
|
expr |
min |
lq |
mean |
median |
uq |
max |
1 |
colWeightedMeans |
50.207 |
52.9730 |
61.60899 |
58.177 |
66.3215 |
159.488 |
2 |
rowWeightedMeans |
142.004 |
151.1195 |
167.38994 |
160.325 |
179.2180 |
262.431 |
|
expr |
min |
lq |
mean |
median |
uq |
max |
1 |
colWeightedMeans |
1.000000 |
1.000000 |
1.000000 |
1.000000 |
1.000000 |
1.000000 |
2 |
rowWeightedMeans |
2.828371 |
2.852765 |
2.716973 |
2.755814 |
2.702261 |
1.645459 |
Figure: Benchmarking of colWeightedMeans() and rowWeightedMeans() on 1000x10 data (original and transposed). Outliers are displayed as crosses. Times are in milliseconds.
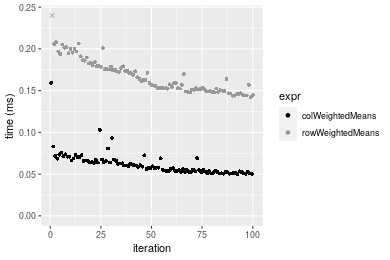
10x1000 matrix
> X <- data[["10x1000"]]
> w <- runif(nrow(X))
> gc()
used (Mb) gc trigger (Mb) max used (Mb)
Ncells 5330581 284.7 7916910 422.9 7916910 422.9
Vcells 10473235 80.0 33191153 253.3 53339345 407.0
> colStats <- microbenchmark(colWeightedMeans = colWeightedMeans(X, w = w, na.rm = FALSE), `apply+weigthed.mean` = apply(X,
+ MARGIN = 2L, FUN = weighted.mean, w = w, na.rm = FALSE), unit = "ms")
> X <- t(X)
> gc()
used (Mb) gc trigger (Mb) max used (Mb)
Ncells 5330575 284.7 7916910 422.9 7916910 422.9
Vcells 10483278 80.0 33191153 253.3 53339345 407.0
> rowStats <- microbenchmark(rowWeightedMeans = rowWeightedMeans(X, w = w, na.rm = FALSE), `apply+weigthed.mean` = apply(X,
+ MARGIN = 1L, FUN = weighted.mean, w = w, na.rm = FALSE), unit = "ms")
Table: Benchmarking of colWeightedMeans() and apply+weigthed.mean() on 10x1000 data. The top panel shows times in milliseconds and the bottom panel shows relative times.
|
expr |
min |
lq |
mean |
median |
uq |
max |
1 |
colWeightedMeans |
0.027890 |
0.031472 |
0.0402594 |
0.0373785 |
0.043889 |
0.100983 |
2 |
apply+weigthed.mean |
4.093371 |
4.224227 |
4.6551195 |
4.3682620 |
4.654880 |
12.683915 |
|
expr |
min |
lq |
mean |
median |
uq |
max |
1 |
colWeightedMeans |
1.0000 |
1.0000 |
1.0000 |
1.0000 |
1.0000 |
1.0000 |
2 |
apply+weigthed.mean |
146.7684 |
134.2218 |
115.6281 |
116.8656 |
106.0603 |
125.6045 |
Table: Benchmarking of rowWeightedMeans() and apply+weigthed.mean() on 10x1000 data (transposed). The top panel shows times in milliseconds and the bottom panel shows relative times.
|
expr |
min |
lq |
mean |
median |
uq |
max |
1 |
rowWeightedMeans |
0.106895 |
0.1135865 |
0.1268637 |
0.121555 |
0.1352205 |
0.223218 |
2 |
apply+weigthed.mean |
4.066901 |
4.2600495 |
4.6186294 |
4.353472 |
4.5128765 |
11.151535 |
|
expr |
min |
lq |
mean |
median |
uq |
max |
1 |
rowWeightedMeans |
1.00000 |
1.00000 |
1.00000 |
1.00000 |
1.0000 |
1.00000 |
2 |
apply+weigthed.mean |
38.04576 |
37.50489 |
36.40624 |
35.81484 |
33.3742 |
49.95805 |
Figure: Benchmarking of colWeightedMeans() and apply+weigthed.mean() on 10x1000 data as well as rowWeightedMeans() and apply+weigthed.mean() on the same data transposed. Outliers are displayed as crosses. Times are in milliseconds.
