colAlls() and rowAlls() benchmarks
This report benchmark the performance of colAlls() and rowAlls() against alternative methods.
Alternative methods
- apply() + all()
- colSums() == n or rowSums() == n
Data
> rmatrix <- function(nrow, ncol, mode = c("logical", "double", "integer", "index"), range = c(-100,
+ +100), na_prob = 0) {
+ mode <- match.arg(mode)
+ n <- nrow * ncol
+ if (mode == "logical") {
+ x <- sample(c(FALSE, TRUE), size = n, replace = TRUE)
+ } else if (mode == "index") {
+ x <- seq_len(n)
+ mode <- "integer"
+ } else {
+ x <- runif(n, min = range[1], max = range[2])
+ }
+ storage.mode(x) <- mode
+ if (na_prob > 0)
+ x[sample(n, size = na_prob * n)] <- NA
+ dim(x) <- c(nrow, ncol)
+ x
+ }
> rmatrices <- function(scale = 10, seed = 1, ...) {
+ set.seed(seed)
+ data <- list()
+ data[[1]] <- rmatrix(nrow = scale * 1, ncol = scale * 1, ...)
+ data[[2]] <- rmatrix(nrow = scale * 10, ncol = scale * 10, ...)
+ data[[3]] <- rmatrix(nrow = scale * 100, ncol = scale * 1, ...)
+ data[[4]] <- t(data[[3]])
+ data[[5]] <- rmatrix(nrow = scale * 10, ncol = scale * 100, ...)
+ data[[6]] <- t(data[[5]])
+ names(data) <- sapply(data, FUN = function(x) paste(dim(x), collapse = "x"))
+ data
+ }
> data <- rmatrices(mode = "logical")
Results
10x10 matrix
> X <- data[["10x10"]]
> gc()
used (Mb) gc trigger (Mb) max used (Mb)
Ncells 5178000 276.6 7916910 422.9 7916910 422.9
Vcells 9439896 72.1 33191153 253.3 53339345 407.0
> colStats <- microbenchmark(colAlls = colAlls(X), `apply+all` = apply(X, MARGIN = 2L, FUN = all),
+ `colSums==n` = (colSums(X) == nrow(X)), unit = "ms")
> X <- t(X)
> gc()
used (Mb) gc trigger (Mb) max used (Mb)
Ncells 5164311 275.9 7916910 422.9 7916910 422.9
Vcells 9394591 71.7 33191153 253.3 53339345 407.0
> rowStats <- microbenchmark(rowAlls = rowAlls(X), `apply+all` = apply(X, MARGIN = 1L, FUN = all),
+ `rowSums==n` = (rowSums(X) == ncol(X)), unit = "ms")
Table: Benchmarking of colAlls(), apply+all() and colSums==n() on 10x10 data. The top panel shows times in milliseconds and the bottom panel shows relative times.
|
expr |
min |
lq |
mean |
median |
uq |
max |
1 |
colAlls |
0.002918 |
0.0033165 |
0.0040829 |
0.0040155 |
0.004426 |
0.015997 |
3 |
colSums==n |
0.006390 |
0.0069745 |
0.0081633 |
0.0076470 |
0.008282 |
0.041658 |
2 |
apply+all |
0.032027 |
0.0346345 |
0.0360910 |
0.0352475 |
0.036222 |
0.086653 |
|
expr |
min |
lq |
mean |
median |
uq |
max |
1 |
colAlls |
1.000000 |
1.00000 |
1.000000 |
1.000000 |
1.000000 |
1.000000 |
3 |
colSums==n |
2.189856 |
2.10297 |
1.999383 |
1.904371 |
1.871215 |
2.604113 |
2 |
apply+all |
10.975668 |
10.44309 |
8.839505 |
8.777861 |
8.183913 |
5.416828 |
Table: Benchmarking of rowAlls(), apply+all() and rowSums==n() on 10x10 data (transposed). The top panel shows times in milliseconds and the bottom panel shows relative times.
|
expr |
min |
lq |
mean |
median |
uq |
max |
1 |
rowAlls |
0.002918 |
0.0032065 |
0.0039516 |
0.0039845 |
0.0042960 |
0.017575 |
3 |
rowSums==n |
0.006662 |
0.0073095 |
0.0084375 |
0.0079800 |
0.0085190 |
0.053558 |
2 |
apply+all |
0.030570 |
0.0324275 |
0.0341682 |
0.0330865 |
0.0341165 |
0.081091 |
|
expr |
min |
lq |
mean |
median |
uq |
max |
1 |
rowAlls |
1.000000 |
1.000000 |
1.000000 |
1.000000 |
1.000000 |
1.000000 |
3 |
rowSums==n |
2.283071 |
2.279588 |
2.135227 |
2.002761 |
1.983007 |
3.047397 |
2 |
apply+all |
10.476354 |
10.113052 |
8.646735 |
8.303802 |
7.941457 |
4.613997 |
Figure: Benchmarking of colAlls(), apply+all() and colSums==n() on 10x10 data as well as rowAlls(), apply+all() and rowSums==n() on the same data transposed. Outliers are displayed as crosses. Times are in milliseconds.
