colAnys() and rowAnys() benchmarks
This report benchmark the performance of colAnys() and rowAnys() against alternative methods.
Alternative methods
- apply() + any()
- colSums() > 0 or rowSums() > 0
Data
> rmatrix <- function(nrow, ncol, mode = c("logical", "double", "integer", "index"), range = c(-100,
+ +100), na_prob = 0) {
+ mode <- match.arg(mode)
+ n <- nrow * ncol
+ if (mode == "logical") {
+ x <- sample(c(FALSE, TRUE), size = n, replace = TRUE)
+ } else if (mode == "index") {
+ x <- seq_len(n)
+ mode <- "integer"
+ } else {
+ x <- runif(n, min = range[1], max = range[2])
+ }
+ storage.mode(x) <- mode
+ if (na_prob > 0)
+ x[sample(n, size = na_prob * n)] <- NA
+ dim(x) <- c(nrow, ncol)
+ x
+ }
> rmatrices <- function(scale = 10, seed = 1, ...) {
+ set.seed(seed)
+ data <- list()
+ data[[1]] <- rmatrix(nrow = scale * 1, ncol = scale * 1, ...)
+ data[[2]] <- rmatrix(nrow = scale * 10, ncol = scale * 10, ...)
+ data[[3]] <- rmatrix(nrow = scale * 100, ncol = scale * 1, ...)
+ data[[4]] <- t(data[[3]])
+ data[[5]] <- rmatrix(nrow = scale * 10, ncol = scale * 100, ...)
+ data[[6]] <- t(data[[5]])
+ names(data) <- sapply(data, FUN = function(x) paste(dim(x), collapse = "x"))
+ data
+ }
> data <- rmatrices(mode = "logical")
Results
10x10 matrix
> X <- data[["10x10"]]
> gc()
used (Mb) gc trigger (Mb) max used (Mb)
Ncells 5192340 277.4 7916910 422.9 7916910 422.9
Vcells 9548887 72.9 33191153 253.3 53339345 407.0
> colStats <- microbenchmark(colAnys = colAnys(X), `apply+any` = apply(X, MARGIN = 2L, FUN = any),
+ `colSums > 0` = (colSums(X) > 0L), unit = "ms")
> X <- t(X)
> gc()
used (Mb) gc trigger (Mb) max used (Mb)
Ncells 5178649 276.6 7916910 422.9 7916910 422.9
Vcells 9503582 72.6 33191153 253.3 53339345 407.0
> rowStats <- microbenchmark(rowAnys = rowAnys(X), `apply+any` = apply(X, MARGIN = 1L, FUN = any),
+ `rowSums > 0` = (rowSums(X) > 0L), unit = "ms")
Table: Benchmarking of colAnys(), apply+any() and colSums > 0() on 10x10 data. The top panel shows times in milliseconds and the bottom panel shows relative times.
|
expr |
min |
lq |
mean |
median |
uq |
max |
1 |
colAnys |
0.003015 |
0.0033380 |
0.0043223 |
0.0042475 |
0.0045720 |
0.019112 |
3 |
colSums > 0 |
0.005908 |
0.0063745 |
0.0080616 |
0.0071145 |
0.0079250 |
0.054657 |
2 |
apply+any |
0.033156 |
0.0347580 |
0.0377213 |
0.0355990 |
0.0368595 |
0.084983 |
|
expr |
min |
lq |
mean |
median |
uq |
max |
1 |
colAnys |
1.000000 |
1.000000 |
1.000000 |
1.000000 |
1.000000 |
1.000000 |
3 |
colSums > 0 |
1.959536 |
1.909676 |
1.865101 |
1.674985 |
1.733377 |
2.859826 |
2 |
apply+any |
10.997015 |
10.412822 |
8.727058 |
8.381165 |
8.062008 |
4.446578 |
Table: Benchmarking of rowAnys(), apply+any() and rowSums > 0() on 10x10 data (transposed). The top panel shows times in milliseconds and the bottom panel shows relative times.
|
expr |
min |
lq |
mean |
median |
uq |
max |
1 |
rowAnys |
0.003149 |
0.0035075 |
0.0044978 |
0.0044310 |
0.0047810 |
0.019036 |
3 |
rowSums > 0 |
0.006862 |
0.0072455 |
0.0086486 |
0.0078765 |
0.0084835 |
0.050237 |
2 |
apply+any |
0.034071 |
0.0349290 |
0.0370099 |
0.0355310 |
0.0361085 |
0.083324 |
|
expr |
min |
lq |
mean |
median |
uq |
max |
1 |
rowAnys |
1.000000 |
1.000000 |
1.000000 |
1.000000 |
1.000000 |
1.000000 |
3 |
rowSums > 0 |
2.179105 |
2.065716 |
1.922851 |
1.777590 |
1.774420 |
2.639052 |
2 |
apply+any |
10.819625 |
9.958375 |
8.228470 |
8.018732 |
7.552499 |
4.377180 |
Figure: Benchmarking of colAnys(), apply+any() and colSums > 0() on 10x10 data as well as rowAnys(), apply+any() and rowSums > 0() on the same data transposed. Outliers are displayed as crosses. Times are in milliseconds.