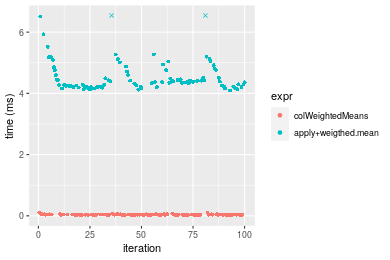
Table: Benchmarking of colWeightedMeans() and rowWeightedMeans() on 10x1000 data (original and transposed). The top panel shows times in milliseconds and the bottom panel shows relative times.
|
expr |
min |
lq |
mean |
median |
uq |
max |
1 |
colWeightedMeans |
27.890 |
31.4720 |
40.25943 |
37.3785 |
43.8890 |
100.983 |
2 |
rowWeightedMeans |
106.895 |
113.5865 |
126.86366 |
121.5550 |
135.2205 |
223.218 |
|
expr |
min |
lq |
mean |
median |
uq |
max |
1 |
colWeightedMeans |
1.000000 |
1.000000 |
1.000000 |
1.000000 |
1.000000 |
1.000000 |
2 |
rowWeightedMeans |
3.832736 |
3.609129 |
3.151154 |
3.252003 |
3.080966 |
2.210451 |
Figure: Benchmarking of colWeightedMeans() and rowWeightedMeans() on 10x1000 data (original and transposed). Outliers are displayed as crosses. Times are in milliseconds.
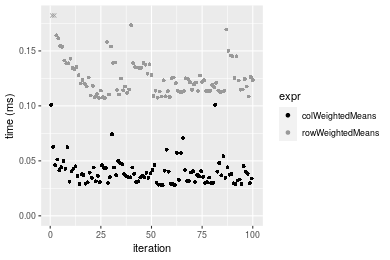
100x1000 matrix
> X <- data[["100x1000"]]
> w <- runif(nrow(X))
> gc()
used (Mb) gc trigger (Mb) max used (Mb)
Ncells 5330777 284.7 7916910 422.9 7916910 422.9
Vcells 10473841 80.0 33191153 253.3 53339345 407.0
> colStats <- microbenchmark(colWeightedMeans = colWeightedMeans(X, w = w, na.rm = FALSE), `apply+weigthed.mean` = apply(X,
+ MARGIN = 2L, FUN = weighted.mean, w = w, na.rm = FALSE), unit = "ms")
> X <- t(X)
> gc()
used (Mb) gc trigger (Mb) max used (Mb)
Ncells 5330765 284.7 7916910 422.9 7916910 422.9
Vcells 10573874 80.7 33191153 253.3 53339345 407.0
> rowStats <- microbenchmark(rowWeightedMeans = rowWeightedMeans(X, w = w, na.rm = FALSE), `apply+weigthed.mean` = apply(X,
+ MARGIN = 1L, FUN = weighted.mean, w = w, na.rm = FALSE), unit = "ms")
Table: Benchmarking of colWeightedMeans() and apply+weigthed.mean() on 100x1000 data. The top panel shows times in milliseconds and the bottom panel shows relative times.
|
expr |
min |
lq |
mean |
median |
uq |
max |
1 |
colWeightedMeans |
0.176290 |
0.2078795 |
0.4456043 |
0.234187 |
0.270846 |
20.88489 |
2 |
apply+weigthed.mean |
5.194491 |
5.3765175 |
6.1930076 |
5.488162 |
5.870243 |
27.26077 |
|
expr |
min |
lq |
mean |
median |
uq |
max |
1 |
colWeightedMeans |
1.0000 |
1.00000 |
1.000 |
1.00000 |
1.00000 |
1.000000 |
2 |
apply+weigthed.mean |
29.4656 |
25.86363 |
13.898 |
23.43496 |
21.67373 |
1.305286 |
Table: Benchmarking of rowWeightedMeans() and apply+weigthed.mean() on 100x1000 data (transposed). The top panel shows times in milliseconds and the bottom panel shows relative times.