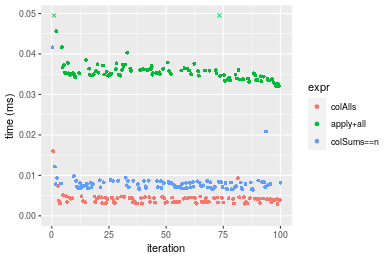
Table: Benchmarking of colAlls() and rowAlls() on 10x10 data (original and transposed). The top panel shows times in milliseconds and the bottom panel shows relative times.
|
expr |
min |
lq |
mean |
median |
uq |
max |
2 |
rowAlls |
2.918 |
3.2065 |
3.95157 |
3.9845 |
4.296 |
17.575 |
1 |
colAlls |
2.918 |
3.3165 |
4.08292 |
4.0155 |
4.426 |
15.997 |
|
expr |
min |
lq |
mean |
median |
uq |
max |
2 |
rowAlls |
1 |
1.000000 |
1.00000 |
1.00000 |
1.000000 |
1.0000000 |
1 |
colAlls |
1 |
1.034305 |
1.03324 |
1.00778 |
1.030261 |
0.9102134 |
Figure: Benchmarking of colAlls() and rowAlls() on 10x10 data (original and transposed). Outliers are displayed as crosses. Times are in milliseconds.
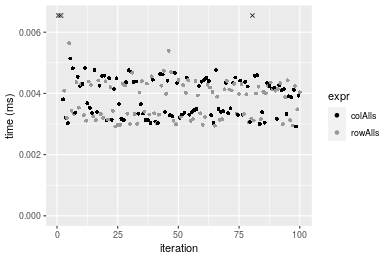
100x100 matrix
> X <- data[["100x100"]]
> gc()
used (Mb) gc trigger (Mb) max used (Mb)
Ncells 5162874 275.8 7916910 422.9 7916910 422.9
Vcells 9200790 70.2 33191153 253.3 53339345 407.0
> colStats <- microbenchmark(colAlls = colAlls(X), `apply+all` = apply(X, MARGIN = 2L, FUN = all),
+ `colSums==n` = (colSums(X) == nrow(X)), unit = "ms")
> X <- t(X)
> gc()
used (Mb) gc trigger (Mb) max used (Mb)
Ncells 5162868 275.8 7916910 422.9 7916910 422.9
Vcells 9205833 70.3 33191153 253.3 53339345 407.0
> rowStats <- microbenchmark(rowAlls = rowAlls(X), `apply+all` = apply(X, MARGIN = 1L, FUN = all),
+ `rowSums==n` = (rowSums(X) == ncol(X)), unit = "ms")
Table: Benchmarking of colAlls(), apply+all() and colSums==n() on 100x100 data. The top panel shows times in milliseconds and the bottom panel shows relative times.
|
expr |
min |
lq |
mean |
median |
uq |
max |
1 |
colAlls |
0.002933 |
0.0038555 |
0.0049758 |
0.0047205 |
0.005187 |
0.025572 |
3 |
colSums==n |
0.015744 |
0.0178695 |
0.0200639 |
0.0191665 |
0.021673 |
0.048562 |
2 |
apply+all |
0.194001 |
0.2085905 |
0.2306413 |
0.2294020 |
0.250898 |
0.341773 |
|
expr |
min |
lq |
mean |
median |
uq |
max |
1 |
colAlls |
1.000000 |
1.000000 |
1.000000 |
1.000000 |
1.00000 |
1.00000 |
3 |
colSums==n |
5.367883 |
4.634807 |
4.032323 |
4.060269 |
4.17833 |
1.89903 |
2 |
apply+all |
66.144221 |
54.102062 |
46.352981 |
48.596971 |
48.37054 |
13.36513 |
Table: Benchmarking of rowAlls(), apply+all() and rowSums==n() on 100x100 data (transposed). The top panel shows times in milliseconds and the bottom panel shows relative times.
