colQuantiles() and rowQuantiles() benchmarks
This report benchmark the performance of colQuantiles() and rowQuantiles() against alternative methods.
Alternative methods
Data
> rmatrix <- function(nrow, ncol, mode = c("logical", "double", "integer", "index"), range = c(-100,
+ +100), na_prob = 0) {
+ mode <- match.arg(mode)
+ n <- nrow * ncol
+ if (mode == "logical") {
+ x <- sample(c(FALSE, TRUE), size = n, replace = TRUE)
+ } else if (mode == "index") {
+ x <- seq_len(n)
+ mode <- "integer"
+ } else {
+ x <- runif(n, min = range[1], max = range[2])
+ }
+ storage.mode(x) <- mode
+ if (na_prob > 0)
+ x[sample(n, size = na_prob * n)] <- NA
+ dim(x) <- c(nrow, ncol)
+ x
+ }
> rmatrices <- function(scale = 10, seed = 1, ...) {
+ set.seed(seed)
+ data <- list()
+ data[[1]] <- rmatrix(nrow = scale * 1, ncol = scale * 1, ...)
+ data[[2]] <- rmatrix(nrow = scale * 10, ncol = scale * 10, ...)
+ data[[3]] <- rmatrix(nrow = scale * 100, ncol = scale * 1, ...)
+ data[[4]] <- t(data[[3]])
+ data[[5]] <- rmatrix(nrow = scale * 10, ncol = scale * 100, ...)
+ data[[6]] <- t(data[[5]])
+ names(data) <- sapply(data, FUN = function(x) paste(dim(x), collapse = "x"))
+ data
+ }
> data <- rmatrices(mode = "double")
Results
10x10 matrix
> X <- data[["10x10"]]
> gc()
used (Mb) gc trigger (Mb) max used (Mb)
Ncells 5283699 282.2 7916910 422.9 7916910 422.9
Vcells 10537175 80.4 33191153 253.3 53339345 407.0
> probs <- seq(from = 0, to = 1, by = 0.25)
> colStats <- microbenchmark(colQuantiles = colQuantiles(X, probs = probs, na.rm = FALSE), `apply+quantile` = apply(X,
+ MARGIN = 2L, FUN = quantile, probs = probs, na.rm = FALSE), unit = "ms")
> X <- t(X)
> gc()
used (Mb) gc trigger (Mb) max used (Mb)
Ncells 5283507 282.2 7916910 422.9 7916910 422.9
Vcells 10536953 80.4 33191153 253.3 53339345 407.0
> rowStats <- microbenchmark(rowQuantiles = rowQuantiles(X, probs = probs, na.rm = FALSE), `apply+quantile` = apply(X,
+ MARGIN = 1L, FUN = quantile, probs = probs, na.rm = FALSE), unit = "ms")
Table: Benchmarking of colQuantiles() and apply+quantile() on 10x10 data. The top panel shows times in milliseconds and the bottom panel shows relative times.
|
expr |
min |
lq |
mean |
median |
uq |
max |
1 |
colQuantiles |
0.302906 |
0.329424 |
0.3677154 |
0.359026 |
0.3966375 |
0.540448 |
2 |
apply+quantile |
1.364473 |
1.438469 |
1.5741078 |
1.511068 |
1.7432090 |
2.199361 |
|
expr |
min |
lq |
mean |
median |
uq |
max |
1 |
colQuantiles |
1.000000 |
1.000000 |
1.000000 |
1.000000 |
1.000000 |
1.000000 |
2 |
apply+quantile |
4.504609 |
4.366619 |
4.280778 |
4.208797 |
4.394968 |
4.069515 |
Table: Benchmarking of rowQuantiles() and apply+quantile() on 10x10 data (transposed). The top panel shows times in milliseconds and the bottom panel shows relative times.
|
expr |
min |
lq |
mean |
median |
uq |
max |
1 |
rowQuantiles |
0.239440 |
0.258194 |
0.3004935 |
0.2638625 |
0.3232105 |
0.561646 |
2 |
apply+quantile |
1.063889 |
1.103623 |
1.2585152 |
1.1329855 |
1.3459045 |
2.063830 |
|
expr |
min |
lq |
mean |
median |
uq |
max |
1 |
rowQuantiles |
1.000000 |
1.000000 |
1.000000 |
1.000000 |
1.000000 |
1.00000 |
2 |
apply+quantile |
4.443238 |
4.274394 |
4.188162 |
4.293848 |
4.164173 |
3.67461 |
Figure: Benchmarking of colQuantiles() and apply+quantile() on 10x10 data as well as rowQuantiles() and apply+quantile() on the same data transposed. Outliers are displayed as crosses. Times are in milliseconds.