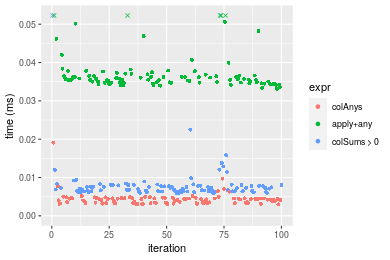
Table: Benchmarking of colAnys() and rowAnys() on 10x10 data (original and transposed). The top panel shows times in milliseconds and the bottom panel shows relative times.
|
expr |
min |
lq |
mean |
median |
uq |
max |
1 |
colAnys |
3.015 |
3.3380 |
4.32234 |
4.2475 |
4.572 |
19.112 |
2 |
rowAnys |
3.149 |
3.5075 |
4.49779 |
4.4310 |
4.781 |
19.036 |
|
expr |
min |
lq |
mean |
median |
uq |
max |
1 |
colAnys |
1.000000 |
1.000000 |
1.000000 |
1.000000 |
1.000000 |
1.0000000 |
2 |
rowAnys |
1.044444 |
1.050779 |
1.040591 |
1.043202 |
1.045713 |
0.9960234 |
Figure: Benchmarking of colAnys() and rowAnys() on 10x10 data (original and transposed). Outliers are displayed as crosses. Times are in milliseconds.
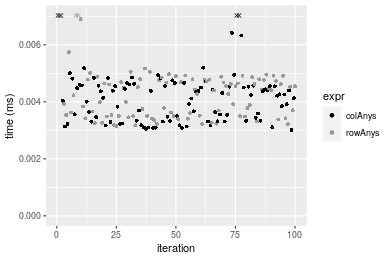
100x100 matrix
> X <- data[["100x100"]]
> gc()
used (Mb) gc trigger (Mb) max used (Mb)
Ncells 5177209 276.5 7916910 422.9 7916910 422.9
Vcells 9309779 71.1 33191153 253.3 53339345 407.0
> colStats <- microbenchmark(colAnys = colAnys(X), `apply+any` = apply(X, MARGIN = 2L, FUN = any),
+ `colSums > 0` = (colSums(X) > 0L), unit = "ms")
> X <- t(X)
> gc()
used (Mb) gc trigger (Mb) max used (Mb)
Ncells 5177203 276.5 7916910 422.9 7916910 422.9
Vcells 9314822 71.1 33191153 253.3 53339345 407.0
> rowStats <- microbenchmark(rowAnys = rowAnys(X), `apply+any` = apply(X, MARGIN = 1L, FUN = any),
+ `rowSums > 0` = (rowSums(X) > 0L), unit = "ms")
Table: Benchmarking of colAnys(), apply+any() and colSums > 0() on 100x100 data. The top panel shows times in milliseconds and the bottom panel shows relative times.
|
expr |
min |
lq |
mean |
median |
uq |
max |
1 |
colAnys |
0.003116 |
0.0039975 |
0.0053324 |
0.0048930 |
0.0055855 |
0.023137 |
3 |
colSums > 0 |
0.015894 |
0.0174145 |
0.0200416 |
0.0187445 |
0.0212160 |
0.054532 |
2 |
apply+any |
0.193797 |
0.2074970 |
0.2319392 |
0.2248080 |
0.2493780 |
0.420242 |
|
expr |
min |
lq |
mean |
median |
uq |
max |
1 |
colAnys |
1.00000 |
1.000000 |
1.000000 |
1.000000 |
1.000000 |
1.000000 |
3 |
colSums > 0 |
5.10077 |
4.356348 |
3.758443 |
3.830881 |
3.798407 |
2.356918 |
2 |
apply+any |
62.19416 |
51.906692 |
43.496134 |
45.944819 |
44.647391 |
18.163202 |
Table: Benchmarking of rowAnys(), apply+any() and rowSums > 0() on 100x100 data (transposed). The top panel shows times in milliseconds and the bottom panel shows relative times.