|
expr |
min |
lq |
mean |
median |
uq |
max |
1 |
rowAlls |
0.007883 |
0.0091750 |
0.0103561 |
0.0098445 |
0.0107805 |
0.025822 |
3 |
rowSums==n |
0.045572 |
0.0481485 |
0.0536306 |
0.0524420 |
0.0584220 |
0.085888 |
2 |
apply+all |
0.185799 |
0.1944955 |
0.2200996 |
0.2165005 |
0.2421380 |
0.335716 |
|
expr |
min |
lq |
mean |
median |
uq |
max |
1 |
rowAlls |
1.000000 |
1.000000 |
1.000000 |
1.000000 |
1.000000 |
1.000000 |
3 |
rowSums==n |
5.781048 |
5.247793 |
5.178666 |
5.327035 |
5.419229 |
3.326156 |
2 |
apply+all |
23.569580 |
21.198420 |
21.253200 |
21.992026 |
22.460739 |
13.001162 |
Figure: Benchmarking of colAlls(), apply+all() and colSums==n() on 100x100 data as well as rowAlls(), apply+all() and rowSums==n() on the same data transposed. Outliers are displayed as crosses. Times are in milliseconds.
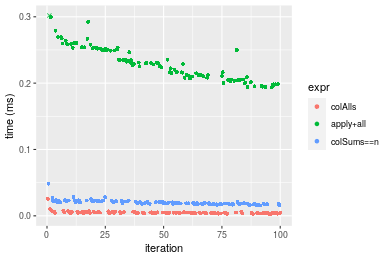
Table: Benchmarking of colAlls() and rowAlls() on 100x100 data (original and transposed). The top panel shows times in milliseconds and the bottom panel shows relative times.
|
expr |
min |
lq |
mean |
median |
uq |
max |
1 |
colAlls |
2.933 |
3.8555 |
4.97576 |
4.7205 |
5.1870 |
25.572 |
2 |
rowAlls |
7.883 |
9.1750 |
10.35607 |
9.8445 |
10.7805 |
25.822 |
|
expr |
min |
lq |
mean |
median |
uq |
max |
1 |
colAlls |
1.000000 |
1.000000 |
1.000000 |
1.000000 |
1.000000 |
1.000000 |
2 |
rowAlls |
2.687692 |
2.379717 |
2.081304 |
2.085478 |
2.078369 |
1.009776 |
Figure: Benchmarking of colAlls() and rowAlls() on 100x100 data (original and transposed). Outliers are displayed as crosses. Times are in milliseconds.
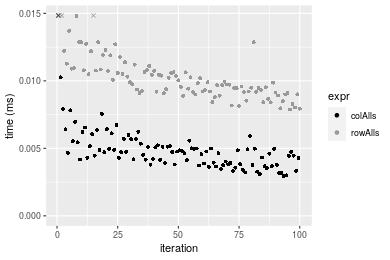
1000x10 matrix
> X <- data[["1000x10"]]
> gc()
used (Mb) gc trigger (Mb) max used (Mb)
Ncells 5163624 275.8 7916910 422.9 7916910 422.9
Vcells 9204309 70.3 33191153 253.3 53339345 407.0
> colStats <- microbenchmark(colAlls = colAlls(X), `apply+all` = apply(X, MARGIN = 2L, FUN = all),
+ `colSums==n` = (colSums(X) == nrow(X)), unit = "ms")
> X <- t(X)
> gc()
used (Mb) gc trigger (Mb) max used (Mb)
Ncells 5163618 275.8 7916910 422.9 7916910 422.9
Vcells 9209352 70.3 33191153 253.3 53339345 407.0
> rowStats <- microbenchmark(rowAlls = rowAlls(X), `apply+all` = apply(X, MARGIN = 1L, FUN = all),
+ `rowSums==n` = (rowSums(X) == ncol(X)), unit = "ms")
Table: Benchmarking of colAlls(), apply+all() and colSums==n() on 1000x10 data. The top panel shows times in milliseconds and the bottom panel shows relative times.
|
expr |
min |
lq |
mean |
median |
uq |
max |
1 |
colAlls |
0.002853 |
0.0035520 |
0.0043257 |
0.0041145 |
0.0047010 |
0.017084 |
3 |
colSums==n |
0.017351 |
0.0194050 |
0.0207849 |
0.0201080 |
0.0213510 |
0.038464 |
2 |
apply+all |
0.106709 |
0.1122245 |
0.1243842 |
0.1259220 |
0.1296695 |
0.202048 |
|
expr |
min |
lq |
mean |
median |
uq |
max |
1 |
colAlls |
1.000000 |
1.000000 |
1.000000 |
1.000000 |
1.00000 |
1.000000 |
3 |
colSums==n |
6.081668 |
5.463119 |
4.805017 |
4.887107 |
4.54180 |
2.251463 |
2 |
apply+all |
37.402383 |
31.594735 |
28.754896 |
30.604448 |
27.58339 |
11.826738 |
Table: Benchmarking of rowAlls(), apply+all() and rowSums==n() on 1000x10 data (transposed). The top panel shows times in milliseconds and the bottom panel shows relative times.
