colLogSumExps() and rowLogSumExps() benchmarks
This report benchmark the performance of colLogSumExps() and rowLogSumExps() against alternative methods.
Alternative methods
- apply() + matrixStats::logSumExp()
- apply() + logSumExp0()
where
> logSumExp0 <- function(lx, ...) {
+ iMax <- which.max(lx)
+ log1p(sum(exp(lx[-iMax] - lx[iMax]))) + lx[iMax]
+ }
Data
> rmatrix <- function(nrow, ncol, mode = c("logical", "double", "integer", "index"), range = c(-100,
+ +100), na_prob = 0) {
+ mode <- match.arg(mode)
+ n <- nrow * ncol
+ if (mode == "logical") {
+ x <- sample(c(FALSE, TRUE), size = n, replace = TRUE)
+ } else if (mode == "index") {
+ x <- seq_len(n)
+ mode <- "integer"
+ } else {
+ x <- runif(n, min = range[1], max = range[2])
+ }
+ storage.mode(x) <- mode
+ if (na_prob > 0)
+ x[sample(n, size = na_prob * n)] <- NA
+ dim(x) <- c(nrow, ncol)
+ x
+ }
> rmatrices <- function(scale = 10, seed = 1, ...) {
+ set.seed(seed)
+ data <- list()
+ data[[1]] <- rmatrix(nrow = scale * 1, ncol = scale * 1, ...)
+ data[[2]] <- rmatrix(nrow = scale * 10, ncol = scale * 10, ...)
+ data[[3]] <- rmatrix(nrow = scale * 100, ncol = scale * 1, ...)
+ data[[4]] <- t(data[[3]])
+ data[[5]] <- rmatrix(nrow = scale * 10, ncol = scale * 100, ...)
+ data[[6]] <- t(data[[5]])
+ names(data) <- sapply(data, FUN = function(x) paste(dim(x), collapse = "x"))
+ data
+ }
> data <- rmatrices(mode = "double")
Results
10x10 matrix
> X <- data[["10x10"]]
> gc()
used (Mb) gc trigger (Mb) max used (Mb)
Ncells 5242264 280.0 7916910 422.9 7916910 422.9
Vcells 10188779 77.8 33191153 253.3 53339345 407.0
> colStats <- microbenchmark(colLogSumExps = colLogSumExps(X, na.rm = FALSE), `apply+logSumExp` = apply(X,
+ MARGIN = 2L, FUN = logSumExp, na.rm = FALSE), `apply+logSumExp0` = apply(X, MARGIN = 2L, FUN = logSumExp0,
+ na.rm = FALSE), unit = "ms")
> X <- t(X)
> gc()
used (Mb) gc trigger (Mb) max used (Mb)
Ncells 5228192 279.3 7916910 422.9 7916910 422.9
Vcells 10142210 77.4 33191153 253.3 53339345 407.0
> rowStats <- microbenchmark(rowLogSumExps = rowLogSumExps(X, na.rm = FALSE), `apply+logSumExp` = apply(X,
+ MARGIN = 1L, FUN = logSumExp, na.rm = FALSE), `apply+logSumExp0` = apply(X, MARGIN = 1L, FUN = logSumExp0,
+ na.rm = FALSE), unit = "ms")
Table: Benchmarking of colLogSumExps(), apply+logSumExp() and apply+logSumExp0() on 10x10 data. The top panel shows times in milliseconds and the bottom panel shows relative times.
|
expr |
min |
lq |
mean |
median |
uq |
max |
1 |
colLogSumExps |
0.008133 |
0.0090895 |
0.0104243 |
0.0104435 |
0.0109565 |
0.032976 |
2 |
apply+logSumExp |
0.047248 |
0.0507385 |
0.0535950 |
0.0527455 |
0.0533990 |
0.155811 |
3 |
apply+logSumExp0 |
0.064913 |
0.0685595 |
0.0716377 |
0.0705830 |
0.0714225 |
0.174637 |
|
expr |
min |
lq |
mean |
median |
uq |
max |
1 |
colLogSumExps |
1.000000 |
1.000000 |
1.000000 |
1.000000 |
1.000000 |
1.000000 |
2 |
apply+logSumExp |
5.809418 |
5.582100 |
5.141343 |
5.050558 |
4.873728 |
4.724982 |
3 |
apply+logSumExp0 |
7.981434 |
7.542714 |
6.872169 |
6.758558 |
6.518733 |
5.295882 |
Table: Benchmarking of rowLogSumExps(), apply+logSumExp() and apply+logSumExp0() on 10x10 data (transposed). The top panel shows times in milliseconds and the bottom panel shows relative times.