|
expr |
min |
lq |
mean |
median |
uq |
max |
1 |
rowAnys |
0.009437 |
0.0112045 |
0.0127247 |
0.012203 |
0.0134225 |
0.035027 |
3 |
rowSums > 0 |
0.046317 |
0.0480945 |
0.0542597 |
0.053143 |
0.0585380 |
0.124492 |
2 |
apply+any |
0.193635 |
0.2000855 |
0.2295396 |
0.218877 |
0.2494360 |
0.410195 |
|
expr |
min |
lq |
mean |
median |
uq |
max |
1 |
rowAnys |
1.000000 |
1.000000 |
1.000000 |
1.000000 |
1.000000 |
1.000000 |
3 |
rowSums > 0 |
4.908022 |
4.292427 |
4.264129 |
4.354913 |
4.361185 |
3.554172 |
2 |
apply+any |
20.518703 |
17.857602 |
18.038933 |
17.936327 |
18.583423 |
11.710823 |
Figure: Benchmarking of colAnys(), apply+any() and colSums > 0() on 100x100 data as well as rowAnys(), apply+any() and rowSums > 0() on the same data transposed. Outliers are displayed as crosses. Times are in milliseconds.
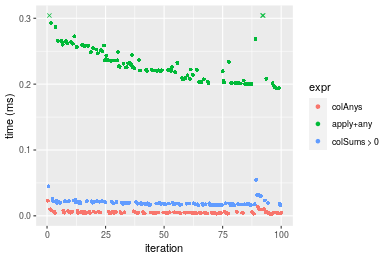
Table: Benchmarking of colAnys() and rowAnys() on 100x100 data (original and transposed). The top panel shows times in milliseconds and the bottom panel shows relative times.
|
expr |
min |
lq |
mean |
median |
uq |
max |
1 |
colAnys |
3.116 |
3.9975 |
5.33241 |
4.893 |
5.5855 |
23.137 |
2 |
rowAnys |
9.437 |
11.2045 |
12.72468 |
12.203 |
13.4225 |
35.027 |
|
expr |
min |
lq |
mean |
median |
uq |
max |
1 |
colAnys |
1.000000 |
1.000000 |
1.000000 |
1.000000 |
1.000000 |
1.000000 |
2 |
rowAnys |
3.028562 |
2.802877 |
2.386291 |
2.493971 |
2.403097 |
1.513896 |
Figure: Benchmarking of colAnys() and rowAnys() on 100x100 data (original and transposed). Outliers are displayed as crosses. Times are in milliseconds.
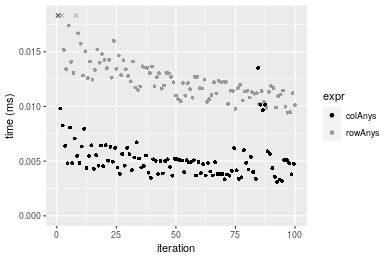
1000x10 matrix
> X <- data[["1000x10"]]
> gc()
used (Mb) gc trigger (Mb) max used (Mb)
Ncells 5177959 276.6 7916910 422.9 7916910 422.9
Vcells 9313300 71.1 33191153 253.3 53339345 407.0
> colStats <- microbenchmark(colAnys = colAnys(X), `apply+any` = apply(X, MARGIN = 2L, FUN = any),
+ `colSums > 0` = (colSums(X) > 0L), unit = "ms")
> X <- t(X)
> gc()
used (Mb) gc trigger (Mb) max used (Mb)
Ncells 5177953 276.6 7916910 422.9 7916910 422.9
Vcells 9318343 71.1 33191153 253.3 53339345 407.0
> rowStats <- microbenchmark(rowAnys = rowAnys(X), `apply+any` = apply(X, MARGIN = 1L, FUN = any),
+ `rowSums > 0` = (rowSums(X) > 0L), unit = "ms")
Table: Benchmarking of colAnys(), apply+any() and colSums > 0() on 1000x10 data. The top panel shows times in milliseconds and the bottom panel shows relative times.
|
expr |
min |
lq |
mean |
median |
uq |
max |
1 |
colAnys |
0.003017 |
0.003642 |
0.0047623 |
0.0043505 |
0.0049420 |
0.022187 |
3 |
colSums > 0 |
0.017179 |
0.019061 |
0.0204056 |
0.0196370 |
0.0209315 |
0.037863 |
2 |
apply+any |
0.111874 |
0.117432 |
0.1270182 |
0.1254865 |
0.1305995 |
0.207890 |
|
expr |
min |
lq |
mean |
median |
uq |
max |
1 |
colAnys |
1.000000 |
1.000000 |
1.000000 |
1.000000 |
1.000000 |
1.000000 |
3 |
colSums > 0 |
5.694067 |
5.233663 |
4.284792 |
4.513734 |
4.235431 |
1.706540 |
2 |
apply+any |
37.081207 |
32.243822 |
26.671505 |
28.844156 |
26.426447 |
9.369901 |
Table: Benchmarking of rowAnys(), apply+any() and rowSums > 0() on 1000x10 data (transposed). The top panel shows times in milliseconds and the bottom panel shows relative times.