|
expr |
min |
lq |
mean |
median |
uq |
max |
1 |
rowAlls |
0.008121 |
0.0095930 |
0.0124842 |
0.0107530 |
0.0134965 |
0.025416 |
2 |
apply+all |
0.096428 |
0.1016105 |
0.1246757 |
0.1089125 |
0.1305395 |
0.250102 |
3 |
rowSums==n |
0.167941 |
0.1753380 |
0.1949295 |
0.1870195 |
0.2103385 |
0.262439 |
|
expr |
min |
lq |
mean |
median |
uq |
max |
1 |
rowAlls |
1.00000 |
1.00000 |
1.000000 |
1.00000 |
1.00000 |
1.000000 |
2 |
apply+all |
11.87391 |
10.59215 |
9.986648 |
10.12857 |
9.67210 |
9.840337 |
3 |
rowSums==n |
20.67984 |
18.27770 |
15.614044 |
17.39231 |
15.58467 |
10.325740 |
Figure: Benchmarking of colAlls(), apply+all() and colSums==n() on 1000x10 data as well as rowAlls(), apply+all() and rowSums==n() on the same data transposed. Outliers are displayed as crosses. Times are in milliseconds.
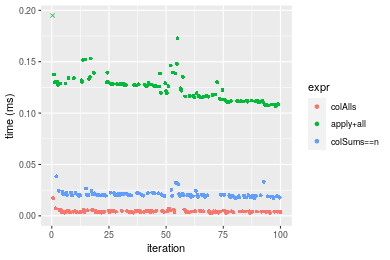
Table: Benchmarking of colAlls() and rowAlls() on 1000x10 data (original and transposed). The top panel shows times in milliseconds and the bottom panel shows relative times.
|
expr |
min |
lq |
mean |
median |
uq |
max |
1 |
colAlls |
2.853 |
3.552 |
4.32567 |
4.1145 |
4.7010 |
17.084 |
2 |
rowAlls |
8.121 |
9.593 |
12.48424 |
10.7530 |
13.4965 |
25.416 |
|
expr |
min |
lq |
mean |
median |
uq |
max |
1 |
colAlls |
1.000000 |
1.000000 |
1.000000 |
1.00000 |
1.000000 |
1.000000 |
2 |
rowAlls |
2.846477 |
2.700732 |
2.886082 |
2.61344 |
2.870985 |
1.487708 |
Figure: Benchmarking of colAlls() and rowAlls() on 1000x10 data (original and transposed). Outliers are displayed as crosses. Times are in milliseconds.
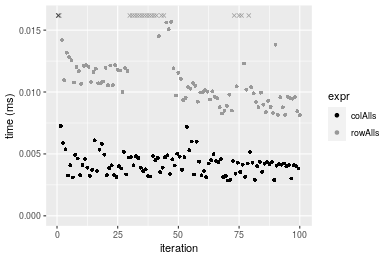
10x1000 matrix
> X <- data[["10x1000"]]
> gc()
used (Mb) gc trigger (Mb) max used (Mb)
Ncells 5163838 275.8 7916910 422.9 7916910 422.9
Vcells 9205044 70.3 33191153 253.3 53339345 407.0
> colStats <- microbenchmark(colAlls = colAlls(X), `apply+all` = apply(X, MARGIN = 2L, FUN = all),
+ `colSums==n` = (colSums(X) == nrow(X)), unit = "ms")
> X <- t(X)
> gc()
used (Mb) gc trigger (Mb) max used (Mb)
Ncells 5163832 275.8 7916910 422.9 7916910 422.9
Vcells 9210087 70.3 33191153 253.3 53339345 407.0
> rowStats <- microbenchmark(rowAlls = rowAlls(X), `apply+all` = apply(X, MARGIN = 1L, FUN = all),
+ `rowSums==n` = (rowSums(X) == ncol(X)), unit = "ms")
Table: Benchmarking of colAlls(), apply+all() and colSums==n() on 10x1000 data. The top panel shows times in milliseconds and the bottom panel shows relative times.