|
expr |
min |
lq |
mean |
median |
uq |
max |
1 |
rowWeightedMeans |
0.939194 |
0.9811385 |
1.280713 |
1.007786 |
1.164391 |
22.33007 |
2 |
apply+weigthed.mean |
5.244182 |
5.3788310 |
6.250966 |
5.525034 |
5.953479 |
28.91215 |
|
expr |
min |
lq |
mean |
median |
uq |
max |
1 |
rowWeightedMeans |
1.000000 |
1.000000 |
1.000000 |
1.000000 |
1.000000 |
1.000000 |
2 |
apply+weigthed.mean |
5.583705 |
5.482234 |
4.880847 |
5.482348 |
5.112957 |
1.294763 |
Figure: Benchmarking of colWeightedMeans() and apply+weigthed.mean() on 100x1000 data as well as rowWeightedMeans() and apply+weigthed.mean() on the same data transposed. Outliers are displayed as crosses. Times are in milliseconds.
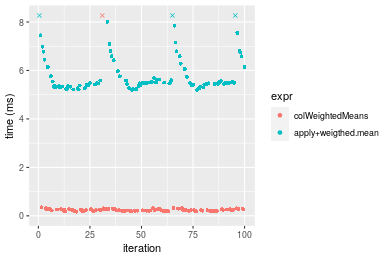
Table: Benchmarking of colWeightedMeans() and rowWeightedMeans() on 100x1000 data (original and transposed). The top panel shows times in milliseconds and the bottom panel shows relative times.
|
expr |
min |
lq |
mean |
median |
uq |
max |
1 |
colWeightedMeans |
176.290 |
207.8795 |
445.6043 |
234.187 |
270.846 |
20884.89 |
2 |
rowWeightedMeans |
939.194 |
981.1385 |
1280.7132 |
1007.786 |
1164.390 |
22330.07 |
|
expr |
min |
lq |
mean |
median |
uq |
max |
1 |
colWeightedMeans |
1.000000 |
1.000000 |
1.000000 |
1.000000 |
1.000000 |
1.000000 |
2 |
rowWeightedMeans |
5.327551 |
4.719746 |
2.874104 |
4.303339 |
4.299087 |
1.069197 |
Figure: Benchmarking of colWeightedMeans() and rowWeightedMeans() on 100x1000 data (original and transposed). Outliers are displayed as crosses. Times are in milliseconds.
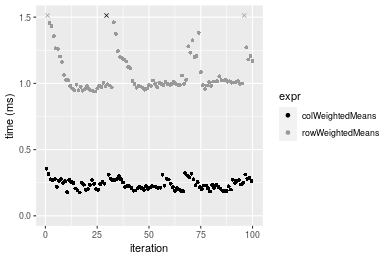
1000x100 matrix
> X <- data[["1000x100"]]
> w <- runif(nrow(X))
> gc()
used (Mb) gc trigger (Mb) max used (Mb)
Ncells 5330958 284.8 7916910 422.9 7916910 422.9
Vcells 10475373 80.0 33191153 253.3 53339345 407.0
> colStats <- microbenchmark(colWeightedMeans = colWeightedMeans(X, w = w, na.rm = FALSE), `apply+weigthed.mean` = apply(X,
+ MARGIN = 2L, FUN = weighted.mean, w = w, na.rm = FALSE), unit = "ms")
> X <- t(X)
> gc()
used (Mb) gc trigger (Mb) max used (Mb)
Ncells 5330952 284.8 7916910 422.9 7916910 422.9
Vcells 10575416 80.7 33191153 253.3 53339345 407.0
> rowStats <- microbenchmark(rowWeightedMeans = rowWeightedMeans(X, w = w, na.rm = FALSE), `apply+weigthed.mean` = apply(X,
+ MARGIN = 1L, FUN = weighted.mean, w = w, na.rm = FALSE), unit = "ms")
Table: Benchmarking of colWeightedMeans() and apply+weigthed.mean() on 1000x100 data. The top panel shows times in milliseconds and the bottom panel shows relative times.