|
expr |
min |
lq |
mean |
median |
uq |
max |
1 |
rowAnys |
0.008582 |
0.0100280 |
0.0113144 |
0.0109640 |
0.0119975 |
0.027385 |
2 |
apply+any |
0.096734 |
0.1010950 |
0.1140153 |
0.1101305 |
0.1276375 |
0.198634 |
3 |
rowSums > 0 |
0.167370 |
0.1747045 |
0.1910449 |
0.1875660 |
0.2097510 |
0.229876 |
|
expr |
min |
lq |
mean |
median |
uq |
max |
1 |
rowAnys |
1.00000 |
1.00000 |
1.00000 |
1.00000 |
1.00000 |
1.000000 |
2 |
apply+any |
11.27173 |
10.08127 |
10.07703 |
10.04474 |
10.63867 |
7.253387 |
3 |
rowSums > 0 |
19.50245 |
17.42167 |
16.88514 |
17.10744 |
17.48289 |
8.394230 |
Figure: Benchmarking of colAnys(), apply+any() and colSums > 0() on 1000x10 data as well as rowAnys(), apply+any() and rowSums > 0() on the same data transposed. Outliers are displayed as crosses. Times are in milliseconds.
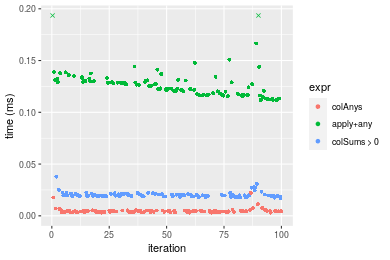
Table: Benchmarking of colAnys() and rowAnys() on 1000x10 data (original and transposed). The top panel shows times in milliseconds and the bottom panel shows relative times.
|
expr |
min |
lq |
mean |
median |
uq |
max |
1 |
colAnys |
3.017 |
3.642 |
4.76232 |
4.3505 |
4.9420 |
22.187 |
2 |
rowAnys |
8.582 |
10.028 |
11.31438 |
10.9640 |
11.9975 |
27.385 |
|
expr |
min |
lq |
mean |
median |
uq |
max |
1 |
colAnys |
1.000000 |
1.000000 |
1.000000 |
1.00000 |
1.000000 |
1.000000 |
2 |
rowAnys |
2.844548 |
2.753432 |
2.375813 |
2.52017 |
2.427661 |
1.234281 |
Figure: Benchmarking of colAnys() and rowAnys() on 1000x10 data (original and transposed). Outliers are displayed as crosses. Times are in milliseconds.
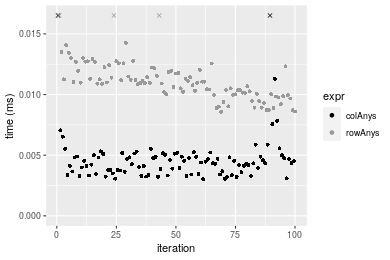
10x1000 matrix
> X <- data[["10x1000"]]
> gc()
used (Mb) gc trigger (Mb) max used (Mb)
Ncells 5178173 276.6 7916910 422.9 7916910 422.9
Vcells 9314049 71.1 33191153 253.3 53339345 407.0
> colStats <- microbenchmark(colAnys = colAnys(X), `apply+any` = apply(X, MARGIN = 2L, FUN = any),
+ `colSums > 0` = (colSums(X) > 0L), unit = "ms")
> X <- t(X)
> gc()
used (Mb) gc trigger (Mb) max used (Mb)
Ncells 5178167 276.6 7916910 422.9 7916910 422.9
Vcells 9319092 71.1 33191153 253.3 53339345 407.0
> rowStats <- microbenchmark(rowAnys = rowAnys(X), `apply+any` = apply(X, MARGIN = 1L, FUN = any),
+ `rowSums > 0` = (rowSums(X) > 0L), unit = "ms")
Table: Benchmarking of colAnys(), apply+any() and colSums > 0() on 10x1000 data. The top panel shows times in milliseconds and the bottom panel shows relative times.