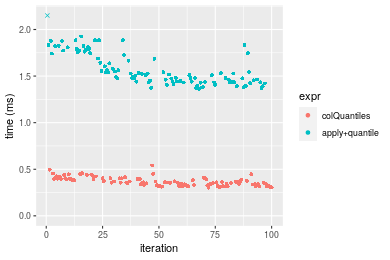
Table: Benchmarking of colQuantiles() and rowQuantiles() on 10x10 data (original and transposed). The top panel shows times in milliseconds and the bottom panel shows relative times.
|
expr |
min |
lq |
mean |
median |
uq |
max |
2 |
rowQuantiles |
239.440 |
258.194 |
300.4935 |
263.8625 |
323.2105 |
561.646 |
1 |
colQuantiles |
302.906 |
329.424 |
367.7154 |
359.0260 |
396.6375 |
540.448 |
|
expr |
min |
lq |
mean |
median |
uq |
max |
2 |
rowQuantiles |
1.00000 |
1.000000 |
1.000000 |
1.000000 |
1.00000 |
1.0000000 |
1 |
colQuantiles |
1.26506 |
1.275878 |
1.223705 |
1.360656 |
1.22718 |
0.9622574 |
Figure: Benchmarking of colQuantiles() and rowQuantiles() on 10x10 data (original and transposed). Outliers are displayed as crosses. Times are in milliseconds.
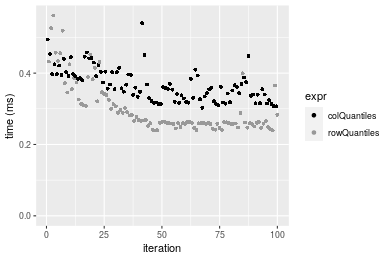
100x100 matrix
> X <- data[["100x100"]]
> gc()
used (Mb) gc trigger (Mb) max used (Mb)
Ncells 5282073 282.1 7916910 422.9 7916910 422.9
Vcells 10153396 77.5 33191153 253.3 53339345 407.0
> probs <- seq(from = 0, to = 1, by = 0.25)
> colStats <- microbenchmark(colQuantiles = colQuantiles(X, probs = probs, na.rm = FALSE), `apply+quantile` = apply(X,
+ MARGIN = 2L, FUN = quantile, probs = probs, na.rm = FALSE), unit = "ms")
> X <- t(X)
> gc()
used (Mb) gc trigger (Mb) max used (Mb)
Ncells 5282061 282.1 7916910 422.9 7916910 422.9
Vcells 10163429 77.6 33191153 253.3 53339345 407.0
> rowStats <- microbenchmark(rowQuantiles = rowQuantiles(X, probs = probs, na.rm = FALSE), `apply+quantile` = apply(X,
+ MARGIN = 1L, FUN = quantile, probs = probs, na.rm = FALSE), unit = "ms")
Table: Benchmarking of colQuantiles() and apply+quantile() on 100x100 data. The top panel shows times in milliseconds and the bottom panel shows relative times.
|
expr |
min |
lq |
mean |
median |
uq |
max |
1 |
colQuantiles |
1.843692 |
1.90874 |
2.24609 |
1.97988 |
2.13335 |
8.8629 |
2 |
apply+quantile |
10.674418 |
10.90321 |
15.60727 |
11.17651 |
12.21271 |
381.8870 |
|
expr |
min |
lq |
mean |
median |
uq |
max |
1 |
colQuantiles |
1.000000 |
1.000000 |
1.000000 |
1.000000 |
1.000000 |
1.00000 |
2 |
apply+quantile |
5.789697 |
5.712257 |
6.948642 |
5.645043 |
5.724664 |
43.08827 |
Table: Benchmarking of rowQuantiles() and apply+quantile() on 100x100 data (transposed). The top panel shows times in milliseconds and the bottom panel shows relative times.