|
expr |
min |
lq |
mean |
median |
uq |
max |
1 |
colAlls |
0.009408 |
0.0118870 |
0.0140773 |
0.0128055 |
0.0148165 |
0.035856 |
3 |
colSums==n |
0.013396 |
0.0143105 |
0.0170158 |
0.0159030 |
0.0184325 |
0.039828 |
2 |
apply+all |
0.858664 |
0.8714435 |
1.0008542 |
0.9282170 |
1.0878390 |
1.492602 |
|
expr |
min |
lq |
mean |
median |
uq |
max |
1 |
colAlls |
1.000000 |
1.000000 |
1.000000 |
1.000000 |
1.000000 |
1.000000 |
3 |
colSums==n |
1.423895 |
1.203878 |
1.208737 |
1.241888 |
1.244052 |
1.110776 |
2 |
apply+all |
91.269558 |
73.310633 |
71.096930 |
72.485807 |
73.420781 |
41.627677 |
Table: Benchmarking of rowAlls(), apply+all() and rowSums==n() on 10x1000 data (transposed). The top panel shows times in milliseconds and the bottom panel shows relative times.
|
expr |
min |
lq |
mean |
median |
uq |
max |
1 |
rowAlls |
0.014285 |
0.0186485 |
0.0226967 |
0.0210525 |
0.0241460 |
0.067273 |
3 |
rowSums==n |
0.028580 |
0.0311925 |
0.0361926 |
0.0347380 |
0.0387395 |
0.062046 |
2 |
apply+all |
0.859912 |
0.8921850 |
1.0275150 |
0.9945310 |
1.1099595 |
1.457040 |
|
expr |
min |
lq |
mean |
median |
uq |
max |
1 |
rowAlls |
1.00000 |
1.000000 |
1.00000 |
1.000000 |
1.000000 |
1.0000000 |
3 |
rowSums==n |
2.00070 |
1.672655 |
1.59462 |
1.650065 |
1.604386 |
0.9223017 |
2 |
apply+all |
60.19685 |
47.842186 |
45.27160 |
47.240518 |
45.968670 |
21.6586149 |
Figure: Benchmarking of colAlls(), apply+all() and colSums==n() on 10x1000 data as well as rowAlls(), apply+all() and rowSums==n() on the same data transposed. Outliers are displayed as crosses. Times are in milliseconds.
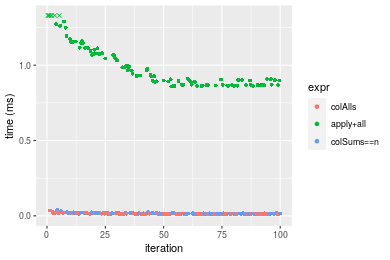
Table: Benchmarking of colAlls() and rowAlls() on 10x1000 data (original and transposed). The top panel shows times in milliseconds and the bottom panel shows relative times.
|
expr |
min |
lq |
mean |
median |
uq |
max |
1 |
colAlls |
9.408 |
11.8870 |
14.07732 |
12.8055 |
14.8165 |
35.856 |
2 |
rowAlls |
14.285 |
18.6485 |
22.69668 |
21.0525 |
24.1460 |
67.273 |
|
expr |
min |
lq |
mean |
median |
uq |
max |
1 |
colAlls |
1.000000 |
1.000000 |
1.000000 |
1.00000 |
1.00000 |
1.000000 |
2 |
rowAlls |
1.518389 |
1.568815 |
1.612287 |
1.64402 |
1.62967 |
1.876199 |
Figure: Benchmarking of colAlls() and rowAlls() on 10x1000 data (original and transposed). Outliers are displayed as crosses. Times are in milliseconds.
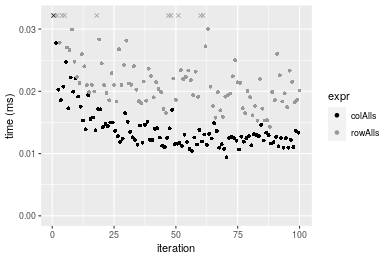
100x1000 matrix
> X <- data[["100x1000"]]
> gc()
used (Mb) gc trigger (Mb) max used (Mb)
Ncells 5164047 275.8 7916910 422.9 7916910 422.9
Vcells 9205557 70.3 33191153 253.3 53339345 407.0
> colStats <- microbenchmark(colAlls = colAlls(X), `apply+all` = apply(X, MARGIN = 2L, FUN = all),
+ `colSums==n` = (colSums(X) == nrow(X)), unit = "ms")
> X <- t(X)
> gc()
used (Mb) gc trigger (Mb) max used (Mb)
Ncells 5164035 275.8 7916910 422.9 7916910 422.9
Vcells 9255590 70.7 33191153 253.3 53339345 407.0
> rowStats <- microbenchmark(rowAlls = rowAlls(X), `apply+all` = apply(X, MARGIN = 1L, FUN = all),
+ `rowSums==n` = (rowSums(X) == ncol(X)), unit = "ms")
Table: Benchmarking of colAlls(), apply+all() and colSums==n() on 100x1000 data. The top panel shows times in milliseconds and the bottom panel shows relative times.