|
expr |
min |
lq |
mean |
median |
uq |
max |
1 |
colWeightedMeans |
0.201873 |
0.2324025 |
0.2648283 |
0.2548875 |
0.281130 |
0.443784 |
2 |
apply+weigthed.mean |
1.514922 |
1.6610620 |
5.8543197 |
1.8988915 |
2.089488 |
376.589480 |
|
expr |
min |
lq |
mean |
median |
uq |
max |
1 |
colWeightedMeans |
1.000000 |
1.00000 |
1.00000 |
1.00000 |
1.000000 |
1.0000 |
2 |
apply+weigthed.mean |
7.504332 |
7.14735 |
22.10609 |
7.44992 |
7.432464 |
848.5873 |
Table: Benchmarking of rowWeightedMeans() and apply+weigthed.mean() on 1000x100 data (transposed). The top panel shows times in milliseconds and the bottom panel shows relative times.
|
expr |
min |
lq |
mean |
median |
uq |
max |
1 |
rowWeightedMeans |
0.959981 |
0.979435 |
1.125434 |
1.090402 |
1.228889 |
1.656196 |
2 |
apply+weigthed.mean |
1.523706 |
1.564525 |
2.017792 |
1.617954 |
1.943826 |
11.460256 |
|
expr |
min |
lq |
mean |
median |
uq |
max |
1 |
rowWeightedMeans |
1.000000 |
1.000000 |
1.000000 |
1.000000 |
1.000000 |
1.000000 |
2 |
apply+weigthed.mean |
1.587225 |
1.597374 |
1.792902 |
1.483814 |
1.581775 |
6.919626 |
Figure: Benchmarking of colWeightedMeans() and apply+weigthed.mean() on 1000x100 data as well as rowWeightedMeans() and apply+weigthed.mean() on the same data transposed. Outliers are displayed as crosses. Times are in milliseconds.
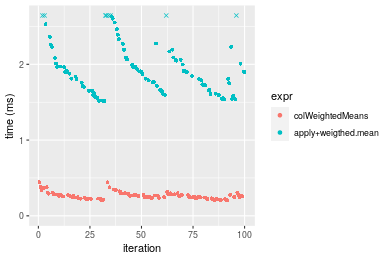
Table: Benchmarking of colWeightedMeans() and rowWeightedMeans() on 1000x100 data (original and transposed). The top panel shows times in milliseconds and the bottom panel shows relative times.
|
expr |
min |
lq |
mean |
median |
uq |
max |
1 |
colWeightedMeans |
201.873 |
232.4025 |
264.8283 |
254.8875 |
281.130 |
443.784 |
2 |
rowWeightedMeans |
959.981 |
979.4350 |
1125.4340 |
1090.4015 |
1228.889 |
1656.196 |
|
expr |
min |
lq |
mean |
median |
uq |
max |
1 |
colWeightedMeans |
1.000000 |
1.000000 |
1.000000 |
1.000000 |
1.000000 |
1.000000 |
2 |
rowWeightedMeans |
4.755371 |
4.214391 |
4.249674 |
4.277972 |
4.371248 |
3.731987 |
Figure: Benchmarking of colWeightedMeans() and rowWeightedMeans() on 1000x100 data (original and transposed). Outliers are displayed as crosses. Times are in milliseconds.