|
expr |
min |
lq |
mean |
median |
uq |
max |
1 |
rowQuantiles |
1.901765 |
1.98259 |
2.19478 |
2.039334 |
2.332585 |
3.054295 |
2 |
apply+quantile |
10.722477 |
11.10321 |
12.93694 |
11.629099 |
14.161271 |
24.969997 |
|
expr |
min |
lq |
mean |
median |
uq |
max |
1 |
rowQuantiles |
1.000000 |
1.000000 |
1.000000 |
1.000000 |
1.000000 |
1.000000 |
2 |
apply+quantile |
5.638171 |
5.600357 |
5.894414 |
5.702402 |
6.071062 |
8.175372 |
Figure: Benchmarking of colQuantiles() and apply+quantile() on 100x100 data as well as rowQuantiles() and apply+quantile() on the same data transposed. Outliers are displayed as crosses. Times are in milliseconds.
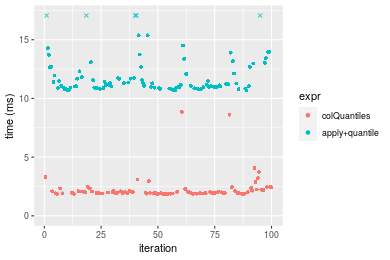
Table: Benchmarking of colQuantiles() and rowQuantiles() on 100x100 data (original and transposed). The top panel shows times in milliseconds and the bottom panel shows relative times.
|
expr |
min |
lq |
mean |
median |
uq |
max |
1 |
colQuantiles |
1.843692 |
1.90874 |
2.24609 |
1.979880 |
2.133350 |
8.862900 |
2 |
rowQuantiles |
1.901765 |
1.98259 |
2.19478 |
2.039334 |
2.332585 |
3.054295 |
|
expr |
min |
lq |
mean |
median |
uq |
max |
1 |
colQuantiles |
1.000000 |
1.000000 |
1.0000000 |
1.000000 |
1.000000 |
1.0000000 |
2 |
rowQuantiles |
1.031498 |
1.038691 |
0.9771561 |
1.030029 |
1.093391 |
0.3446158 |
Figure: Benchmarking of colQuantiles() and rowQuantiles() on 100x100 data (original and transposed). Outliers are displayed as crosses. Times are in milliseconds.
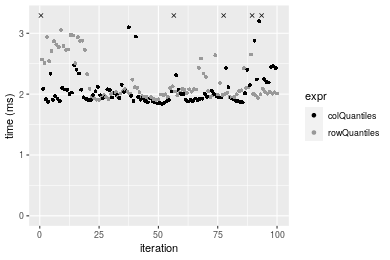
1000x10 matrix
> X <- data[["1000x10"]]
> gc()
used (Mb) gc trigger (Mb) max used (Mb)
Ncells 5282815 282.2 7916910 422.9 7916910 422.9
Vcells 10156922 77.5 33191153 253.3 53339345 407.0
> probs <- seq(from = 0, to = 1, by = 0.25)
> colStats <- microbenchmark(colQuantiles = colQuantiles(X, probs = probs, na.rm = FALSE), `apply+quantile` = apply(X,
+ MARGIN = 2L, FUN = quantile, probs = probs, na.rm = FALSE), unit = "ms")
> X <- t(X)
> gc()
used (Mb) gc trigger (Mb) max used (Mb)
Ncells 5282791 282.2 7916910 422.9 7916910 422.9
Vcells 10166935 77.6 33191153 253.3 53339345 407.0
> rowStats <- microbenchmark(rowQuantiles = rowQuantiles(X, probs = probs, na.rm = FALSE), `apply+quantile` = apply(X,
+ MARGIN = 1L, FUN = quantile, probs = probs, na.rm = FALSE), unit = "ms")
Table: Benchmarking of colQuantiles() and apply+quantile() on 1000x10 data. The top panel shows times in milliseconds and the bottom panel shows relative times.
|
expr |
min |
lq |
mean |
median |
uq |
max |
1 |
colQuantiles |
0.590347 |
0.6104945 |
0.681985 |
0.6246515 |
0.678449 |
1.248694 |
2 |
apply+quantile |
1.476754 |
1.5267405 |
1.687743 |
1.5651400 |
1.762205 |
2.788720 |
|
expr |
min |
lq |
mean |
median |
uq |
max |
1 |
colQuantiles |
1.000000 |
1.000000 |
1.000000 |
1.000000 |
1.000000 |
1.000000 |
2 |
apply+quantile |
2.501502 |
2.500826 |
2.474751 |
2.505621 |
2.597402 |
2.233309 |
Table: Benchmarking of rowQuantiles() and apply+quantile() on 1000x10 data (transposed). The top panel shows times in milliseconds and the bottom panel shows relative times.