|
expr |
min |
lq |
mean |
median |
uq |
max |
1 |
rowLogSumExps |
0.007400 |
0.0081525 |
0.0094867 |
0.0093865 |
0.0099785 |
0.028806 |
2 |
apply+logSumExp |
0.047769 |
0.0521430 |
0.0536802 |
0.0528420 |
0.0533980 |
0.085639 |
3 |
apply+logSumExp0 |
0.065200 |
0.0696110 |
0.0724195 |
0.0713380 |
0.0721935 |
0.155864 |
|
expr |
min |
lq |
mean |
median |
uq |
max |
1 |
rowLogSumExps |
1.000000 |
1.000000 |
1.000000 |
1.000000 |
1.000000 |
1.000000 |
2 |
apply+logSumExp |
6.455270 |
6.395952 |
5.658454 |
5.629574 |
5.351305 |
2.972957 |
3 |
apply+logSumExp0 |
8.810811 |
8.538608 |
7.633780 |
7.600064 |
7.234905 |
5.410817 |
Figure: Benchmarking of colLogSumExps(), apply+logSumExp() and apply+logSumExp0() on 10x10 data as well as rowLogSumExps(), apply+logSumExp() and apply+logSumExp0() on the same data transposed. Outliers are displayed as crosses. Times are in milliseconds.
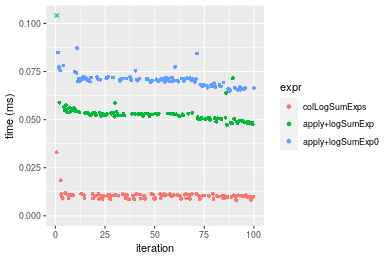
Table: Benchmarking of colLogSumExps() and rowLogSumExps() on 10x10 data (original and transposed). The top panel shows times in milliseconds and the bottom panel shows relative times.
|
expr |
min |
lq |
mean |
median |
uq |
max |
2 |
rowLogSumExps |
7.400 |
8.1525 |
9.48672 |
9.3865 |
9.9785 |
28.806 |
1 |
colLogSumExps |
8.133 |
9.0895 |
10.42432 |
10.4435 |
10.9565 |
32.976 |
|
expr |
min |
lq |
mean |
median |
uq |
max |
2 |
rowLogSumExps |
1.000000 |
1.000000 |
1.000000 |
1.000000 |
1.000000 |
1.000000 |
1 |
colLogSumExps |
1.099054 |
1.114934 |
1.098833 |
1.112608 |
1.098011 |
1.144761 |
Figure: Benchmarking of colLogSumExps() and rowLogSumExps() on 10x10 data (original and transposed). Outliers are displayed as crosses. Times are in milliseconds.
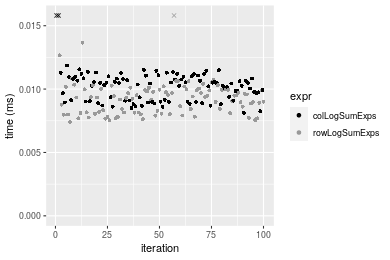
100x100 matrix
> X <- data[["100x100"]]
> gc()
used (Mb) gc trigger (Mb) max used (Mb)
Ncells 5226752 279.2 7916910 422.9 7916910 422.9
Vcells 9758795 74.5 33191153 253.3 53339345 407.0
> colStats <- microbenchmark(colLogSumExps = colLogSumExps(X, na.rm = FALSE), `apply+logSumExp` = apply(X,
+ MARGIN = 2L, FUN = logSumExp, na.rm = FALSE), `apply+logSumExp0` = apply(X, MARGIN = 2L, FUN = logSumExp0,
+ na.rm = FALSE), unit = "ms")
> X <- t(X)
> gc()
used (Mb) gc trigger (Mb) max used (Mb)
Ncells 5226746 279.2 7916910 422.9 7916910 422.9
Vcells 9768838 74.6 33191153 253.3 53339345 407.0
> rowStats <- microbenchmark(rowLogSumExps = rowLogSumExps(X, na.rm = FALSE), `apply+logSumExp` = apply(X,
+ MARGIN = 1L, FUN = logSumExp, na.rm = FALSE), `apply+logSumExp0` = apply(X, MARGIN = 1L, FUN = logSumExp0,
+ na.rm = FALSE), unit = "ms")
Table: Benchmarking of colLogSumExps(), apply+logSumExp() and apply+logSumExp0() on 100x100 data. The top panel shows times in milliseconds and the bottom panel shows relative times.
|
expr |
min |
lq |
mean |
median |
uq |
max |
1 |
colLogSumExps |
0.147286 |
0.1533570 |
0.1748630 |
0.1615145 |
0.1881190 |
0.318889 |
2 |
apply+logSumExp |
0.374943 |
0.3857225 |
0.4386438 |
0.4127325 |
0.4663460 |
0.766932 |
3 |
apply+logSumExp0 |
0.532599 |
0.5451135 |
0.6201473 |
0.5709585 |
0.6800395 |
1.043200 |
|
expr |
min |
lq |
mean |
median |
uq |
max |
1 |
colLogSumExps |
1.000000 |
1.000000 |
1.000000 |
1.000000 |
1.000000 |
1.000000 |
2 |
apply+logSumExp |
2.545680 |
2.515193 |
2.508500 |
2.555390 |
2.478995 |
2.405012 |
3 |
apply+logSumExp0 |
3.616087 |
3.554539 |
3.546476 |
3.535029 |
3.614943 |
3.271358 |
Table: Benchmarking of rowLogSumExps(), apply+logSumExp() and apply+logSumExp0() on 100x100 data (transposed). The top panel shows times in milliseconds and the bottom panel shows relative times.