|
expr |
min |
lq |
mean |
median |
uq |
max |
1 |
colAnys |
0.007453 |
0.0092850 |
0.0118857 |
0.0106215 |
0.0129310 |
0.034985 |
3 |
colSums > 0 |
0.013042 |
0.0147795 |
0.0171880 |
0.0161970 |
0.0188685 |
0.039224 |
2 |
apply+any |
0.851302 |
0.8912870 |
1.0151367 |
0.9475960 |
1.1103635 |
1.456249 |
|
expr |
min |
lq |
mean |
median |
uq |
max |
1 |
colAnys |
1.000000 |
1.000000 |
1.000000 |
1.000000 |
1.000000 |
1.000000 |
3 |
colSums > 0 |
1.749899 |
1.591761 |
1.446111 |
1.524926 |
1.459168 |
1.121166 |
2 |
apply+any |
114.222729 |
95.992138 |
85.408528 |
89.214894 |
85.868340 |
41.624954 |
Table: Benchmarking of rowAnys(), apply+any() and rowSums > 0() on 10x1000 data (transposed). The top panel shows times in milliseconds and the bottom panel shows relative times.
|
expr |
min |
lq |
mean |
median |
uq |
max |
1 |
rowAnys |
0.019350 |
0.0236435 |
0.0290122 |
0.0263760 |
0.0318340 |
0.068443 |
3 |
rowSums > 0 |
0.028014 |
0.0312395 |
0.0377033 |
0.0352885 |
0.0407545 |
0.124822 |
2 |
apply+any |
0.847844 |
0.9052355 |
1.0829320 |
1.0152130 |
1.1993540 |
1.783223 |
|
expr |
min |
lq |
mean |
median |
uq |
max |
1 |
rowAnys |
1.000000 |
1.000000 |
1.000000 |
1.000000 |
1.000000 |
1.000000 |
3 |
rowSums > 0 |
1.447752 |
1.321272 |
1.299569 |
1.337902 |
1.280219 |
1.823737 |
2 |
apply+any |
43.816227 |
38.286865 |
37.326820 |
38.490029 |
37.675253 |
26.054133 |
Figure: Benchmarking of colAnys(), apply+any() and colSums > 0() on 10x1000 data as well as rowAnys(), apply+any() and rowSums > 0() on the same data transposed. Outliers are displayed as crosses. Times are in milliseconds.
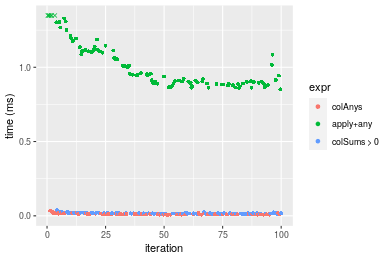
Table: Benchmarking of colAnys() and rowAnys() on 10x1000 data (original and transposed). The top panel shows times in milliseconds and the bottom panel shows relative times.
|
expr |
min |
lq |
mean |
median |
uq |
max |
1 |
colAnys |
7.453 |
9.2850 |
11.88566 |
10.6215 |
12.931 |
34.985 |
2 |
rowAnys |
19.350 |
23.6435 |
29.01217 |
26.3760 |
31.834 |
68.443 |
|
expr |
min |
lq |
mean |
median |
uq |
max |
1 |
colAnys |
1.00000 |
1.000000 |
1.000000 |
1.000000 |
1.000000 |
1.000000 |
2 |
rowAnys |
2.59627 |
2.546419 |
2.440939 |
2.483265 |
2.461836 |
1.956353 |
Figure: Benchmarking of colAnys() and rowAnys() on 10x1000 data (original and transposed). Outliers are displayed as crosses. Times are in milliseconds.
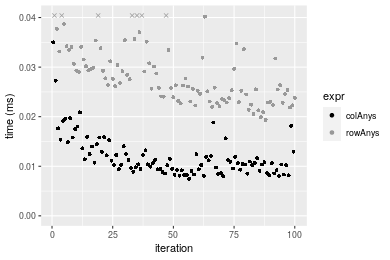
100x1000 matrix
> X <- data[["100x1000"]]
> gc()
used (Mb) gc trigger (Mb) max used (Mb)
Ncells 5178381 276.6 7916910 422.9 7916910 422.9
Vcells 9314568 71.1 33191153 253.3 53339345 407.0
> colStats <- microbenchmark(colAnys = colAnys(X), `apply+any` = apply(X, MARGIN = 2L, FUN = any),
+ `colSums > 0` = (colSums(X) > 0L), unit = "ms")
> X <- t(X)
> gc()
used (Mb) gc trigger (Mb) max used (Mb)
Ncells 5178369 276.6 7916910 422.9 7916910 422.9
Vcells 9364601 71.5 33191153 253.3 53339345 407.0
> rowStats <- microbenchmark(rowAnys = rowAnys(X), `apply+any` = apply(X, MARGIN = 1L, FUN = any),
+ `rowSums > 0` = (rowSums(X) > 0L), unit = "ms")
Table: Benchmarking of colAnys(), apply+any() and colSums > 0() on 100x1000 data. The top panel shows times in milliseconds and the bottom panel shows relative times.