|
expr |
min |
lq |
mean |
median |
uq |
max |
1 |
rowQuantiles |
0.626120 |
0.6527315 |
0.736542 |
0.691613 |
0.807727 |
1.219093 |
2 |
apply+quantile |
1.494004 |
1.5361655 |
1.707736 |
1.597339 |
1.789035 |
2.667647 |
|
expr |
min |
lq |
mean |
median |
uq |
max |
1 |
rowQuantiles |
1.000000 |
1.000000 |
1.000000 |
1.000000 |
1.000000 |
1.000000 |
2 |
apply+quantile |
2.386131 |
2.353442 |
2.318586 |
2.309586 |
2.214901 |
2.188223 |
Figure: Benchmarking of colQuantiles() and apply+quantile() on 1000x10 data as well as rowQuantiles() and apply+quantile() on the same data transposed. Outliers are displayed as crosses. Times are in milliseconds.
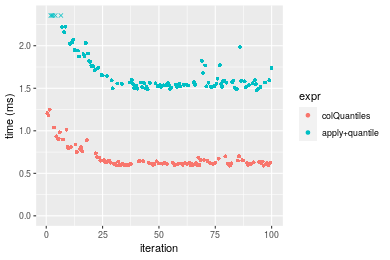
Table: Benchmarking of colQuantiles() and rowQuantiles() on 1000x10 data (original and transposed). The top panel shows times in milliseconds and the bottom panel shows relative times.
|
expr |
min |
lq |
mean |
median |
uq |
max |
1 |
colQuantiles |
590.347 |
610.4945 |
681.985 |
624.6515 |
678.449 |
1248.694 |
2 |
rowQuantiles |
626.120 |
652.7315 |
736.542 |
691.6130 |
807.727 |
1219.093 |
|
expr |
min |
lq |
mean |
median |
uq |
max |
1 |
colQuantiles |
1.000000 |
1.000000 |
1.000000 |
1.000000 |
1.000000 |
1.0000000 |
2 |
rowQuantiles |
1.060597 |
1.069185 |
1.079997 |
1.107198 |
1.190549 |
0.9762944 |
Figure: Benchmarking of colQuantiles() and rowQuantiles() on 1000x10 data (original and transposed). Outliers are displayed as crosses. Times are in milliseconds.
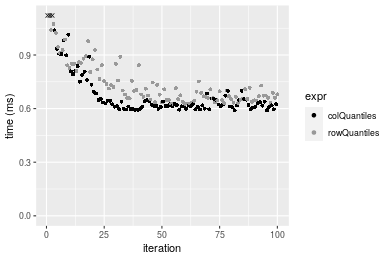
10x1000 matrix
> X <- data[["10x1000"]]
> gc()
used (Mb) gc trigger (Mb) max used (Mb)
Ncells 5282991 282.2 7916910 422.9 7916910 422.9
Vcells 10157622 77.5 33191153 253.3 53339345 407.0
> probs <- seq(from = 0, to = 1, by = 0.25)
> colStats <- microbenchmark(colQuantiles = colQuantiles(X, probs = probs, na.rm = FALSE), `apply+quantile` = apply(X,
+ MARGIN = 2L, FUN = quantile, probs = probs, na.rm = FALSE), unit = "ms")
> X <- t(X)
> gc()
used (Mb) gc trigger (Mb) max used (Mb)
Ncells 5282979 282.2 7916910 422.9 7916910 422.9
Vcells 10167655 77.6 33191153 253.3 53339345 407.0
> rowStats <- microbenchmark(rowQuantiles = rowQuantiles(X, probs = probs, na.rm = FALSE), `apply+quantile` = apply(X,
+ MARGIN = 1L, FUN = quantile, probs = probs, na.rm = FALSE), unit = "ms")
Table: Benchmarking of colQuantiles() and apply+quantile() on 10x1000 data. The top panel shows times in milliseconds and the bottom panel shows relative times.