|
expr |
min |
lq |
mean |
median |
uq |
max |
1 |
rowLogSumExps |
0.151541 |
0.1609810 |
0.1803268 |
0.1699135 |
0.192550 |
0.266127 |
2 |
apply+logSumExp |
0.375183 |
0.3909565 |
0.4443629 |
0.4233270 |
0.470347 |
0.679589 |
3 |
apply+logSumExp0 |
0.534134 |
0.5546200 |
0.6243859 |
0.5808370 |
0.671827 |
1.073041 |
|
expr |
min |
lq |
mean |
median |
uq |
max |
1 |
rowLogSumExps |
1.000000 |
1.000000 |
1.000000 |
1.000000 |
1.000000 |
1.000000 |
2 |
apply+logSumExp |
2.475785 |
2.428588 |
2.464208 |
2.491427 |
2.442727 |
2.553627 |
3 |
apply+logSumExp0 |
3.524683 |
3.445251 |
3.462523 |
3.418428 |
3.489104 |
4.032064 |
Figure: Benchmarking of colLogSumExps(), apply+logSumExp() and apply+logSumExp0() on 100x100 data as well as rowLogSumExps(), apply+logSumExp() and apply+logSumExp0() on the same data transposed. Outliers are displayed as crosses. Times are in milliseconds.
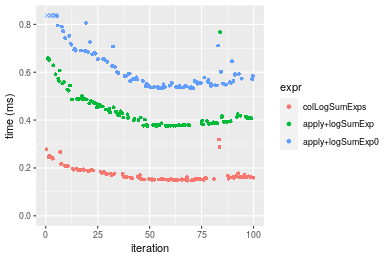
Table: Benchmarking of colLogSumExps() and rowLogSumExps() on 100x100 data (original and transposed). The top panel shows times in milliseconds and the bottom panel shows relative times.
|
expr |
min |
lq |
mean |
median |
uq |
max |
1 |
colLogSumExps |
147.286 |
153.357 |
174.8630 |
161.5145 |
188.119 |
318.889 |
2 |
rowLogSumExps |
151.541 |
160.981 |
180.3268 |
169.9135 |
192.550 |
266.127 |
|
expr |
min |
lq |
mean |
median |
uq |
max |
1 |
colLogSumExps |
1.000000 |
1.000000 |
1.000000 |
1.000000 |
1.000000 |
1.0000000 |
2 |
rowLogSumExps |
1.028889 |
1.049714 |
1.031247 |
1.052002 |
1.023554 |
0.8345443 |
Figure: Benchmarking of colLogSumExps() and rowLogSumExps() on 100x100 data (original and transposed). Outliers are displayed as crosses. Times are in milliseconds.
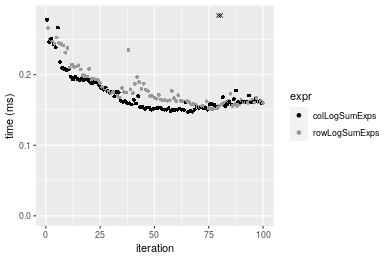
1000x10 matrix
> X <- data[["1000x10"]]
> gc()
used (Mb) gc trigger (Mb) max used (Mb)
Ncells 5227507 279.2 7916910 422.9 7916910 422.9
Vcells 9762590 74.5 33191153 253.3 53339345 407.0
> colStats <- microbenchmark(colLogSumExps = colLogSumExps(X, na.rm = FALSE), `apply+logSumExp` = apply(X,
+ MARGIN = 2L, FUN = logSumExp, na.rm = FALSE), `apply+logSumExp0` = apply(X, MARGIN = 2L, FUN = logSumExp0,
+ na.rm = FALSE), unit = "ms")
> X <- t(X)
> gc()
used (Mb) gc trigger (Mb) max used (Mb)
Ncells 5227495 279.2 7916910 422.9 7916910 422.9
Vcells 9772623 74.6 33191153 253.3 53339345 407.0
> rowStats <- microbenchmark(rowLogSumExps = rowLogSumExps(X, na.rm = FALSE), `apply+logSumExp` = apply(X,
+ MARGIN = 1L, FUN = logSumExp, na.rm = FALSE), `apply+logSumExp0` = apply(X, MARGIN = 1L, FUN = logSumExp0,
+ na.rm = FALSE), unit = "ms")
Table: Benchmarking of colLogSumExps(), apply+logSumExp() and apply+logSumExp0() on 1000x10 data. The top panel shows times in milliseconds and the bottom panel shows relative times.