|
expr |
min |
lq |
mean |
median |
uq |
max |
1 |
colAlls |
0.011273 |
0.0132780 |
0.0157696 |
0.0147690 |
0.016544 |
0.040375 |
3 |
colSums==n |
0.081055 |
0.0825335 |
0.0932127 |
0.0871075 |
0.095800 |
0.193283 |
2 |
apply+all |
1.347308 |
1.3854070 |
1.5263032 |
1.3998815 |
1.611465 |
2.504890 |
|
expr |
min |
lq |
mean |
median |
uq |
max |
1 |
colAlls |
1.000000 |
1.000000 |
1.00000 |
1.000000 |
1.000000 |
1.000000 |
3 |
colSums==n |
7.190189 |
6.215808 |
5.91093 |
5.897996 |
5.790619 |
4.787195 |
2 |
apply+all |
119.516367 |
104.338530 |
96.78800 |
94.785124 |
97.404769 |
62.040619 |
Table: Benchmarking of rowAlls(), apply+all() and rowSums==n() on 100x1000 data (transposed). The top panel shows times in milliseconds and the bottom panel shows relative times.
|
expr |
min |
lq |
mean |
median |
uq |
max |
1 |
rowAlls |
0.036765 |
0.0383855 |
0.0443383 |
0.0407925 |
0.0451965 |
0.108958 |
3 |
rowSums==n |
0.224237 |
0.2258145 |
0.2543480 |
0.2275955 |
0.2768195 |
0.412877 |
2 |
apply+all |
1.350284 |
1.3681070 |
1.4806585 |
1.3936725 |
1.5038460 |
2.501126 |
|
expr |
min |
lq |
mean |
median |
uq |
max |
1 |
rowAlls |
1.000000 |
1.000000 |
1.000000 |
1.000000 |
1.000000 |
1.000000 |
3 |
rowSums==n |
6.099198 |
5.882807 |
5.736533 |
5.579347 |
6.124799 |
3.789322 |
2 |
apply+all |
36.727431 |
35.641245 |
33.394586 |
34.164920 |
33.273506 |
22.954955 |
Figure: Benchmarking of colAlls(), apply+all() and colSums==n() on 100x1000 data as well as rowAlls(), apply+all() and rowSums==n() on the same data transposed. Outliers are displayed as crosses. Times are in milliseconds.
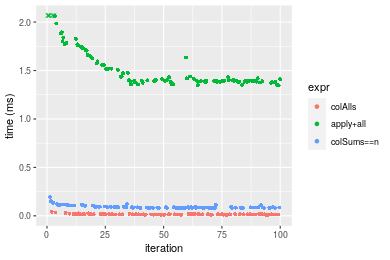
Table: Benchmarking of colAlls() and rowAlls() on 100x1000 data (original and transposed). The top panel shows times in milliseconds and the bottom panel shows relative times.
|
expr |
min |
lq |
mean |
median |
uq |
max |
1 |
colAlls |
11.273 |
13.2780 |
15.76955 |
14.7690 |
16.5440 |
40.375 |
2 |
rowAlls |
36.765 |
38.3855 |
44.33828 |
40.7925 |
45.1965 |
108.958 |
|
expr |
min |
lq |
mean |
median |
uq |
max |
1 |
colAlls |
1.000000 |
1.00000 |
1.000000 |
1.000000 |
1.000000 |
1.00000 |
2 |
rowAlls |
3.261332 |
2.89091 |
2.811639 |
2.762035 |
2.731897 |
2.69865 |
Figure: Benchmarking of colAlls() and rowAlls() on 100x1000 data (original and transposed). Outliers are displayed as crosses. Times are in milliseconds.