|
expr |
min |
lq |
mean |
median |
uq |
max |
1 |
colAnys |
0.008363 |
0.0114285 |
0.0166425 |
0.0138285 |
0.0176545 |
0.054207 |
3 |
colSums > 0 |
0.080974 |
0.0836290 |
0.0967549 |
0.0930810 |
0.1028945 |
0.148354 |
2 |
apply+any |
1.348433 |
1.3932855 |
1.5654713 |
1.4423025 |
1.6878120 |
2.607238 |
|
expr |
min |
lq |
mean |
median |
uq |
max |
1 |
colAnys |
1.000000 |
1.000000 |
1.000000 |
1.000000 |
1.000000 |
1.000000 |
3 |
colSums > 0 |
9.682411 |
7.317583 |
5.813722 |
6.731099 |
5.828231 |
2.736805 |
2 |
apply+any |
161.237953 |
121.913243 |
94.064670 |
104.299273 |
95.602368 |
48.097810 |
Table: Benchmarking of rowAnys(), apply+any() and rowSums > 0() on 100x1000 data (transposed). The top panel shows times in milliseconds and the bottom panel shows relative times.
|
expr |
min |
lq |
mean |
median |
uq |
max |
1 |
rowAnys |
0.054470 |
0.056363 |
0.0662752 |
0.059969 |
0.0705985 |
0.131714 |
3 |
rowSums > 0 |
0.224264 |
0.226941 |
0.2577230 |
0.235087 |
0.2797685 |
0.415196 |
2 |
apply+any |
1.354361 |
1.384301 |
1.5227031 |
1.418225 |
1.5867925 |
2.406700 |
|
expr |
min |
lq |
mean |
median |
uq |
max |
1 |
rowAnys |
1.000000 |
1.000000 |
1.00000 |
1.000000 |
1.000000 |
1.000000 |
3 |
rowSums > 0 |
4.117202 |
4.026418 |
3.88868 |
3.920142 |
3.962811 |
3.152254 |
2 |
apply+any |
24.864347 |
24.560456 |
22.97546 |
23.649310 |
22.476292 |
18.272165 |
Figure: Benchmarking of colAnys(), apply+any() and colSums > 0() on 100x1000 data as well as rowAnys(), apply+any() and rowSums > 0() on the same data transposed. Outliers are displayed as crosses. Times are in milliseconds.
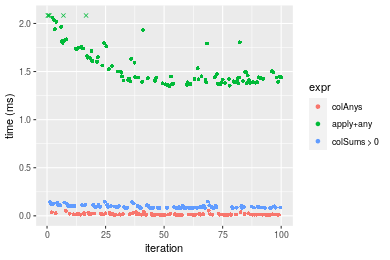
Table: Benchmarking of colAnys() and rowAnys() on 100x1000 data (original and transposed). The top panel shows times in milliseconds and the bottom panel shows relative times.
|
expr |
min |
lq |
mean |
median |
uq |
max |
1 |
colAnys |
8.363 |
11.4285 |
16.6425 |
13.8285 |
17.6545 |
54.207 |
2 |
rowAnys |
54.470 |
56.3630 |
66.2752 |
59.9690 |
70.5985 |
131.714 |
|
expr |
min |
lq |
mean |
median |
uq |
max |
1 |
colAnys |
1.000000 |
1.000000 |
1.000000 |
1.000000 |
1.000000 |
1.000000 |
2 |
rowAnys |
6.513213 |
4.931793 |
3.982286 |
4.336624 |
3.998896 |
2.429834 |
Figure: Benchmarking of colAnys() and rowAnys() on 100x1000 data (original and transposed). Outliers are displayed as crosses. Times are in milliseconds.