|
expr |
min |
lq |
mean |
median |
uq |
max |
1 |
colLogSumExps |
0.148651 |
0.1647045 |
0.1906819 |
0.1847195 |
0.2085615 |
0.350757 |
2 |
apply+logSumExp |
0.227003 |
0.2507095 |
0.2880074 |
0.2765740 |
0.3024020 |
0.486887 |
3 |
apply+logSumExp0 |
0.269485 |
0.3098875 |
0.3494949 |
0.3436425 |
0.3780010 |
0.480715 |
|
expr |
min |
lq |
mean |
median |
uq |
max |
1 |
colLogSumExps |
1.000000 |
1.000000 |
1.000000 |
1.000000 |
1.000000 |
1.000000 |
2 |
apply+logSumExp |
1.527087 |
1.522178 |
1.510408 |
1.497265 |
1.449942 |
1.388103 |
3 |
apply+logSumExp0 |
1.812870 |
1.881476 |
1.832869 |
1.860348 |
1.812420 |
1.370507 |
Table: Benchmarking of rowLogSumExps(), apply+logSumExp() and apply+logSumExp0() on 1000x10 data (transposed). The top panel shows times in milliseconds and the bottom panel shows relative times.
|
expr |
min |
lq |
mean |
median |
uq |
max |
1 |
rowLogSumExps |
0.148117 |
0.1623880 |
0.1906410 |
0.1862295 |
0.2183835 |
0.266496 |
2 |
apply+logSumExp |
0.216779 |
0.2381875 |
0.2746292 |
0.2602685 |
0.3046930 |
0.471987 |
3 |
apply+logSumExp0 |
0.261497 |
0.2902070 |
0.3273246 |
0.3186795 |
0.3461150 |
0.478835 |
|
expr |
min |
lq |
mean |
median |
uq |
max |
1 |
rowLogSumExps |
1.000000 |
1.000000 |
1.000000 |
1.000000 |
1.000000 |
1.000000 |
2 |
apply+logSumExp |
1.463566 |
1.466780 |
1.440557 |
1.397569 |
1.395220 |
1.771085 |
3 |
apply+logSumExp0 |
1.765476 |
1.787121 |
1.716968 |
1.711219 |
1.584895 |
1.796781 |
Figure: Benchmarking of colLogSumExps(), apply+logSumExp() and apply+logSumExp0() on 1000x10 data as well as rowLogSumExps(), apply+logSumExp() and apply+logSumExp0() on the same data transposed. Outliers are displayed as crosses. Times are in milliseconds.
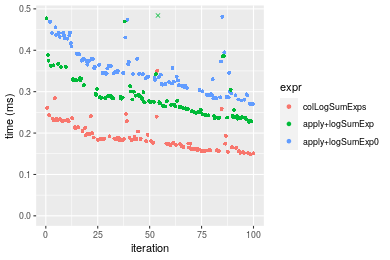
Table: Benchmarking of colLogSumExps() and rowLogSumExps() on 1000x10 data (original and transposed). The top panel shows times in milliseconds and the bottom panel shows relative times.
|
expr |
min |
lq |
mean |
median |
uq |
max |
1 |
colLogSumExps |
148.651 |
164.7045 |
190.6819 |
184.7195 |
208.5615 |
350.757 |
2 |
rowLogSumExps |
148.117 |
162.3880 |
190.6410 |
186.2295 |
218.3835 |
266.496 |
|
expr |
min |
lq |
mean |
median |
uq |
max |
1 |
colLogSumExps |
1.0000000 |
1.0000000 |
1.0000000 |
1.000000 |
1.000000 |
1.0000000 |
2 |
rowLogSumExps |
0.9964077 |
0.9859354 |
0.9997857 |
1.008175 |
1.047094 |
0.7597739 |
Figure: Benchmarking of colLogSumExps() and rowLogSumExps() on 1000x10 data (original and transposed). Outliers are displayed as crosses. Times are in milliseconds.
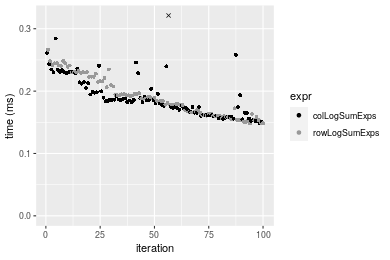
10x1000 matrix
> X <- data[["10x1000"]]
> gc()
used (Mb) gc trigger (Mb) max used (Mb)
Ncells 5227714 279.2 7916910 422.9 7916910 422.9
Vcells 9763394 74.5 33191153 253.3 53339345 407.0
> colStats <- microbenchmark(colLogSumExps = colLogSumExps(X, na.rm = FALSE), `apply+logSumExp` = apply(X,
+ MARGIN = 2L, FUN = logSumExp, na.rm = FALSE), `apply+logSumExp0` = apply(X, MARGIN = 2L, FUN = logSumExp0,
+ na.rm = FALSE), unit = "ms")
> X <- t(X)
> gc()
used (Mb) gc trigger (Mb) max used (Mb)
Ncells 5227708 279.2 7916910 422.9 7916910 422.9
Vcells 9773437 74.6 33191153 253.3 53339345 407.0
> rowStats <- microbenchmark(rowLogSumExps = rowLogSumExps(X, na.rm = FALSE), `apply+logSumExp` = apply(X,
+ MARGIN = 1L, FUN = logSumExp, na.rm = FALSE), `apply+logSumExp0` = apply(X, MARGIN = 1L, FUN = logSumExp0,
+ na.rm = FALSE), unit = "ms")
Table: Benchmarking of colLogSumExps(), apply+logSumExp() and apply+logSumExp0() on 10x1000 data. The top panel shows times in milliseconds and the bottom panel shows relative times.