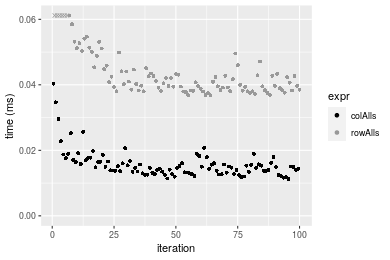
1000x100 matrix
> X <- data[["1000x100"]]
> gc()
used (Mb) gc trigger (Mb) max used (Mb)
Ncells 5164253 275.9 7916910 422.9 7916910 422.9
Vcells 9206220 70.3 33191153 253.3 53339345 407.0
> colStats <- microbenchmark(colAlls = colAlls(X), `apply+all` = apply(X, MARGIN = 2L, FUN = all),
+ `colSums==n` = (colSums(X) == nrow(X)), unit = "ms")
> X <- t(X)
> gc()
used (Mb) gc trigger (Mb) max used (Mb)
Ncells 5164241 275.9 7916910 422.9 7916910 422.9
Vcells 9256253 70.7 33191153 253.3 53339345 407.0
> rowStats <- microbenchmark(rowAlls = rowAlls(X), `apply+all` = apply(X, MARGIN = 1L, FUN = all),
+ `rowSums==n` = (rowSums(X) == ncol(X)), unit = "ms")
Table: Benchmarking of colAlls(), apply+all() and colSums==n() on 1000x100 data. The top panel shows times in milliseconds and the bottom panel shows relative times.
|
expr |
min |
lq |
mean |
median |
uq |
max |
1 |
colAlls |
0.002754 |
0.0036585 |
0.0047849 |
0.0042275 |
0.0053800 |
0.022840 |
3 |
colSums==n |
0.082708 |
0.0839100 |
0.0986052 |
0.0910275 |
0.1086585 |
0.168674 |
2 |
apply+all |
0.624761 |
0.6350055 |
0.7332335 |
0.6904375 |
0.7986980 |
1.114454 |
|
expr |
min |
lq |
mean |
median |
uq |
max |
1 |
colAlls |
1.00000 |
1.00000 |
1.00000 |
1.00000 |
1.00000 |
1.000000 |
3 |
colSums==n |
30.03195 |
22.93563 |
20.60766 |
21.53223 |
20.19675 |
7.385026 |
2 |
apply+all |
226.85585 |
173.56991 |
153.23969 |
163.32052 |
148.45688 |
48.793958 |
Table: Benchmarking of rowAlls(), apply+all() and rowSums==n() on 1000x100 data (transposed). The top panel shows times in milliseconds and the bottom panel shows relative times.
|
expr |
min |
lq |
mean |
median |
uq |
max |
1 |
rowAlls |
0.040793 |
0.0419205 |
0.0475654 |
0.0435670 |
0.0531445 |
0.082903 |
3 |
rowSums==n |
0.324184 |
0.3255680 |
0.3669326 |
0.3330525 |
0.4075610 |
0.550911 |
2 |
apply+all |
0.628847 |
0.6355465 |
0.7025238 |
0.6401720 |
0.7288735 |
1.145160 |
|
expr |
min |
lq |
mean |
median |
uq |
max |
1 |
rowAlls |
1.00000 |
1.00000 |
1.000000 |
1.000000 |
1.000000 |
1.000000 |
3 |
rowSums==n |
7.94705 |
7.76632 |
7.714273 |
7.644605 |
7.668921 |
6.645248 |
2 |
apply+all |
15.41556 |
15.16076 |
14.769633 |
14.693966 |
13.714938 |
13.813252 |
Figure: Benchmarking of colAlls(), apply+all() and colSums==n() on 1000x100 data as well as rowAlls(), apply+all() and rowSums==n() on the same data transposed. Outliers are displayed as crosses. Times are in milliseconds.
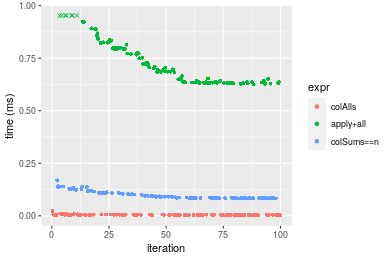
Table: Benchmarking of colAlls() and rowAlls() on 1000x100 data (original and transposed). The top panel shows times in milliseconds and the bottom panel shows relative times.
|
expr |
min |
lq |
mean |
median |
uq |
max |
1 |
colAlls |
2.754 |
3.6585 |
4.78488 |
4.2275 |
5.3800 |
22.840 |
2 |
rowAlls |
40.793 |
41.9205 |
47.56542 |
43.5670 |
53.1445 |
82.903 |
|
expr |
min |
lq |
mean |
median |
uq |
max |
1 |
colAlls |
1.00000 |
1.00000 |
1.000000 |
1.00000 |
1.00000 |
1.000000 |
2 |
rowAlls |
14.81227 |
11.45838 |
9.940776 |
10.30562 |
9.87816 |
3.629729 |
Figure: Benchmarking of colAlls() and rowAlls() on 1000x100 data (original and transposed). Outliers are displayed as crosses. Times are in milliseconds.