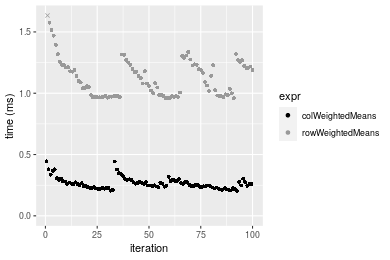
Appendix
R version 4.1.1 Patched (2021-08-10 r80727)
Platform: x86_64-pc-linux-gnu (64-bit)
Running under: Ubuntu 18.04.5 LTS
Matrix products: default
BLAS: /home/hb/software/R-devel/R-4-1-branch/lib/R/lib/libRblas.so
LAPACK: /home/hb/software/R-devel/R-4-1-branch/lib/R/lib/libRlapack.so
locale:
[1] LC_CTYPE=en_US.UTF-8 LC_NUMERIC=C
[3] LC_TIME=en_US.UTF-8 LC_COLLATE=en_US.UTF-8
[5] LC_MONETARY=en_US.UTF-8 LC_MESSAGES=en_US.UTF-8
[7] LC_PAPER=en_US.UTF-8 LC_NAME=C
[9] LC_ADDRESS=C LC_TELEPHONE=C
[11] LC_MEASUREMENT=en_US.UTF-8 LC_IDENTIFICATION=C
attached base packages:
[1] stats graphics grDevices utils datasets methods base
other attached packages:
[1] microbenchmark_1.4-7 matrixStats_0.60.0 ggplot2_3.3.5
[4] knitr_1.33 R.devices_2.17.0 R.utils_2.10.1
[7] R.oo_1.24.0 R.methodsS3_1.8.1-9001 history_0.0.1-9000
loaded via a namespace (and not attached):
[1] Biobase_2.52.0 httr_1.4.2 splines_4.1.1
[4] bit64_4.0.5 network_1.17.1 assertthat_0.2.1
[7] highr_0.9 stats4_4.1.1 blob_1.2.2
[10] GenomeInfoDbData_1.2.6 robustbase_0.93-8 pillar_1.6.2
[13] RSQLite_2.2.8 lattice_0.20-44 glue_1.4.2
[16] digest_0.6.27 XVector_0.32.0 colorspace_2.0-2
[19] Matrix_1.3-4 XML_3.99-0.7 pkgconfig_2.0.3
[22] zlibbioc_1.38.0 genefilter_1.74.0 purrr_0.3.4
[25] ergm_4.1.2 xtable_1.8-4 scales_1.1.1
[28] tibble_3.1.4 annotate_1.70.0 KEGGREST_1.32.0
[31] farver_2.1.0 generics_0.1.0 IRanges_2.26.0
[34] ellipsis_0.3.2 cachem_1.0.6 withr_2.4.2
[37] BiocGenerics_0.38.0 mime_0.11 survival_3.2-13
[40] magrittr_2.0.1 crayon_1.4.1 statnet.common_4.5.0
[43] memoise_2.0.0 laeken_0.5.1 fansi_0.5.0
[46] R.cache_0.15.0 MASS_7.3-54 R.rsp_0.44.0
[49] progressr_0.8.0 tools_4.1.1 lifecycle_1.0.0
[52] S4Vectors_0.30.0 trust_0.1-8 munsell_0.5.0
[55] tabby_0.0.1-9001 AnnotationDbi_1.54.1 Biostrings_2.60.2
[58] compiler_4.1.1 GenomeInfoDb_1.28.1 rlang_0.4.11
[61] grid_4.1.1 RCurl_1.98-1.4 cwhmisc_6.6
[64] rstudioapi_0.13 rappdirs_0.3.3 startup_0.15.0
[67] labeling_0.4.2 bitops_1.0-7 base64enc_0.1-3
[70] boot_1.3-28 gtable_0.3.0 DBI_1.1.1
[73] markdown_1.1 R6_2.5.1 lpSolveAPI_5.5.2.0-17.7
[76] rle_0.9.2 dplyr_1.0.7 fastmap_1.1.0
[79] bit_4.0.4 utf8_1.2.2 parallel_4.1.1
[82] Rcpp_1.0.7 vctrs_0.3.8 png_0.1-7
[85] DEoptimR_1.0-9 tidyselect_1.1.1 xfun_0.25
[88] coda_0.19-4
Total processing time was 15.44 secs.
Reproducibility
To reproduce this report, do:
html <- matrixStats:::benchmark('colWeightedMeans')
Copyright Henrik Bengtsson. Last updated on 2021-08-25 22:32:10 (+0200 UTC). Powered by RSP.