|
expr |
min |
lq |
mean |
median |
uq |
max |
1 |
colLogSumExps |
0.182868 |
0.198522 |
0.2186889 |
0.206460 |
0.2248295 |
0.425178 |
2 |
apply+logSumExp |
1.811853 |
1.978557 |
2.1914504 |
2.065537 |
2.1991665 |
4.675994 |
3 |
apply+logSumExp0 |
3.031848 |
3.310961 |
3.8202764 |
3.474716 |
3.6859950 |
12.717593 |
|
expr |
min |
lq |
mean |
median |
uq |
max |
1 |
colLogSumExps |
1.000000 |
1.000000 |
1.00000 |
1.00000 |
1.000000 |
1.00000 |
2 |
apply+logSumExp |
9.907983 |
9.966439 |
10.02086 |
10.00454 |
9.781486 |
10.99773 |
3 |
apply+logSumExp0 |
16.579434 |
16.678058 |
17.46900 |
16.82997 |
16.394624 |
29.91122 |
Table: Benchmarking of rowLogSumExps(), apply+logSumExp() and apply+logSumExp0() on 10x1000 data (transposed). The top panel shows times in milliseconds and the bottom panel shows relative times.
|
expr |
min |
lq |
mean |
median |
uq |
max |
1 |
rowLogSumExps |
0.177854 |
0.2003505 |
0.2230796 |
0.2116575 |
0.2355875 |
0.378667 |
2 |
apply+logSumExp |
1.798603 |
1.9887350 |
2.1438652 |
2.0944100 |
2.2260755 |
3.235006 |
3 |
apply+logSumExp0 |
3.021550 |
3.3278055 |
3.5985985 |
3.4443350 |
3.6162465 |
8.963276 |
|
expr |
min |
lq |
mean |
median |
uq |
max |
1 |
rowLogSumExps |
1.00000 |
1.000000 |
1.000000 |
1.000000 |
1.000000 |
1.000000 |
2 |
apply+logSumExp |
10.11281 |
9.926279 |
9.610316 |
9.895279 |
9.449039 |
8.543142 |
3 |
apply+logSumExp0 |
16.98893 |
16.609919 |
16.131456 |
16.273154 |
15.349908 |
23.670602 |
Figure: Benchmarking of colLogSumExps(), apply+logSumExp() and apply+logSumExp0() on 10x1000 data as well as rowLogSumExps(), apply+logSumExp() and apply+logSumExp0() on the same data transposed. Outliers are displayed as crosses. Times are in milliseconds.
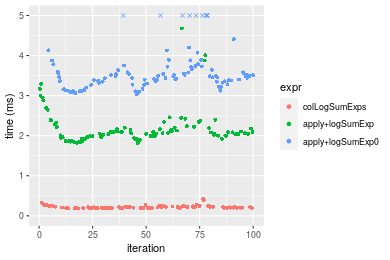
Table: Benchmarking of colLogSumExps() and rowLogSumExps() on 10x1000 data (original and transposed). The top panel shows times in milliseconds and the bottom panel shows relative times.
|
expr |
min |
lq |
mean |
median |
uq |
max |
1 |
colLogSumExps |
182.868 |
198.5220 |
218.6889 |
206.4600 |
224.8295 |
425.178 |
2 |
rowLogSumExps |
177.854 |
200.3505 |
223.0796 |
211.6575 |
235.5875 |
378.667 |
|
expr |
min |
lq |
mean |
median |
uq |
max |
1 |
colLogSumExps |
1.0000000 |
1.000000 |
1.000000 |
1.000000 |
1.00000 |
1.0000000 |
2 |
rowLogSumExps |
0.9725813 |
1.009211 |
1.020077 |
1.025174 |
1.04785 |
0.8906082 |
Figure: Benchmarking of colLogSumExps() and rowLogSumExps() on 10x1000 data (original and transposed). Outliers are displayed as crosses. Times are in milliseconds.