|
expr |
min |
lq |
mean |
median |
uq |
max |
1 |
colQuantiles |
13.55287 |
14.01786 |
15.7543 |
14.57951 |
16.36505 |
26.8499 |
2 |
apply+quantile |
100.83011 |
104.24423 |
112.1036 |
110.51778 |
117.83936 |
145.3915 |
|
expr |
min |
lq |
mean |
median |
uq |
max |
1 |
colQuantiles |
1.00000 |
1.000000 |
1.000000 |
1.000000 |
1.000000 |
1.000000 |
2 |
apply+quantile |
7.43976 |
7.436527 |
7.115746 |
7.580347 |
7.200672 |
5.414975 |
Table: Benchmarking of rowQuantiles() and apply+quantile() on 10x1000 data (transposed). The top panel shows times in milliseconds and the bottom panel shows relative times.
|
expr |
min |
lq |
mean |
median |
uq |
max |
1 |
rowQuantiles |
13.58450 |
13.81358 |
15.58997 |
14.30125 |
15.59021 |
32.9124 |
2 |
apply+quantile |
99.82761 |
104.50799 |
114.91728 |
112.48729 |
121.21521 |
178.8486 |
|
expr |
min |
lq |
mean |
median |
uq |
max |
1 |
rowQuantiles |
1.00000 |
1.000000 |
1.000000 |
1.000000 |
1.000000 |
1.00000 |
2 |
apply+quantile |
7.34864 |
7.565596 |
7.371232 |
7.865559 |
7.775085 |
5.43408 |
Figure: Benchmarking of colQuantiles() and apply+quantile() on 10x1000 data as well as rowQuantiles() and apply+quantile() on the same data transposed. Outliers are displayed as crosses. Times are in milliseconds.
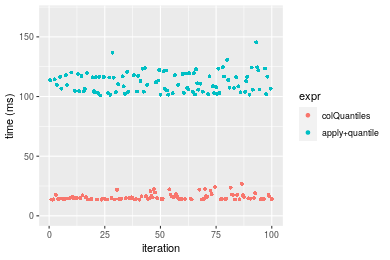
Table: Benchmarking of colQuantiles() and rowQuantiles() on 10x1000 data (original and transposed). The top panel shows times in milliseconds and the bottom panel shows relative times.
|
expr |
min |
lq |
mean |
median |
uq |
max |
2 |
rowQuantiles |
13.58450 |
13.81358 |
15.58997 |
14.30125 |
15.59021 |
32.9124 |
1 |
colQuantiles |
13.55287 |
14.01786 |
15.75430 |
14.57951 |
16.36505 |
26.8499 |
|
expr |
min |
lq |
mean |
median |
uq |
max |
2 |
rowQuantiles |
1.0000000 |
1.000000 |
1.000000 |
1.000000 |
1.0000 |
1.0000000 |
1 |
colQuantiles |
0.9976717 |
1.014788 |
1.010541 |
1.019458 |
1.0497 |
0.8157989 |
Figure: Benchmarking of colQuantiles() and rowQuantiles() on 10x1000 data (original and transposed). Outliers are displayed as crosses. Times are in milliseconds.
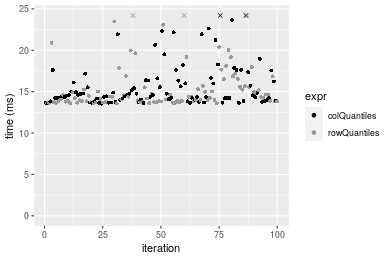
100x1000 matrix
> X <- data[["100x1000"]]
> gc()
used (Mb) gc trigger (Mb) max used (Mb)
Ncells 5283181 282.2 7916910 422.9 7916910 422.9
Vcells 10158119 77.6 33191153 253.3 53339345 407.0
> probs <- seq(from = 0, to = 1, by = 0.25)
> colStats <- microbenchmark(colQuantiles = colQuantiles(X, probs = probs, na.rm = FALSE), `apply+quantile` = apply(X,
+ MARGIN = 2L, FUN = quantile, probs = probs, na.rm = FALSE), unit = "ms")
> X <- t(X)
> gc()
used (Mb) gc trigger (Mb) max used (Mb)
Ncells 5283163 282.2 7916910 422.9 7916910 422.9
Vcells 10258142 78.3 33191153 253.3 53339345 407.0
> rowStats <- microbenchmark(rowQuantiles = rowQuantiles(X, probs = probs, na.rm = FALSE), `apply+quantile` = apply(X,
+ MARGIN = 1L, FUN = quantile, probs = probs, na.rm = FALSE), unit = "ms")
Table: Benchmarking of colQuantiles() and apply+quantile() on 100x1000 data. The top panel shows times in milliseconds and the bottom panel shows relative times.