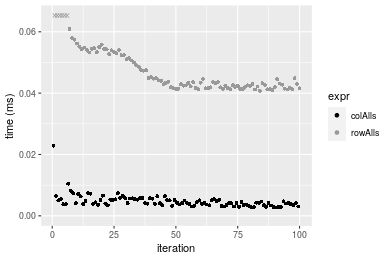
Appendix
R version 4.1.1 Patched (2021-08-10 r80727)
Platform: x86_64-pc-linux-gnu (64-bit)
Running under: Ubuntu 18.04.5 LTS
Matrix products: default
BLAS: /home/hb/software/R-devel/R-4-1-branch/lib/R/lib/libRblas.so
LAPACK: /home/hb/software/R-devel/R-4-1-branch/lib/R/lib/libRlapack.so
locale:
[1] LC_CTYPE=en_US.UTF-8 LC_NUMERIC=C
[3] LC_TIME=en_US.UTF-8 LC_COLLATE=en_US.UTF-8
[5] LC_MONETARY=en_US.UTF-8 LC_MESSAGES=en_US.UTF-8
[7] LC_PAPER=en_US.UTF-8 LC_NAME=C
[9] LC_ADDRESS=C LC_TELEPHONE=C
[11] LC_MEASUREMENT=en_US.UTF-8 LC_IDENTIFICATION=C
attached base packages:
[1] stats graphics grDevices utils datasets methods base
other attached packages:
[1] microbenchmark_1.4-7 matrixStats_0.60.0 ggplot2_3.3.5
[4] knitr_1.33 R.devices_2.17.0 R.utils_2.10.1
[7] R.oo_1.24.0 R.methodsS3_1.8.1-9001 history_0.0.1-9000
loaded via a namespace (and not attached):
[1] Biobase_2.52.0 httr_1.4.2 splines_4.1.1
[4] bit64_4.0.5 network_1.17.1 assertthat_0.2.1
[7] highr_0.9 stats4_4.1.1 blob_1.2.2
[10] GenomeInfoDbData_1.2.6 robustbase_0.93-8 pillar_1.6.2
[13] RSQLite_2.2.8 lattice_0.20-44 glue_1.4.2
[16] digest_0.6.27 XVector_0.32.0 colorspace_2.0-2
[19] Matrix_1.3-4 XML_3.99-0.7 pkgconfig_2.0.3
[22] zlibbioc_1.38.0 genefilter_1.74.0 purrr_0.3.4
[25] ergm_4.1.2 xtable_1.8-4 scales_1.1.1
[28] tibble_3.1.4 annotate_1.70.0 KEGGREST_1.32.0
[31] farver_2.1.0 generics_0.1.0 IRanges_2.26.0
[34] ellipsis_0.3.2 cachem_1.0.6 withr_2.4.2
[37] BiocGenerics_0.38.0 mime_0.11 survival_3.2-13
[40] magrittr_2.0.1 crayon_1.4.1 statnet.common_4.5.0
[43] memoise_2.0.0 laeken_0.5.1 fansi_0.5.0
[46] R.cache_0.15.0 MASS_7.3-54 R.rsp_0.44.0
[49] progressr_0.8.0 tools_4.1.1 lifecycle_1.0.0
[52] S4Vectors_0.30.0 trust_0.1-8 munsell_0.5.0
[55] tabby_0.0.1-9001 AnnotationDbi_1.54.1 Biostrings_2.60.2
[58] compiler_4.1.1 GenomeInfoDb_1.28.1 rlang_0.4.11
[61] grid_4.1.1 RCurl_1.98-1.4 cwhmisc_6.6
[64] rstudioapi_0.13 rappdirs_0.3.3 startup_0.15.0
[67] labeling_0.4.2 bitops_1.0-7 base64enc_0.1-3
[70] boot_1.3-28 gtable_0.3.0 DBI_1.1.1
[73] markdown_1.1 R6_2.5.1 lpSolveAPI_5.5.2.0-17.7
[76] rle_0.9.2 dplyr_1.0.7 fastmap_1.1.0
[79] bit_4.0.4 utf8_1.2.2 parallel_4.1.1
[82] Rcpp_1.0.7 vctrs_0.3.8 png_0.1-7
[85] DEoptimR_1.0-9 tidyselect_1.1.1 xfun_0.25
[88] coda_0.19-4
Total processing time was 11.79 secs.
Reproducibility
To reproduce this report, do:
html <- matrixStats:::benchmark('colAlls')
Copyright Henrik Bengtsson. Last updated on 2021-08-25 22:10:11 (+0200 UTC). Powered by RSP.