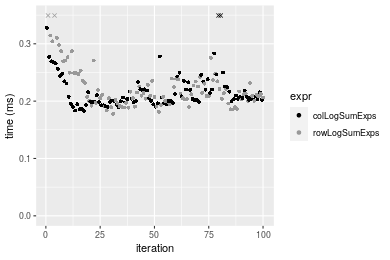
100x1000 matrix
> X <- data[["100x1000"]]
> gc()
used (Mb) gc trigger (Mb) max used (Mb)
Ncells 5227930 279.3 7916910 422.9 7916910 422.9
Vcells 9763989 74.5 33191153 253.3 53339345 407.0
> colStats <- microbenchmark(colLogSumExps = colLogSumExps(X, na.rm = FALSE), `apply+logSumExp` = apply(X,
+ MARGIN = 2L, FUN = logSumExp, na.rm = FALSE), `apply+logSumExp0` = apply(X, MARGIN = 2L, FUN = logSumExp0,
+ na.rm = FALSE), unit = "ms")
> X <- t(X)
> gc()
used (Mb) gc trigger (Mb) max used (Mb)
Ncells 5227912 279.3 7916910 422.9 7916910 422.9
Vcells 9864012 75.3 33191153 253.3 53339345 407.0
> rowStats <- microbenchmark(rowLogSumExps = rowLogSumExps(X, na.rm = FALSE), `apply+logSumExp` = apply(X,
+ MARGIN = 1L, FUN = logSumExp, na.rm = FALSE), `apply+logSumExp0` = apply(X, MARGIN = 1L, FUN = logSumExp0,
+ na.rm = FALSE), unit = "ms")
Table: Benchmarking of colLogSumExps(), apply+logSumExp() and apply+logSumExp0() on 100x1000 data. The top panel shows times in milliseconds and the bottom panel shows relative times.
|
expr |
min |
lq |
mean |
median |
uq |
max |
1 |
colLogSumExps |
1.537931 |
1.665246 |
1.759681 |
1.704182 |
1.837969 |
2.555003 |
2 |
apply+logSumExp |
3.701825 |
4.101192 |
4.849556 |
4.249950 |
4.569222 |
26.022281 |
3 |
apply+logSumExp0 |
5.369943 |
5.892519 |
6.522110 |
6.149206 |
6.676341 |
28.776985 |
|
expr |
min |
lq |
mean |
median |
uq |
max |
1 |
colLogSumExps |
1.000000 |
1.000000 |
1.000000 |
1.000000 |
1.000000 |
1.00000 |
2 |
apply+logSumExp |
2.407016 |
2.462814 |
2.755929 |
2.493836 |
2.486017 |
10.18483 |
3 |
apply+logSumExp0 |
3.491667 |
3.538527 |
3.706415 |
3.608304 |
3.632455 |
11.26299 |
Table: Benchmarking of rowLogSumExps(), apply+logSumExp() and apply+logSumExp0() on 100x1000 data (transposed). The top panel shows times in milliseconds and the bottom panel shows relative times.
|
expr |
min |
lq |
mean |
median |
uq |
max |
1 |
rowLogSumExps |
1.580767 |
1.732992 |
2.018422 |
1.765429 |
1.823624 |
21.395092 |
2 |
apply+logSumExp |
3.719148 |
4.072647 |
4.282412 |
4.166019 |
4.295800 |
5.740941 |
3 |
apply+logSumExp0 |
5.225207 |
5.850327 |
6.585593 |
5.939724 |
6.127233 |
29.342143 |
|
expr |
min |
lq |
mean |
median |
uq |
max |
1 |
rowLogSumExps |
1.000000 |
1.000000 |
1.000000 |
1.000000 |
1.000000 |
1.0000000 |
2 |
apply+logSumExp |
2.352749 |
2.350067 |
2.121663 |
2.359777 |
2.355639 |
0.2683298 |
3 |
apply+logSumExp0 |
3.305488 |
3.375853 |
3.262744 |
3.364465 |
3.359921 |
1.3714427 |
Figure: Benchmarking of colLogSumExps(), apply+logSumExp() and apply+logSumExp0() on 100x1000 data as well as rowLogSumExps(), apply+logSumExp() and apply+logSumExp0() on the same data transposed. Outliers are displayed as crosses. Times are in milliseconds.
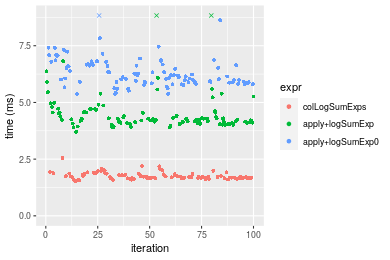
Table: Benchmarking of colLogSumExps() and rowLogSumExps() on 100x1000 data (original and transposed). The top panel shows times in milliseconds and the bottom panel shows relative times.
|
expr |
min |
lq |
mean |
median |
uq |
max |
1 |
colLogSumExps |
1.537931 |
1.665246 |
1.759681 |
1.704182 |
1.837969 |
2.555003 |
2 |
rowLogSumExps |
1.580767 |
1.732992 |
2.018422 |
1.765429 |
1.823624 |
21.395092 |
|
expr |
min |
lq |
mean |
median |
uq |
max |
1 |
colLogSumExps |
1.000000 |
1.000000 |
1.000000 |
1.000000 |
1.0000000 |
1.000000 |
2 |
rowLogSumExps |
1.027853 |
1.040682 |
1.147038 |
1.035939 |
0.9921952 |
8.373803 |
Figure: Benchmarking of colLogSumExps() and rowLogSumExps() on 100x1000 data (original and transposed). Outliers are displayed as crosses. Times are in milliseconds.