|
expr |
min |
lq |
mean |
median |
uq |
max |
1 |
colQuantiles |
17.29224 |
17.73178 |
23.86813 |
18.47384 |
21.20117 |
412.5931 |
2 |
apply+quantile |
106.29891 |
109.58439 |
123.38824 |
121.01874 |
130.89134 |
196.2455 |
|
expr |
min |
lq |
mean |
median |
uq |
max |
1 |
colQuantiles |
1.000000 |
1.000000 |
1.000000 |
1.000000 |
1.00000 |
1.0000000 |
2 |
apply+quantile |
6.147205 |
6.180113 |
5.169581 |
6.550816 |
6.17378 |
0.4756392 |
Table: Benchmarking of rowQuantiles() and apply+quantile() on 100x1000 data (transposed). The top panel shows times in milliseconds and the bottom panel shows relative times.
|
expr |
min |
lq |
mean |
median |
uq |
max |
1 |
rowQuantiles |
17.89716 |
18.4045 |
19.64489 |
18.70136 |
20.01506 |
31.27969 |
2 |
apply+quantile |
106.93395 |
111.9668 |
123.09826 |
121.39408 |
131.45883 |
161.34232 |
|
expr |
min |
lq |
mean |
median |
uq |
max |
1 |
rowQuantiles |
1.000000 |
1.000000 |
1.000000 |
1.000000 |
1.000000 |
1.000000 |
2 |
apply+quantile |
5.974913 |
6.083664 |
6.266172 |
6.491189 |
6.567997 |
5.158054 |
Figure: Benchmarking of colQuantiles() and apply+quantile() on 100x1000 data as well as rowQuantiles() and apply+quantile() on the same data transposed. Outliers are displayed as crosses. Times are in milliseconds.
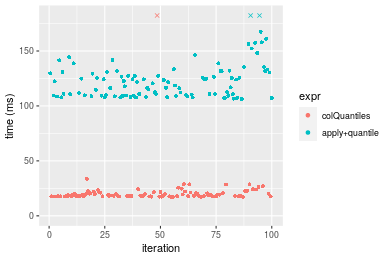
Table: Benchmarking of colQuantiles() and rowQuantiles() on 100x1000 data (original and transposed). The top panel shows times in milliseconds and the bottom panel shows relative times.
|
expr |
min |
lq |
mean |
median |
uq |
max |
1 |
colQuantiles |
17.29224 |
17.73178 |
23.86813 |
18.47384 |
21.20117 |
412.59314 |
2 |
rowQuantiles |
17.89716 |
18.40450 |
19.64489 |
18.70136 |
20.01506 |
31.27969 |
|
expr |
min |
lq |
mean |
median |
uq |
max |
1 |
colQuantiles |
1.000000 |
1.000000 |
1.0000000 |
1.000000 |
1.0000000 |
1.0000000 |
2 |
rowQuantiles |
1.034982 |
1.037939 |
0.8230594 |
1.012316 |
0.9440544 |
0.0758124 |
Figure: Benchmarking of colQuantiles() and rowQuantiles() on 100x1000 data (original and transposed). Outliers are displayed as crosses. Times are in milliseconds.