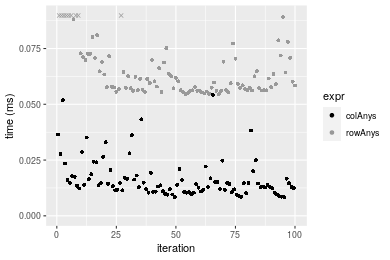
1000x100 matrix
> X <- data[["1000x100"]]
> gc()
used (Mb) gc trigger (Mb) max used (Mb)
Ncells 5178587 276.6 7916910 422.9 7916910 422.9
Vcells 9315221 71.1 33191153 253.3 53339345 407.0
> colStats <- microbenchmark(colAnys = colAnys(X), `apply+any` = apply(X, MARGIN = 2L, FUN = any),
+ `colSums > 0` = (colSums(X) > 0L), unit = "ms")
> X <- t(X)
> gc()
used (Mb) gc trigger (Mb) max used (Mb)
Ncells 5178575 276.6 7916910 422.9 7916910 422.9
Vcells 9365254 71.5 33191153 253.3 53339345 407.0
> rowStats <- microbenchmark(rowAnys = rowAnys(X), `apply+any` = apply(X, MARGIN = 1L, FUN = any),
+ `rowSums > 0` = (rowSums(X) > 0L), unit = "ms")
Table: Benchmarking of colAnys(), apply+any() and colSums > 0() on 1000x100 data. The top panel shows times in milliseconds and the bottom panel shows relative times.
|
expr |
min |
lq |
mean |
median |
uq |
max |
1 |
colAnys |
0.002769 |
0.0039285 |
0.0056187 |
0.0050880 |
0.0066740 |
0.026367 |
3 |
colSums > 0 |
0.082616 |
0.0849765 |
0.1018653 |
0.0940155 |
0.1148010 |
0.167160 |
2 |
apply+any |
0.624251 |
0.6449835 |
0.7633539 |
0.7123450 |
0.8540075 |
1.186777 |
|
expr |
min |
lq |
mean |
median |
uq |
max |
1 |
colAnys |
1.00000 |
1.00000 |
1.00000 |
1.00000 |
1.00000 |
1.000000 |
3 |
colSums > 0 |
29.83604 |
21.63078 |
18.12979 |
18.47789 |
17.20123 |
6.339743 |
2 |
apply+any |
225.44276 |
164.18060 |
135.86026 |
140.00491 |
127.96037 |
45.009937 |
Table: Benchmarking of rowAnys(), apply+any() and rowSums > 0() on 1000x100 data (transposed). The top panel shows times in milliseconds and the bottom panel shows relative times.
|
expr |
min |
lq |
mean |
median |
uq |
max |
1 |
rowAnys |
0.052694 |
0.0560710 |
0.0656955 |
0.0606060 |
0.0714685 |
0.114980 |
3 |
rowSums > 0 |
0.324020 |
0.3257205 |
0.3804144 |
0.3626460 |
0.4216195 |
0.541018 |
2 |
apply+any |
0.630176 |
0.6376395 |
0.7300979 |
0.6797115 |
0.7868810 |
1.176739 |
|
expr |
min |
lq |
mean |
median |
uq |
max |
1 |
rowAnys |
1.000000 |
1.000000 |
1.000000 |
1.000000 |
1.000000 |
1.000000 |
3 |
rowSums > 0 |
6.149087 |
5.809072 |
5.790572 |
5.983665 |
5.899375 |
4.705323 |
2 |
apply+any |
11.959160 |
11.372002 |
11.113366 |
11.215251 |
11.010179 |
10.234293 |
Figure: Benchmarking of colAnys(), apply+any() and colSums > 0() on 1000x100 data as well as rowAnys(), apply+any() and rowSums > 0() on the same data transposed. Outliers are displayed as crosses. Times are in milliseconds.
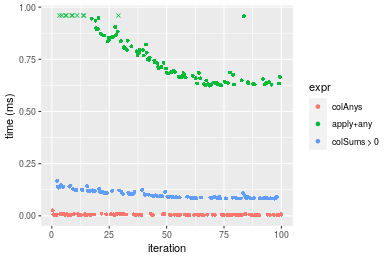
Table: Benchmarking of colAnys() and rowAnys() on 1000x100 data (original and transposed). The top panel shows times in milliseconds and the bottom panel shows relative times.
|
expr |
min |
lq |
mean |
median |
uq |
max |
1 |
colAnys |
2.769 |
3.9285 |
5.61867 |
5.088 |
6.6740 |
26.367 |
2 |
rowAnys |
52.694 |
56.0710 |
65.69548 |
60.606 |
71.4685 |
114.980 |
|
expr |
min |
lq |
mean |
median |
uq |
max |
1 |
colAnys |
1.00000 |
1.00000 |
1.00000 |
1.00000 |
1.0000 |
1.000000 |
2 |
rowAnys |
19.02997 |
14.27288 |
11.69235 |
11.91156 |
10.7085 |
4.360754 |
Figure: Benchmarking of colAnys() and rowAnys() on 1000x100 data (original and transposed). Outliers are displayed as crosses. Times are in milliseconds.