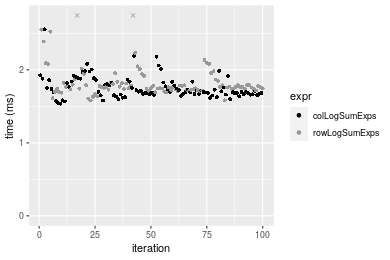
1000x100 matrix
> X <- data[["1000x100"]]
> gc()
used (Mb) gc trigger (Mb) max used (Mb)
Ncells 5228130 279.3 7916910 422.9 7916910 422.9
Vcells 9764643 74.5 33191153 253.3 53339345 407.0
> colStats <- microbenchmark(colLogSumExps = colLogSumExps(X, na.rm = FALSE), `apply+logSumExp` = apply(X,
+ MARGIN = 2L, FUN = logSumExp, na.rm = FALSE), `apply+logSumExp0` = apply(X, MARGIN = 2L, FUN = logSumExp0,
+ na.rm = FALSE), unit = "ms")
> X <- t(X)
> gc()
used (Mb) gc trigger (Mb) max used (Mb)
Ncells 5228118 279.3 7916910 422.9 7916910 422.9
Vcells 9864676 75.3 33191153 253.3 53339345 407.0
> rowStats <- microbenchmark(rowLogSumExps = rowLogSumExps(X, na.rm = FALSE), `apply+logSumExp` = apply(X,
+ MARGIN = 1L, FUN = logSumExp, na.rm = FALSE), `apply+logSumExp0` = apply(X, MARGIN = 1L, FUN = logSumExp0,
+ na.rm = FALSE), unit = "ms")
Table: Benchmarking of colLogSumExps(), apply+logSumExp() and apply+logSumExp0() on 1000x100 data. The top panel shows times in milliseconds and the bottom panel shows relative times.
|
expr |
min |
lq |
mean |
median |
uq |
max |
1 |
colLogSumExps |
1.461910 |
1.665638 |
1.874952 |
1.758566 |
1.838413 |
9.37362 |
2 |
apply+logSumExp |
2.183029 |
2.406405 |
2.725101 |
2.592052 |
2.748403 |
10.75560 |
3 |
apply+logSumExp0 |
2.546085 |
2.931929 |
3.311470 |
3.118014 |
3.495567 |
10.58627 |
|
expr |
min |
lq |
mean |
median |
uq |
max |
1 |
colLogSumExps |
1.000000 |
1.000000 |
1.000000 |
1.000000 |
1.000000 |
1.000000 |
2 |
apply+logSumExp |
1.493272 |
1.444735 |
1.453425 |
1.473958 |
1.494986 |
1.147433 |
3 |
apply+logSumExp0 |
1.741615 |
1.760244 |
1.766163 |
1.773044 |
1.901404 |
1.129369 |
Table: Benchmarking of rowLogSumExps(), apply+logSumExp() and apply+logSumExp0() on 1000x100 data (transposed). The top panel shows times in milliseconds and the bottom panel shows relative times.
|
expr |
min |
lq |
mean |
median |
uq |
max |
1 |
rowLogSumExps |
1.475570 |
1.718748 |
1.871399 |
1.757151 |
1.810914 |
9.543469 |
2 |
apply+logSumExp |
2.189496 |
2.400485 |
2.563202 |
2.460184 |
2.625558 |
5.026601 |
3 |
apply+logSumExp0 |
2.670531 |
2.930343 |
3.328314 |
2.997537 |
3.231213 |
10.639849 |
|
expr |
min |
lq |
mean |
median |
uq |
max |
1 |
rowLogSumExps |
1.000000 |
1.000000 |
1.000000 |
1.000000 |
1.000000 |
1.0000000 |
2 |
apply+logSumExp |
1.483831 |
1.396648 |
1.369671 |
1.400098 |
1.449852 |
0.5267059 |
3 |
apply+logSumExp0 |
1.809830 |
1.704929 |
1.778517 |
1.705907 |
1.784299 |
1.1148828 |
Figure: Benchmarking of colLogSumExps(), apply+logSumExp() and apply+logSumExp0() on 1000x100 data as well as rowLogSumExps(), apply+logSumExp() and apply+logSumExp0() on the same data transposed. Outliers are displayed as crosses. Times are in milliseconds.
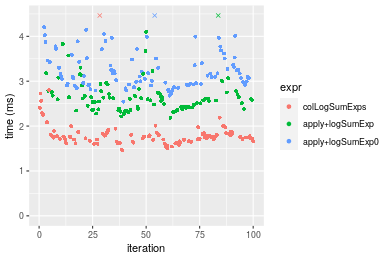
Table: Benchmarking of colLogSumExps() and rowLogSumExps() on 1000x100 data (original and transposed). The top panel shows times in milliseconds and the bottom panel shows relative times.
|
expr |
min |
lq |
mean |
median |
uq |
max |
2 |
rowLogSumExps |
1.47557 |
1.718748 |
1.871399 |
1.757151 |
1.810914 |
9.543469 |
1 |
colLogSumExps |
1.46191 |
1.665638 |
1.874952 |
1.758566 |
1.838413 |
9.373620 |
|
expr |
min |
lq |
mean |
median |
uq |
max |
2 |
rowLogSumExps |
1.0000000 |
1.0000000 |
1.000000 |
1.000000 |
1.000000 |
1.0000000 |
1 |
colLogSumExps |
0.9907426 |
0.9690996 |
1.001898 |
1.000805 |
1.015185 |
0.9822026 |
Figure: Benchmarking of colLogSumExps() and rowLogSumExps() on 1000x100 data (original and transposed). Outliers are displayed as crosses. Times are in milliseconds.