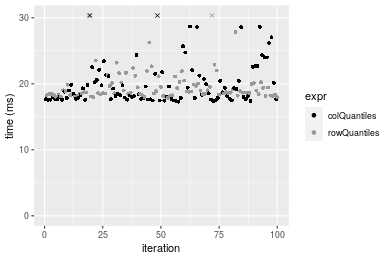
1000x100 matrix
> X <- data[["1000x100"]]
> gc()
used (Mb) gc trigger (Mb) max used (Mb)
Ncells 5283373 282.2 7916910 422.9 7916910 422.9
Vcells 10158745 77.6 33191153 253.3 53339345 407.0
> probs <- seq(from = 0, to = 1, by = 0.25)
> colStats <- microbenchmark(colQuantiles = colQuantiles(X, probs = probs, na.rm = FALSE), `apply+quantile` = apply(X,
+ MARGIN = 2L, FUN = quantile, probs = probs, na.rm = FALSE), unit = "ms")
> X <- t(X)
> gc()
used (Mb) gc trigger (Mb) max used (Mb)
Ncells 5283355 282.2 7916910 422.9 7916910 422.9
Vcells 10258768 78.3 33191153 253.3 53339345 407.0
> rowStats <- microbenchmark(rowQuantiles = rowQuantiles(X, probs = probs, na.rm = FALSE), `apply+quantile` = apply(X,
+ MARGIN = 1L, FUN = quantile, probs = probs, na.rm = FALSE), unit = "ms")
Table: Benchmarking of colQuantiles() and apply+quantile() on 1000x100 data. The top panel shows times in milliseconds and the bottom panel shows relative times.
|
expr |
min |
lq |
mean |
median |
uq |
max |
1 |
colQuantiles |
4.951451 |
5.02867 |
5.23471 |
5.110415 |
5.16578 |
8.446151 |
2 |
apply+quantile |
14.330868 |
14.50606 |
15.34585 |
14.602870 |
14.90682 |
25.848297 |
|
expr |
min |
lq |
mean |
median |
uq |
max |
1 |
colQuantiles |
1.000000 |
1.000000 |
1.000000 |
1.000000 |
1.000000 |
1.000000 |
2 |
apply+quantile |
2.894276 |
2.884672 |
2.931556 |
2.857473 |
2.885687 |
3.060364 |
Table: Benchmarking of rowQuantiles() and apply+quantile() on 1000x100 data (transposed). The top panel shows times in milliseconds and the bottom panel shows relative times.
|
expr |
min |
lq |
mean |
median |
uq |
max |
1 |
rowQuantiles |
5.324899 |
5.445932 |
5.764332 |
5.531068 |
5.691759 |
14.66775 |
2 |
apply+quantile |
14.449878 |
14.704087 |
15.582007 |
14.877622 |
15.320830 |
26.38245 |
|
expr |
min |
lq |
mean |
median |
uq |
max |
1 |
rowQuantiles |
1.000000 |
1.000000 |
1.000000 |
1.000000 |
1.000000 |
1.00000 |
2 |
apply+quantile |
2.713644 |
2.700013 |
2.703177 |
2.689828 |
2.691756 |
1.79867 |
Figure: Benchmarking of colQuantiles() and apply+quantile() on 1000x100 data as well as rowQuantiles() and apply+quantile() on the same data transposed. Outliers are displayed as crosses. Times are in milliseconds.
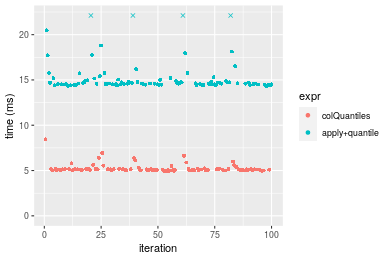
Table: Benchmarking of colQuantiles() and rowQuantiles() on 1000x100 data (original and transposed). The top panel shows times in milliseconds and the bottom panel shows relative times.
|
expr |
min |
lq |
mean |
median |
uq |
max |
1 |
colQuantiles |
4.951451 |
5.028670 |
5.234710 |
5.110415 |
5.165780 |
8.446151 |
2 |
rowQuantiles |
5.324899 |
5.445932 |
5.764332 |
5.531068 |
5.691759 |
14.667752 |
|
expr |
min |
lq |
mean |
median |
uq |
max |
1 |
colQuantiles |
1.000000 |
1.000000 |
1.000000 |
1.000000 |
1.00000 |
1.00000 |
2 |
rowQuantiles |
1.075422 |
1.082977 |
1.101175 |
1.082313 |
1.10182 |
1.73662 |
Figure: Benchmarking of colQuantiles() and rowQuantiles() on 1000x100 data (original and transposed). Outliers are displayed as crosses. Times are in milliseconds.