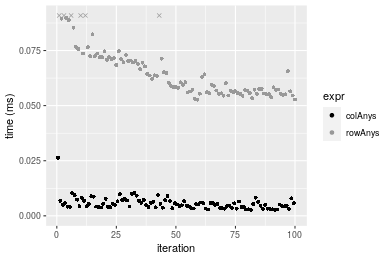
Appendix
R version 4.1.1 Patched (2021-08-10 r80727)
Platform: x86_64-pc-linux-gnu (64-bit)
Running under: Ubuntu 18.04.5 LTS
Matrix products: default
BLAS: /home/hb/software/R-devel/R-4-1-branch/lib/R/lib/libRblas.so
LAPACK: /home/hb/software/R-devel/R-4-1-branch/lib/R/lib/libRlapack.so
locale:
[1] LC_CTYPE=en_US.UTF-8 LC_NUMERIC=C
[3] LC_TIME=en_US.UTF-8 LC_COLLATE=en_US.UTF-8
[5] LC_MONETARY=en_US.UTF-8 LC_MESSAGES=en_US.UTF-8
[7] LC_PAPER=en_US.UTF-8 LC_NAME=C
[9] LC_ADDRESS=C LC_TELEPHONE=C
[11] LC_MEASUREMENT=en_US.UTF-8 LC_IDENTIFICATION=C
attached base packages:
[1] stats graphics grDevices utils datasets methods base
other attached packages:
[1] microbenchmark_1.4-7 matrixStats_0.60.0 ggplot2_3.3.5
[4] knitr_1.33 R.devices_2.17.0 R.utils_2.10.1
[7] R.oo_1.24.0 R.methodsS3_1.8.1-9001 history_0.0.1-9000
loaded via a namespace (and not attached):
[1] Biobase_2.52.0 httr_1.4.2 splines_4.1.1
[4] bit64_4.0.5 network_1.17.1 assertthat_0.2.1
[7] highr_0.9 stats4_4.1.1 blob_1.2.2
[10] GenomeInfoDbData_1.2.6 robustbase_0.93-8 pillar_1.6.2
[13] RSQLite_2.2.8 lattice_0.20-44 glue_1.4.2
[16] digest_0.6.27 XVector_0.32.0 colorspace_2.0-2
[19] Matrix_1.3-4 XML_3.99-0.7 pkgconfig_2.0.3
[22] zlibbioc_1.38.0 genefilter_1.74.0 purrr_0.3.4
[25] ergm_4.1.2 xtable_1.8-4 scales_1.1.1
[28] tibble_3.1.4 annotate_1.70.0 KEGGREST_1.32.0
[31] farver_2.1.0 generics_0.1.0 IRanges_2.26.0
[34] ellipsis_0.3.2 cachem_1.0.6 withr_2.4.2
[37] BiocGenerics_0.38.0 mime_0.11 survival_3.2-13
[40] magrittr_2.0.1 crayon_1.4.1 statnet.common_4.5.0
[43] memoise_2.0.0 laeken_0.5.1 fansi_0.5.0
[46] R.cache_0.15.0 MASS_7.3-54 R.rsp_0.44.0
[49] progressr_0.8.0 tools_4.1.1 lifecycle_1.0.0
[52] S4Vectors_0.30.0 trust_0.1-8 munsell_0.5.0
[55] tabby_0.0.1-9001 AnnotationDbi_1.54.1 Biostrings_2.60.2
[58] compiler_4.1.1 GenomeInfoDb_1.28.1 rlang_0.4.11
[61] grid_4.1.1 RCurl_1.98-1.4 cwhmisc_6.6
[64] rstudioapi_0.13 rappdirs_0.3.3 startup_0.15.0
[67] labeling_0.4.2 bitops_1.0-7 base64enc_0.1-3
[70] boot_1.3-28 gtable_0.3.0 DBI_1.1.1
[73] markdown_1.1 R6_2.5.1 lpSolveAPI_5.5.2.0-17.7
[76] rle_0.9.2 dplyr_1.0.7 fastmap_1.1.0
[79] bit_4.0.4 utf8_1.2.2 parallel_4.1.1
[82] Rcpp_1.0.7 vctrs_0.3.8 png_0.1-7
[85] DEoptimR_1.0-9 tidyselect_1.1.1 xfun_0.25
[88] coda_0.19-4
Total processing time was 12.11 secs.
Reproducibility
To reproduce this report, do:
html <- matrixStats:::benchmark('colAnys')
Copyright Henrik Bengtsson. Last updated on 2021-08-25 22:11:25 (+0200 UTC). Powered by RSP.