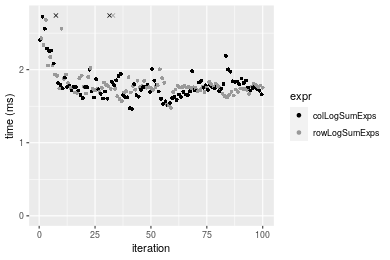
Appendix
R version 4.1.1 Patched (2021-08-10 r80727)
Platform: x86_64-pc-linux-gnu (64-bit)
Running under: Ubuntu 18.04.5 LTS
Matrix products: default
BLAS: /home/hb/software/R-devel/R-4-1-branch/lib/R/lib/libRblas.so
LAPACK: /home/hb/software/R-devel/R-4-1-branch/lib/R/lib/libRlapack.so
locale:
[1] LC_CTYPE=en_US.UTF-8 LC_NUMERIC=C
[3] LC_TIME=en_US.UTF-8 LC_COLLATE=en_US.UTF-8
[5] LC_MONETARY=en_US.UTF-8 LC_MESSAGES=en_US.UTF-8
[7] LC_PAPER=en_US.UTF-8 LC_NAME=C
[9] LC_ADDRESS=C LC_TELEPHONE=C
[11] LC_MEASUREMENT=en_US.UTF-8 LC_IDENTIFICATION=C
attached base packages:
[1] stats graphics grDevices utils datasets methods base
other attached packages:
[1] microbenchmark_1.4-7 matrixStats_0.60.0 ggplot2_3.3.5
[4] knitr_1.33 R.devices_2.17.0 R.utils_2.10.1
[7] R.oo_1.24.0 R.methodsS3_1.8.1-9001 history_0.0.1-9000
loaded via a namespace (and not attached):
[1] Biobase_2.52.0 httr_1.4.2 splines_4.1.1
[4] bit64_4.0.5 network_1.17.1 assertthat_0.2.1
[7] highr_0.9 stats4_4.1.1 blob_1.2.2
[10] GenomeInfoDbData_1.2.6 robustbase_0.93-8 pillar_1.6.2
[13] RSQLite_2.2.8 lattice_0.20-44 glue_1.4.2
[16] digest_0.6.27 XVector_0.32.0 colorspace_2.0-2
[19] Matrix_1.3-4 XML_3.99-0.7 pkgconfig_2.0.3
[22] zlibbioc_1.38.0 genefilter_1.74.0 purrr_0.3.4
[25] ergm_4.1.2 xtable_1.8-4 scales_1.1.1
[28] tibble_3.1.4 annotate_1.70.0 KEGGREST_1.32.0
[31] farver_2.1.0 generics_0.1.0 IRanges_2.26.0
[34] ellipsis_0.3.2 cachem_1.0.6 withr_2.4.2
[37] BiocGenerics_0.38.0 mime_0.11 survival_3.2-13
[40] magrittr_2.0.1 crayon_1.4.1 statnet.common_4.5.0
[43] memoise_2.0.0 laeken_0.5.1 fansi_0.5.0
[46] R.cache_0.15.0 MASS_7.3-54 R.rsp_0.44.0
[49] progressr_0.8.0 tools_4.1.1 lifecycle_1.0.0
[52] S4Vectors_0.30.0 trust_0.1-8 munsell_0.5.0
[55] tabby_0.0.1-9001 AnnotationDbi_1.54.1 Biostrings_2.60.2
[58] compiler_4.1.1 GenomeInfoDb_1.28.1 rlang_0.4.11
[61] grid_4.1.1 RCurl_1.98-1.4 cwhmisc_6.6
[64] rstudioapi_0.13 rappdirs_0.3.3 startup_0.15.0
[67] labeling_0.4.2 bitops_1.0-7 base64enc_0.1-3
[70] boot_1.3-28 gtable_0.3.0 DBI_1.1.1
[73] markdown_1.1 R6_2.5.1 lpSolveAPI_5.5.2.0-17.7
[76] rle_0.9.2 dplyr_1.0.7 fastmap_1.1.0
[79] bit_4.0.4 utf8_1.2.2 parallel_4.1.1
[82] Rcpp_1.0.7 vctrs_0.3.8 png_0.1-7
[85] DEoptimR_1.0-9 tidyselect_1.1.1 xfun_0.25
[88] coda_0.19-4
Total processing time was 17.62 secs.
Reproducibility
To reproduce this report, do:
html <- matrixStats:::benchmark('colLogSumExps')
Copyright Henrik Bengtsson. Last updated on 2021-08-25 22:16:45 (+0200 UTC). Powered by RSP.