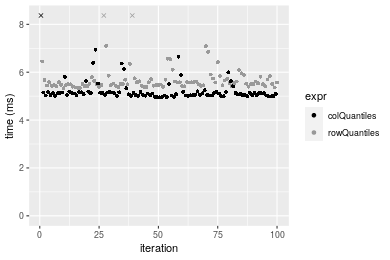
Appendix
R version 4.1.1 Patched (2021-08-10 r80727)
Platform: x86_64-pc-linux-gnu (64-bit)
Running under: Ubuntu 18.04.5 LTS
Matrix products: default
BLAS: /home/hb/software/R-devel/R-4-1-branch/lib/R/lib/libRblas.so
LAPACK: /home/hb/software/R-devel/R-4-1-branch/lib/R/lib/libRlapack.so
locale:
[1] LC_CTYPE=en_US.UTF-8 LC_NUMERIC=C
[3] LC_TIME=en_US.UTF-8 LC_COLLATE=en_US.UTF-8
[5] LC_MONETARY=en_US.UTF-8 LC_MESSAGES=en_US.UTF-8
[7] LC_PAPER=en_US.UTF-8 LC_NAME=C
[9] LC_ADDRESS=C LC_TELEPHONE=C
[11] LC_MEASUREMENT=en_US.UTF-8 LC_IDENTIFICATION=C
attached base packages:
[1] stats graphics grDevices utils datasets methods base
other attached packages:
[1] microbenchmark_1.4-7 matrixStats_0.60.0 ggplot2_3.3.5
[4] knitr_1.33 R.devices_2.17.0 R.utils_2.10.1
[7] R.oo_1.24.0 R.methodsS3_1.8.1-9001 history_0.0.1-9000
loaded via a namespace (and not attached):
[1] Biobase_2.52.0 httr_1.4.2 splines_4.1.1
[4] bit64_4.0.5 network_1.17.1 assertthat_0.2.1
[7] highr_0.9 stats4_4.1.1 blob_1.2.2
[10] GenomeInfoDbData_1.2.6 robustbase_0.93-8 pillar_1.6.2
[13] RSQLite_2.2.8 lattice_0.20-44 glue_1.4.2
[16] digest_0.6.27 XVector_0.32.0 colorspace_2.0-2
[19] Matrix_1.3-4 XML_3.99-0.7 pkgconfig_2.0.3
[22] zlibbioc_1.38.0 genefilter_1.74.0 purrr_0.3.4
[25] ergm_4.1.2 xtable_1.8-4 scales_1.1.1
[28] tibble_3.1.4 annotate_1.70.0 KEGGREST_1.32.0
[31] farver_2.1.0 generics_0.1.0 IRanges_2.26.0
[34] ellipsis_0.3.2 cachem_1.0.6 withr_2.4.2
[37] BiocGenerics_0.38.0 mime_0.11 survival_3.2-13
[40] magrittr_2.0.1 crayon_1.4.1 statnet.common_4.5.0
[43] memoise_2.0.0 laeken_0.5.1 fansi_0.5.0
[46] R.cache_0.15.0 MASS_7.3-54 R.rsp_0.44.0
[49] progressr_0.8.0 tools_4.1.1 lifecycle_1.0.0
[52] S4Vectors_0.30.0 trust_0.1-8 munsell_0.5.0
[55] tabby_0.0.1-9001 AnnotationDbi_1.54.1 Biostrings_2.60.2
[58] compiler_4.1.1 GenomeInfoDb_1.28.1 rlang_0.4.11
[61] grid_4.1.1 RCurl_1.98-1.4 cwhmisc_6.6
[64] rstudioapi_0.13 rappdirs_0.3.3 startup_0.15.0
[67] labeling_0.4.2 bitops_1.0-7 base64enc_0.1-3
[70] boot_1.3-28 gtable_0.3.0 DBI_1.1.1
[73] markdown_1.1 R6_2.5.1 lpSolveAPI_5.5.2.0-17.7
[76] rle_0.9.2 dplyr_1.0.7 fastmap_1.1.0
[79] bit_4.0.4 utf8_1.2.2 parallel_4.1.1
[82] Rcpp_1.0.7 vctrs_0.3.8 png_0.1-7
[85] DEoptimR_1.0-9 tidyselect_1.1.1 xfun_0.25
[88] coda_0.19-4
Total processing time was 1.25 mins.
Reproducibility
To reproduce this report, do:
html <- matrixStats:::benchmark('colQuantiles')
Copyright Henrik Bengtsson. Last updated on 2021-08-25 22:26:54 (+0200 UTC). Powered by RSP.